Data reigns supreme, but bridging the gap between raw information and actionable insights remains problematic for many organizations. As teams strive to harness the power of their data, the need for effective data governance becomes increasingly apparent.
In a recent webinar, we were joined by experts from Xebia and authors of the book Fundamentals of Analytics Engineering to discuss how data governance is foundational to the simple-yet-profound thesis of analytics engineering: quality pipelines yield quality data.
What is Analytics Engineering?
Author Ricardo Granados said analytics engineering bridges the gap between data consumers and data engineering teams. Born of the twin imperatives to streamline processes and enhance data quality, analytics engineering shapes organizations’ data landscapes.
Analytics engineering shepherds data from the raw state data engineers work on to the models data analysts use for business intelligence. It accelerates the development of quality data pipelines by enabling seamless communication and collaboration.
Data engineers construct datasets that analytics engineers organize into models, allowing data analysts to draw actionable insights.
Without analytics engineering, data doesn’t have the context required to drive decisions. Teams lacking a dedicated analytics engineer need data engineers with deep business understanding or data analysts with strong technical skills to fill the gap.
"If you don't have an analytics engineer in your team... someone else needs to do [the] task," Ricardo explained.
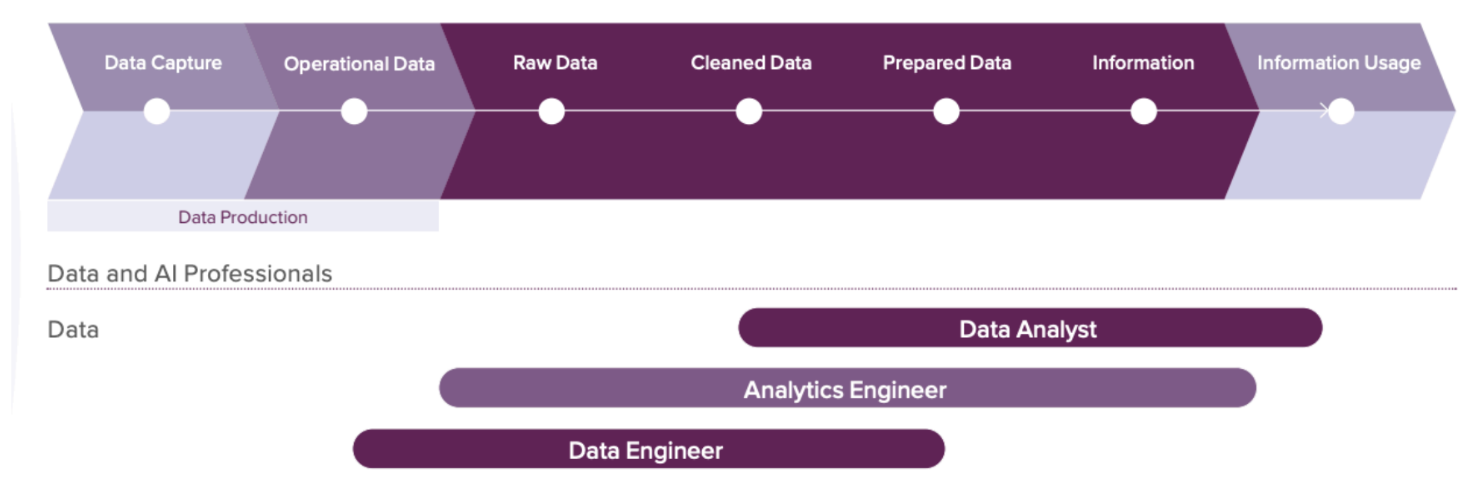
Addressing Data Quality Issues
Author Dumky De Wilde identified three key pipeline phases to identify and solve data quality problems: source, transformation, and governance.

Source
Before it can solve data quality problems, an organization must first define their root cause – identifying discrepancies, inaccuracies, and inconsistencies that may compromise the integrity of its data.
