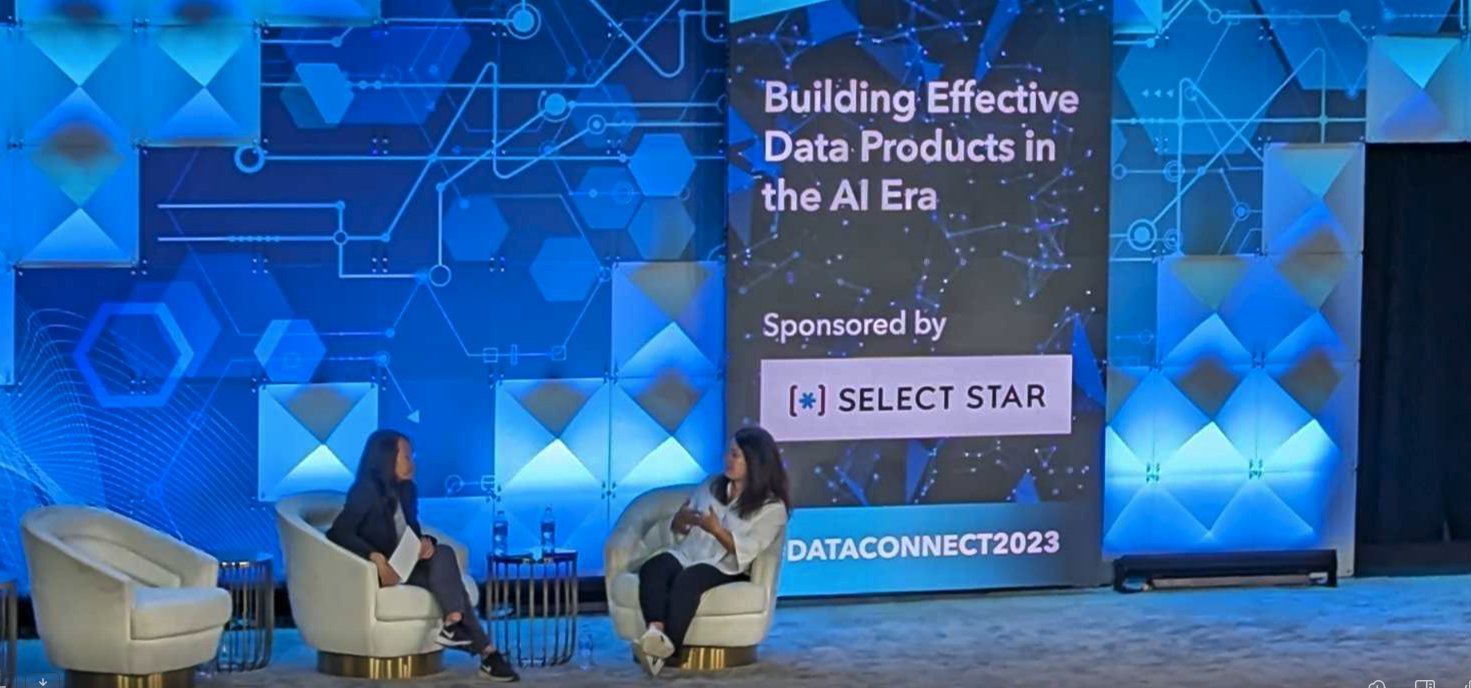
Most of the products we use every day at home and at work are expanding how they’re leveraging data. Consider Gmail as a prime example: as you draft a message, Gmail intuitively suggests sentence completions derived from patterns and frequently used phrases.
Such enhancements emphasize a broader shift in consumer expectations. With the recent advances in AI and infrastructure, consumers expect their product experiences to be smarter, more personalized, and more aware of their needs and historical context.
To make this a reality, data product development practices and tools must also evolve to meet the needs of users, business stakeholders, data scientists, engineers, and designers.
- Users want intuitive and tailored experiences that accurately reflect their prior interactions.
- Business stakeholders seek alignment with organizational goals and a strong ROI.
- Data scientists and engineers require efficient tools for building, testing, and deploying models.
- Designers aim to blend user-friendly designs with complex data functions.
Select Star founder and CEO, Shinji Kim, discussed this topic in detail with the Founding Partner of Backbone Angels and former Head of Data at Shopify, Solmaz Shahalizadeh, during this year’s DataConnect Conference. This fireside chat touched on three key aspects:
- Best practices for building data products
- Modern solutions for data discovery
- Opportunities and risks with AI and ML
Best Practices for Building Data Products
We can define a data product as a purpose-built entity that goes beyond a simple data set. A data product needs to be designed to deliver value to end users or customers by putting data into their hands for meaningful insights and decision-making.
In modern context, data products come in various forms and are accessible to a wide range of users within organizations. These forms include:
- Datasets: Data is the product. It’s a one-time dataset (e.g. marketing leads), or a contract for a refreshed set of data.
- Data as a Product: Product management and software development are applied to creating and managing internal data.
- Data Products: Entire features that are primarily data-driven (e.g. fraud detection and recommendation systems).
Data products can be visualized in interactive dashboards in tools such as Tableau or Looker, a recommendation engine behind a retail website or your social media feed, or large language models that interact with users directly. Within many companies, we see data products in innovations like real-time fraud detection and recommender systems.
These innovations have seamlessly integrated into modern user experiences, encompassing apps, websites, everyday appliances, and vehicles. For that reason, organizations must align product optimization goals with the desired user experiences to create user-friendly and efficient data products.
For instance, while developing solutions for fraud detection, users expect fast and responsive UX and the ability to quickly identify and mitigate risky transactions, which designers need to be able to reconcile with the realities of the underlying data and ML systems. From there, striking a balance between curtailing fraudulent transactions and bolstering sales is crucial. Together, this ensures the provision of a seamless and rewarding user experience while maintaining the security and integrity of the transactions.
