
Are you tired of analysts wasting hours hunting for the right dataset? Sick of new hires taking months to get up to speed? Data catalogs promise to solve many data management headaches, but don't rush into implementation without a solid proof of concept (POC).
A data catalog POC enables you to understand the value a data catalog can provide to your organization and identify the tool that best positions you for success. Select Star has guided numerous customers through POCs, helping them evaluate Select Star's data catalog capabilities and make informed decisions about implementation.
This post takes you through our 5 best practices for a data catalog POC: defining success criteria, engaging stakeholders early, timeboxing the evaluation, using production data, and tracking time and insights.
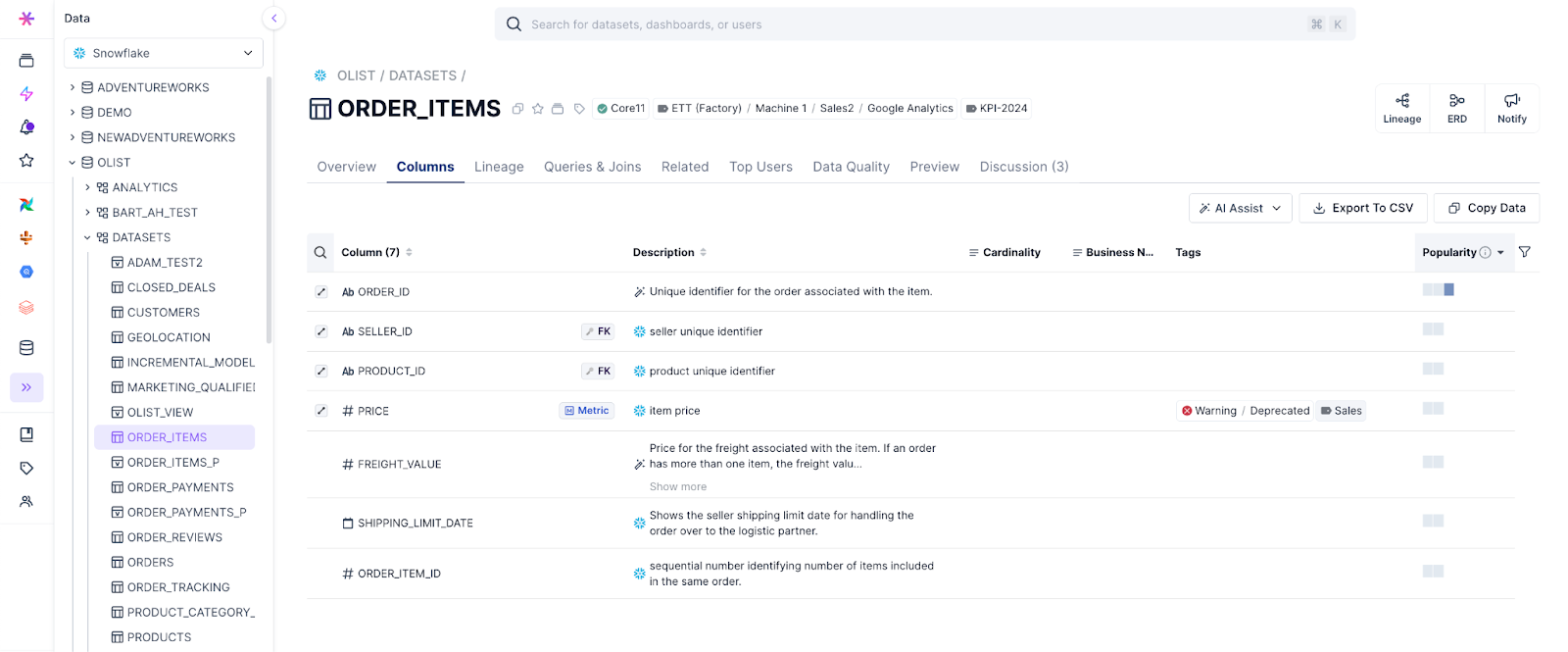
1. Define Success Criteria
Before jumping into the POC, set clear success metrics. Ask:
- What business problem are we solving? (e.g., reducing time spent finding data, improving governance compliance)
- What does success look like? (e.g., complete metadata ingestion within X hours, column-level lineage for snowflake & tableau)
- Who benefits from this integration? (data engineers, analysts, governance teams)
A clearly defined success criteria will help determine if and which data catalog delivers value and aligns with your needs.
2. Engage Key Stakeholders Early
Successful data catalog rollouts need buy-in from multiple teams. Examples:
- Data engineers (who set up the integrations) or Software engineers (data producers)
- Analysts & BI teams (who rely on metadata for discovery)
- Governance & compliance teams (who manage access & policies)
Getting their feedback early ensures the tool meets their needs and adoption isn’t an afterthought. Have them fill out a simple survey.
3. Timebox the Evaluation
Avoid open-ended POCs. Instead, set a structured testing window:
- 1-2 weeks for evaluating core metadata ingestion, lineage mapping, and user experience
- 1-3 months for validating governance workflows & user adoption
Keep it focused with specific goals to validate key assumptions, like:
- How often does metadata refresh?
- How accurate is column- & table-level lineage?
- Does the tool surface useful recommendations?
4. Use Production Data
- Use production data for key integrations (e.g., Snowflake, BigQuery, dbt)
- Check performance & scalability under real workloads
- Evaluate security & permissions handling
5. Track Time & Insights
- How long does the integration take from connection setup to full metadata population?
- Evaluate key workflows: On finding data, writing SQLs, debugging data issues - do you see a meaningful difference in speed and quality?
- Anything you didn't know before about your data that you know now?
We often hear that data catalog implementations take 9 months to 2 years—but they don’t have to. A structured, timeboxed evaluation with clear success criteria and stakeholder buy-in can accelerate adoption and help you make informed decisions faster.
Frequently Asked Questions
What is a data catalog?
A data catalog is a centralized repository that organizes and manages metadata about an organization's data assets. It helps users discover, understand, and trust data across various sources.
What is a POC?
A POC, or Proof of Concept, is a small-scale, focused test to validate a concept's viability. In the context of data catalogs, a POC helps organizations evaluate a tool's functionality, assess its fit with existing systems, and determine its potential value before committing to full implementation.