Data observability tools have become essential components of modern data stacks. These tools enable data teams to monitor the health of their data systems, swiftly identify anomalies, and ensure data quality and reliability. By providing real-time insights into data flows and system performance, observability tools help organizations maintain high standards of data integrity and operational efficiency.
Selecting the right data observability tool is crucial for organizations aiming to optimize their data management practices. Numerous options are available, each with unique features and capabilities. Here's an overview of some leading data observability tools trusted by growing tech companies in 2025.
- Acceldata
- Bigeye
- dbt Test
- Elementary
- AWS Glue Data Quality
- Great Expectations
- Metaplane
- Monte Carlo Data
- Pantomath
- Synq
- Telmai
- Unravel
- Validio
Acceldata
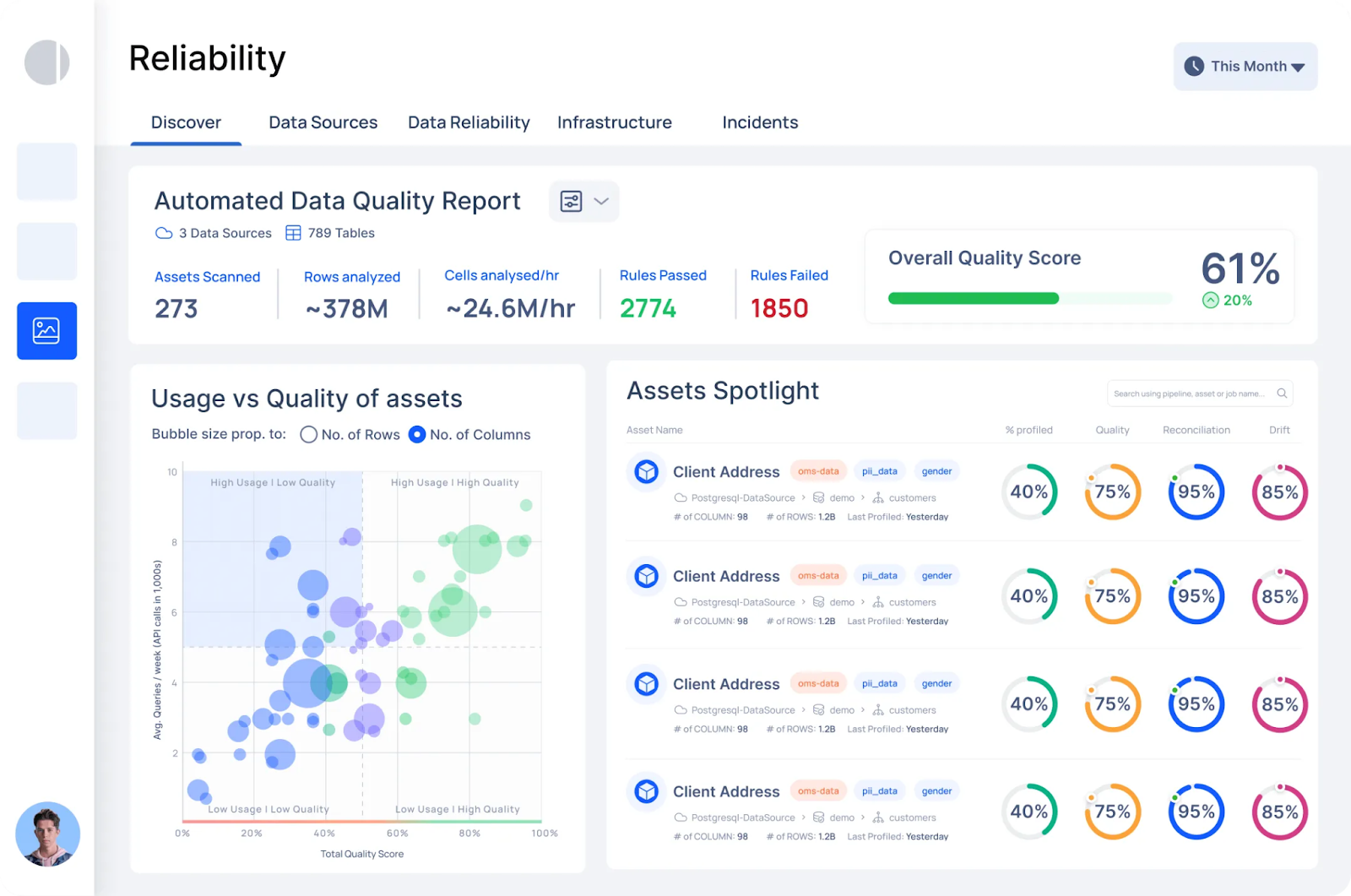
Acceldata offers a user-friendly interface coupled with powerful features like multi-layered data analysis and automated reliability checks. Its ability to provide comprehensive insights into data operations in real-time makes it an attractive option for organizations seeking to enhance their data observability practices. Acceldata's integration capabilities with multiple platforms further add to its versatility.
