Organizations increasingly recognize data as a crucial asset for driving business success. The concept of treating "data as a product" has emerged as a powerful approach to maximize the value of data assets. Many of Select Star customers embody this mindset and use Select Star to effectively deliver data as a product across their organizations today. This blog post explores the key principles, benefits, and challenges of implementing data as a product, along with practical steps for organizations to adopt this mindset.
Table of Contents
- What is Data as a Product?
- What are the benefits and challenges of treating Data as a Product?
- The rising need for Data Product Managers
- 5 steps to follow when building Data Products
- What’s next for Data as a Product?
What is Data as a Product?
Data as a product represents a shift in how organizations view and manage their data assets. This approach applies product management principles to datasets, emphasizing user-centricity, quality, accessibility, and scalability. Unlike traditional data products, which may be standalone tools or features using data, data as a product focuses on treating datasets themselves as valuable assets designed for consumption by downstream users.
Key principles of data as a product include:
- User-centricity: Focusing on the needs and goals of data consumers
- Quality and reliability: Ensuring data accuracy, completeness, and timeliness
- Accessibility and discoverability: Making data easy to find and use across your organization
- Scalability and flexibility: Adapting to changing requirements and growing data volumes
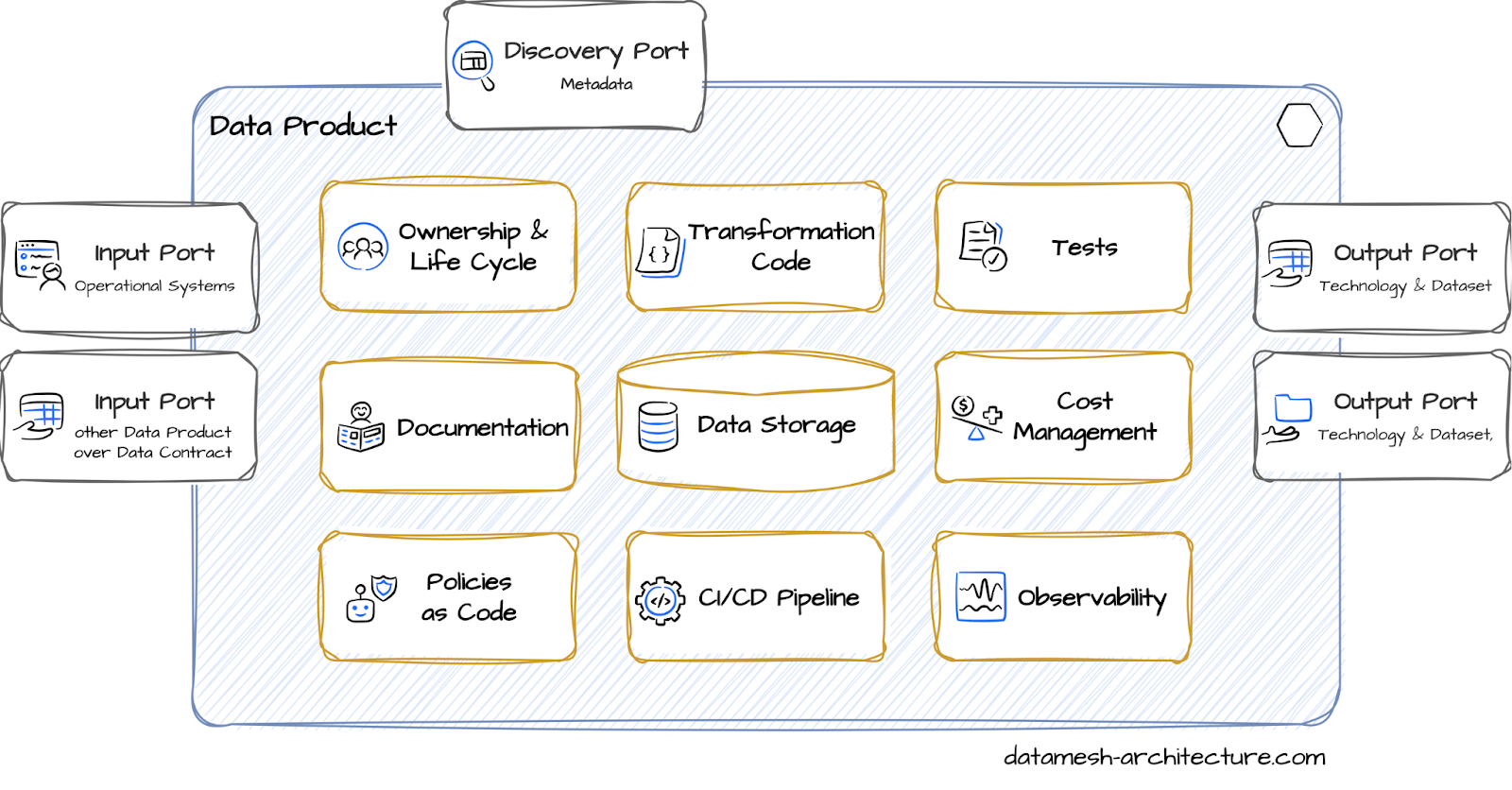
What are the benefits and challenges of treating Data as a Product?
Embracing a data-as-a-product approach offers substantial advantages for organizations. When your organization treats data as a product, you can unlock more actionable insights, leading to better decision-making at all organizational levels. This mindset fosters increased trust in data, as users recognize the rigorous management applied to these assets, much like other critical products. As a result, your users are more inclined to rely on this data for essential business processes. Furthermore, this product-centric view of data promotes cross-functional collaboration, effectively dismantling barriers between technical and business teams. Perhaps most importantly, viewing data as a product ensures that data initiatives are closely aligned with real business challenges and overarching company objectives, maximizing the value derived from these efforts.
