Since our introductory post about data discovery, we’ve had conversations with more than 100 data leaders who were interested in Select Star. Many of them have shared that they are looking for a better way to approach the issue of data governance.
Traditionally, data governance has been defined as managing data integrity and the access of enterprise systems. Usually it consists of a centralized team with a steering committee, data stewards, process workflows and policies. In a traditional environment when you have a centralized data team, this can work well, but in today’s world where each department has their own analysts creating different analyses, such a project will likely fail.
If modern organizations want to enable data-driven decisions across departments, the traditional, top-down governance model must evolve to embrace the reality of distributed data ownership. This article is the first of a two-part series we’re going to write on data governance. In part one, we’ll explain why traditional data governance strategies are failing in modern organizations using modern data stacks. In part two, we’ll talk about how to work within the modern data world to achieve data governance.
Centralized vs. decentralized data governance
To understand why data governance is changing, we need to understand the difference between centralized and decentralized data governance models.
Traditional data governance follows a top-down model where business leaders control the master data, and data stewards manage data creation and access points. This gives a central body the authority, responsibility, and control over data access. Larger corporations choose this method to control data by putting limitations and restrictions on specific material.
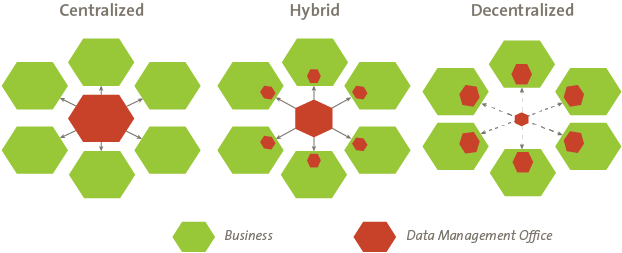
Decentralized data governance is a newer approach to data governance, arising alongside concepts like data mesh. Decentralized data governance empowers data producers and domain experts to manage and monitor data flow and access points on their own. This model creates multiple groups of authority based on where the data originated.
Traditional data governance strategies are no longer effective
With the rise of the modern data stack, all business data is now being centralized into cloud data lakes and data warehouses. This makes it easier for teams to join and analyze different datasets together, whether it’s product, marketing, sales, or finance data. But it can also create challenges for those charged with creating a data governance strategy in the organization.
There are a few trends starting to take hold as the need for access data expands beyond engineering teams.
1. Decentralized data ownership
Decentralized data ownership gives each department the power to create its own datasets and reports instead of having to rely on a centralized BI team. Some organizations accomplish this by implementing data mesh, encouraging domain owners to create data transformations and analysis independently.
Under this structure, different departments have freedom to utilize the company's core datasets (“data mart”), as well as what is gathered from the tools they use directly. Business and operations software available today, like Salesforce, Google Ads, and Zuora, provide all data and activity logs via API. This makes it easy to transfer data to and from data warehouses that contain production data.
Often, the tool becomes the source of truth for whichever group is using it, since all the analysis is in the tool. But this means two different business groups, say Sales and Finance, may end up calculating the same metric to represent “monthly active users” in different ways, and come to different conclusions.
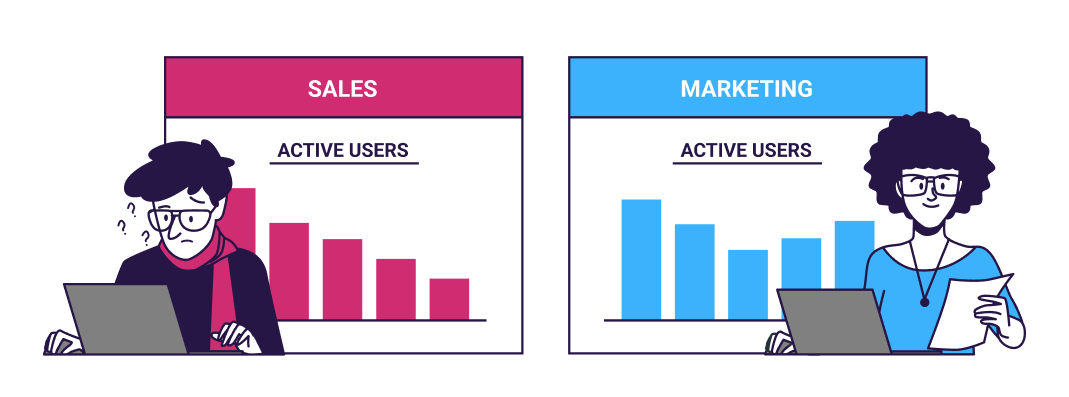
It’s very easy for different groups to develop disparate understanding of the same dataset as well. Dashboards may have the same name, but would look slightly different since the data has been transformed differently.
So how do we get everyone on the same page? With decentralized data ownership, it’s very difficult and costly to control data transformation to the point where everyone uses one universal name or definition. Instead, the goal should be to make the domain context and differences more visible, so that users can find relevant definitions that’s already been created and being used.
In order to effectively govern data as a whole, it is important to provide visibility into how the data is transformed and used by different teams that’s accessible to all data users.
2. Data democratization
Many users, including non-technical users, can now access and run data analysis directly through BI tools as a by-product of data democratization. Data democratization empowers everyone in the organization by giving them access to relevant data and helping them understand how they can use it. Different business departments will often curate the data they use, and may attempt to keep track of the semantic meaning of data created within their tools.
Traditional data governance thinking assumes the data warehouse is only accessed by the data platform team, and that analysis layers built on top of the data warehouse are controlled by an approval process. This bottleneck may still exist, and there may be an expectation that the creation of new datasets is controlled in a way that it should be possible to track data. However, connecting BI tools to data warehouses means it’s going to be easier for people to get access to data in general.
Access to data through a BI tool like Tableau is typically less restricted than direct access to a database. Many BI tools also have their own access control layer. Today’s data governance requires more integrations on the consumption layer in order to support data democratization in organizations.
3. Emerging data roles affect data control
As new roles like “analytics engineer” and “citizen data scientist” are created so marketing, operations, and other non-engineering teams can make data-driven decisions, companies face a higher potential of data silos within these departments. Engineering teams charged with data governance compliance have a harder time tracking data access because people with these new roles can create their own datasets, analysis, and reports as needed.
The speed at which these individuals create, access, and explore data can lead to an uncontrolled increase in the amount of data there is to manage. While everyone agrees data is more useful when it is documented, few people want to be responsible for creating all that documentation themselves.
It is common for companies to try to address data governance by distributing the work of documenting and maintaining datasets among teams, but such a solution is not sustainable. Data is simply being created too quickly for any number of people to manage manually.
Data discovery is a key for data governance
How, then, do we implement data governance in an environment where the volume of data is uncontrollable and ever-increasing? We believe an automated data catalog can play a big role here.
Decentralized data governance, data democratization, and new embedded data roles allow domain experts to freely explore and make progress with data quickly. These trends have been a naturally occurring response to the realization that data can drive business growth from more angles than just engineering. The aim of these trends is to enable data discovery within an organization—to make it easier for anyone to see what data is available and understand how it can be used.
If we shift our focus and understand that data discovery is necessary, we can plan for data governance accordingly. To make data discovery possible for everyone, and to allow data to grow in a controlled but organized way, we must think of data catalogs as necessary as well.
In part 2 of the article, we will explain how to use a data catalog to create data discovery and data governance in a balanced way that can scale with your organization.