Data Product Nuances
Part of developing data products with user experience at the forefront involves careful attention to several nuances.
Understand the user and use case needs
Creating a data product is not merely about manipulating data and applying algorithms; it’s increasingly about devising experiences that are meaningful and valuable to the users. Data practitioners must, therefore, place the user and use case at the forefront of their design process. They need to understand the user’s desires, expectations, and how they interact with the product, ensuring the outcome is not just data-driven but also user-centric.
Develop product metrics that define the user experience
Product metrics play a crucial role in gauging efficacy and shaping the user experience. They determine what aspects the underlying data and models should optimize for to align with user expectations and needs.
Taking Shopify's fraud detection as an example, the balance between false positives (type 1 error) and false negatives (type 2 error) is a testament to the criticality of choosing the right metrics. A false positive in this context would mean a legitimate transaction being flagged as fraudulent, which can deteriorate user trust and satisfaction. Conversely, a false negative – where a fraudulent transaction goes undetected – could lead to monetary losses.
Optimizing models for either reducing false positives or minimizing false negatives significantly impacts the overall user experience. Therefore, Shopify had to take a meticulous approach to ensure that the risk mitigation strategies don’t adversely affect their genuine users, while still effectively identifying and preventing fraudulent activities.
Uncertainty versus accuracy
Incorporating uncertainty and accuracy is a nuanced aspect of creating data products. Often, users expect clear and deterministic outcomes from the products they interact with. However, data, by nature, contains uncertainties and potential inaccuracies. So, we must design products that can effectively handle and communicate these uncertainties without compromising the user experience.
In instances where data models, like those used for fraud detection, lack sufficient confidence in their predictions, the product must guide users on subsequent steps they can undertake to ascertain the validity of an order. This proactive education is pivotal not only for immediate user interactions but also for fostering long-term user trust and reliance on the product.
Formalizing data in data contracts
To create effective and reliable data products, there needs to be a clear and deep understanding of all the data components involved, including the inputs, outputs, and feedback loops within the system. This understanding is then formalized through what is known as data contracts.
These contracts put in a formalized guarantee of data quality and characteristics, and make it easier to detect issues in time. They also make the maintenance of these products easier. What may seem obvious when you are building products now may not be as clear years later when it is being maintained.
Modern Solutions for Data Discovery
Machine Learning (ML) and Artificial Intelligence (AI) have expanded both the number and variety of data products, as well as the amount of data utilized, causing data teams to develop a more nuanced understanding and reasoning of their data. They must also become advocates for using data responsibly, and identifying and implementing products effectively.
Teams must counteract risks and adhere to data privacy standards to maintain user trust and prevent legal and ethical complications associated with data misuse. Complying with AI regulations related to ownership and licensing avoids legal repercussions and ensures AI is used ethically.
Data teams also need to address the opaque nature of ‘black box’ models which lack interpretability, as they can lead to user distrust and issues in accountability. Developing clear and interpretable models and deploying them responsibly is crucial to overcome these challenges.
Plus, the emergence of more third-party tools demands careful consideration. Data practitioners need to examine each tool closely to maintain control over vital components and choose those that best meet the company’s goals.
Data Discovery for Developing Effective Data Products
The volume of data collected across the world is growing rapidly. As a result, everyone has significantly more data today than 5 or 10 years ago. Working with more data and moving quickly, it’s more important to know what data you have and where it’s going. That’s why you need an automated discovery platform like Select Star that will keep up with your data needs as the organization and its data change.
For instance, when many customers come to Select Star, they have an empty data warehouse with only table and column names. However, Select Star can generate context based on their SQL queries as well as any other applications that are connected to it. This gives them richer documentation that they can then share with other citizen data scientists, business analysts, or stakeholders who want to learn more about the data.
This facilitates swift and informed data usage as well as data democratization, empowering more individuals to create data products. It also ensures that the needs of data creators align effectively with those of data consumers, enabling a seamless flow of information and insights.
Standardization and Governance
Establishing unified definitions in data management – such as data classifications and SLA hierarchies like gold, silver, and bronze tables – ensures consistency, reducing ambiguities and inefficiencies. This standardized approach also accelerates decision-making, since teams can now work from a shared understanding.
Equally important is the handling of PII. Organizations must adopt a company-wide unified classification system for personal information so that all departments treat sensitive data with consistent security and compliance measures, thereby minimizing the risk of breaches and upholding regulatory requirements.
Opportunities and Risks with AI and ML
In the context of data products, advanced machine learning and AI tools create dozens of opportunities, specifically through improving and democratizing access to data. In fact, it’s pivotal to facilitating more equitable and widespread utilization of data-driven insights, and fostering innovation across various domains.
The surge in Generative AI – particularly open-sourced models – enables the creation of domain-specific solutions and addresses real, nuanced problems. Especially in e-commerce or finance, where fraud detection is critical, Generative AI can model normal transaction behaviors, to identify anomalies and potential fraudulent activities with enhanced precision and reliability. This is critical for maintaining user trust and security in digital platforms.
Ultimately, with the elevation of data products and improved accessibility, organizations are empowered to leverage data effectively, promising a future rich with informed, data-driven decisions. Whether it’s maintaining data privacy, understanding and mitigating risks, or complying with AI regulations, building data products today involves being innovative as well as responsible and user-centric.
Now, advanced tools are commoditizing access to data and fostering an environment where organizations, irrespective of their scale, can leverage high-level technologies to solve specific domain problems. By aligning AI technology with user-centric approaches and responsible practices, organizations enhance user experiences and boost organizational success.
📹 For a deeper dive into the discussions and insights shared, watch the full recording of the fireside chat here.