Bigeye

Bigeye provides automated data quality monitoring and observability solutions. The platform uses machine learning to detect anomalies and data drift, enabling teams to proactively address issues before they impact downstream processes. Bigeye's customizable thresholds and alerting system allow organizations to tailor monitoring to their specific needs. With its ability to integrate with various data sources and collaboration features, Bigeye helps foster a data-driven culture across teams.
dbt Test
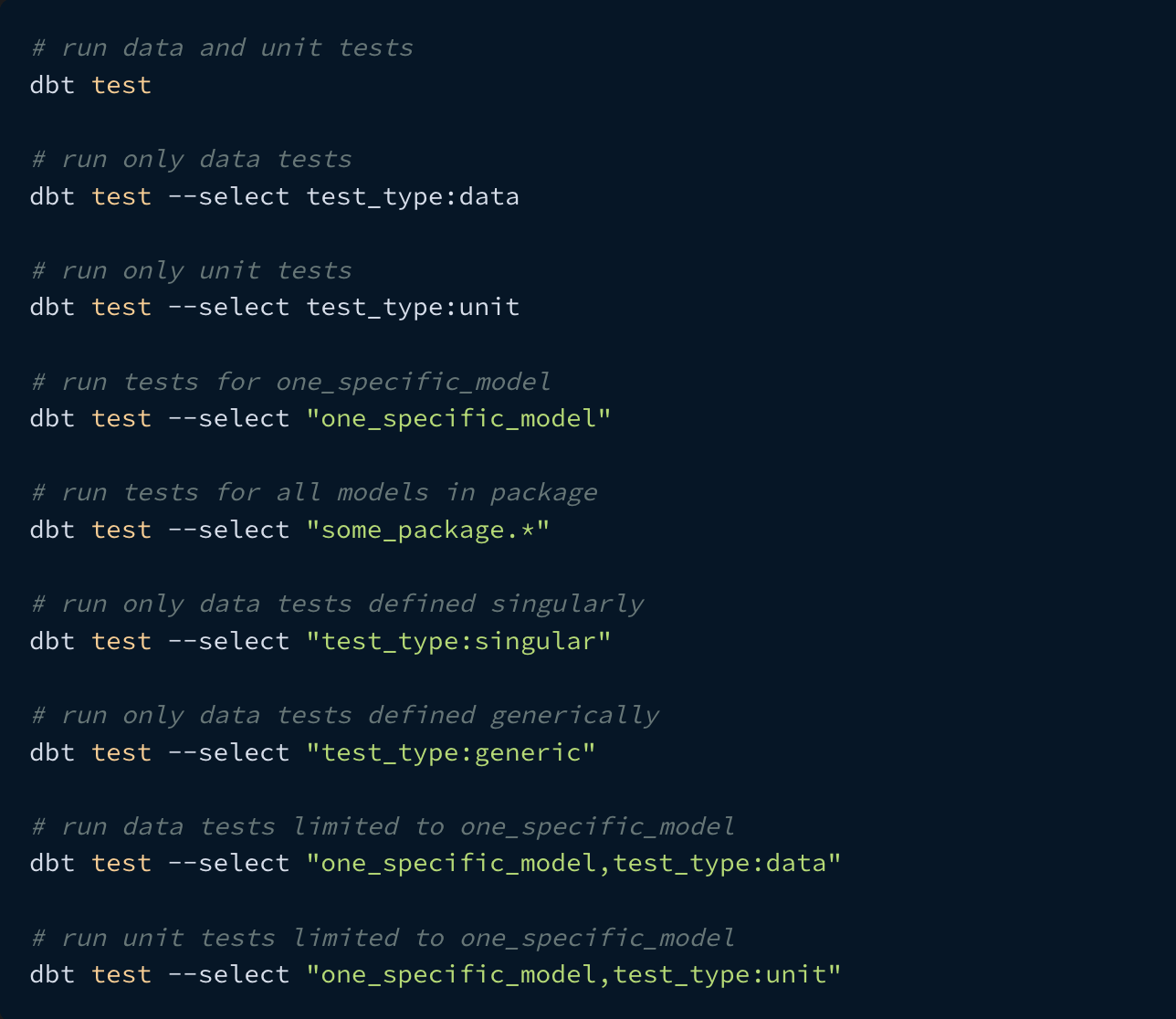
dbt offers a powerful testing framework for data quality assurance. As part of the broader dbt ecosystem, dbt Test allows data teams to define and run automated checks on their data models. These tests can validate data integrity, uniqueness, relationships between tables, and custom business logic. By integrating testing directly into the data transformation workflow, dbt test helps catch issues early, ensuring the reliability of data pipelines. Its seamless integration with version control systems and CI/CD pipelines makes it an essential tool for maintaining data quality in modern data stacks.