Despite its clear benefits, transitioning to a data-as-a-product approach presents several challenges for organizations. Adopting this mindset often demands significant cultural shifts, requiring your teams to fundamentally change how they view and interact with data. Existing technical infrastructure may need substantial updates or replacements to support a more product-oriented approach to data management. Ensuring consistent data quality across diverse sources and use cases can be complex, while quantifying the value of data products to justify investments poses its own difficulties. Creating intuitive data models that meet diverse user needs requires careful planning and execution. Additionally, compliance with data privacy laws and security requirements adds another layer of complexity to the implementation process. These challenges underscore the need for a comprehensive strategy when embracing data as a product.
The rising need for Data Product Managers
As organizations embrace data as a product, the demand for specialized data product managers is increasing. Data product managers play a pivotal role in addressing the challenges inherent in implementing a data-as-a-product approach. By bridging the gap between technical and business perspectives, data product managers facilitate the cultural shifts required for successful adoption. They navigate the complexities of updating technical infrastructure, ensuring data quality across diverse sources, and creating intuitive data models that meet varied user needs. Moreover, these experts are instrumental in quantifying the business value of data products, justifying investments, and aligning data initiatives with overarching company objectives. Their ability to translate data insights into actionable strategies while adhering to privacy and security requirements makes them indispensable in driving data-driven decision-making and fostering cross-functional collaboration in increasingly complex data ecosystems.
5 steps to follow when building Data Products

To successfully implement a data-as-a-product approach, data product managers in your organization should follow these key steps:
- Define the purpose and audience: Ensure that your data initiatives align with real business problems and company objectives. This alignment is crucial for gaining stakeholder buy-in and driving meaningful impact.
- Set measurable goals: Key performance indicators (KPIs) or ways to measure impact for your data products will help track progress and demonstrate the value of data to the organization.
- Build a cross-functional team: Work collaboratively with data scientists, engineers, and business stakeholders to ensure that data products meet the needs of all users and align with organizational goals.
- Develop robust design and launch plans: Create a comprehensive approach that combines traditional product planning with data-specific considerations, including data collection, maintenance, and ongoing governance. Establish processes to ensure the accuracy and reliability of your data products throughout their lifecycle.
- Continue to test and iterate after launch: Leverage your cross-functional team for feedback, review usage analytics, and monitor evolving requirements to implement changes as needed.
The launch process for data products can be further broken down into commercial release cycle and data product maturity levels. Start with ideation, where concepts are explored and initial prototypes developed. Move to a private preview stage, allowing a select group of users to test and provide early feedback. Next, transition to a public preview, expanding access of the fairly fleshed -out data product to a wider audience while still gathering input and refining features. Finally, reach general availability, where the data product is fully launched and accessible to all intended users. This phased approach allows for controlled testing, iterative improvements, and gradual scaling of your data product.
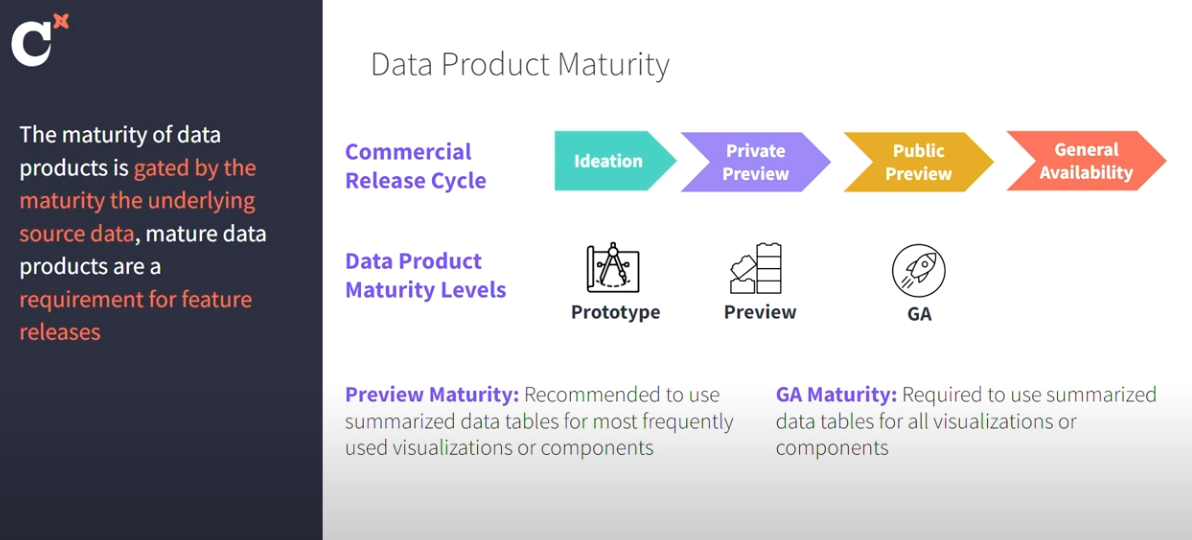
What’s next for Data as a Product?
Emerging technologies and evolving business needs will shape this approach of treating data as a product. Artificial intelligence and machine learning are playing increasingly important roles in data product development, enabling increased automation of data curation and maintenance.
The long-term impact of treating data as a product will fundamentally change your organization operates and makes decisions. By applying a product mindset to data management, you foster a data-driven culture throughout the organization. Data shifts from being a byproduct of business operations to an increasingly valuable asset with its own lifecycle. This in turn enables data-driven decision-making to scale throughout your organization. In the coming years, data will not just inform decisions. It will drive entirely new business models, positioning companies to lead in the data-driven economy.
As you think about managing data within your organization, don't hesitate to connect with our team to see how we can help your data journey.