Once the problem is defined, individual issues can be categorized and available solutions can be identified.
Each category – from data model discrepancies to transformation challenges – demands a uniquely tailored approach. Such targeted strategies minimize disruptions and maximize data integrity while issues are resolved.
Transformation
Transformation is what happens to data as it travels from point A to point B. This critical stage can be fraught with challenges such as formatting discrepancies, inconsistent schema, and integration problems.
Modern tools and technologies reduce the risks of the transformation stage. Processes like data cleansing and ETL can streamline data movement and protect data quality.
Governance
Governance is the final check verifying and protecting data quality.
"Governance to me is everything around how we look at and define data," Dumky said.
Good data governance includes clear documentation, defined ownership responsibilities, and well-managed metadata.
- Documentation serves as a roadmap, clarifying data lineage, usage, and transformations.
- Defined ownership facilitates accountability, holding individuals who touch data responsible for quality at every stage of the data lifecycle.
- Metadata management provides crucial insights into data assets, enabling discoverability, lineage tracking, and compliance.
How Governance Impacts Data Quality
As important as governance is, it’s easy to go overboard with documentation, consensus, and accountability, Dumky warned.
“It has to fit your business,” he explained. “Where is your investment most warranted?
Documentation
Meticulously recording assumptions, processes, and decisions around data facilitates clarity and transparency, ensuring that everyone involved understands the underlying assumptions guiding operations.
“Document your assumptions and the choices that you make in a way that you can look back at your historical self and really know what happened at the time you made that choice,” Dumky advised. “It’s all too often that we come back to our SQL query a year from now and we’re like, ‘I don’t remember what I did here or why I did this.’”
Consensus
When data governance frameworks enable consensus-building processes, organizations can effectively navigate data complexities, driving alignment and collaboration across teams.
By democratizing access to data, organizations empower individuals to make informed decisions, elevating the overall quality of data-driven insights.
Ownership and Accountability
In a mature data team, assets can be easily transferred from one owner to another, said Juan Manuel Perafan.
Once ownership is established, SLAs ensure operations meet defined standards and benchmarks. This contractual approach enables trust and reliability, instilling confidence in the integrity of data processes.
How Select Star Helps Analytics Engineering Teams
Shinji explained several ways Select Star helps organizations with data governance.
1. Modern Data Catalog and Automated Documentation Tool
Select Star provides a comprehensive overview of data assets, facilitating seamless collaboration and knowledge sharing among team members.
2. Seamless Data Source Connectivity
One of Select Star's standout features is its ability to seamlessly connect to data sources, extracting metadata, processing history, and logs.
This connectivity ensures that organizations have real-time insights into their data ecosystem, enabling informed decision-making and proactive problem-solving.
3. Prioritization of Data Insights
Select Star goes beyond data cataloging and offers insights into data utilization patterns.
By analyzing usage data, Select Star identifies the top-performing data assets and users, allowing organizations to prioritize documentation efforts accordingly.
4. Column-Level Lineage
With Select Star, organizations gain granular visibility into data lineage and column-level usage.
This deep understanding of data relationships and dependencies empowers analytics engineering teams to trace data flows, identify bottlenecks, and optimize data pipelines for enhanced performance and reliability.
Select Star's flexible API enables seamless integration with existing data management and analytics tools, enhancing interoperability and scalability.
5. Simplified Data Migration
Select Star streamlines the data migration process, simplifying the transfer of data between systems while maintaining data integrity and lineage.
Select Star provides invaluable insights and tools to ensure a seamless transition with minimal disruption.
Bridge the Gap Between Consumers and Engineering Teams with Select Star
With Select Star, you can overcome conflicting data sources, prioritize critical data assets, and gain granular insights into your data lineage.
Watch how Select Star's modern data catalog, seamless connectivity, and advanced analytics capabilities can transform your analytics engineering workflows and propel your organization toward data excellence. Request a demo today.