Elementary
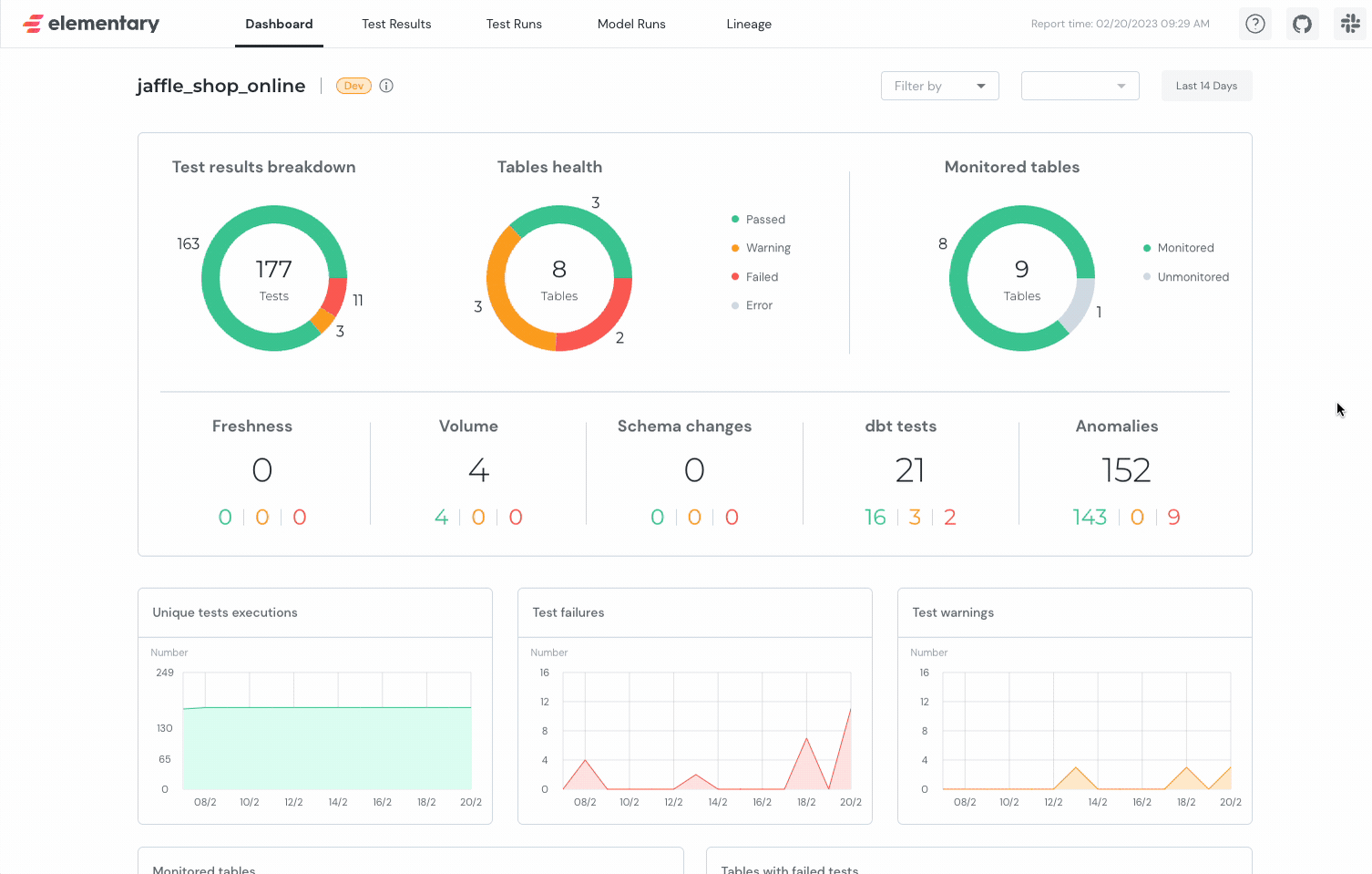
Elementary is an open-source platform for monitoring data quality and pipelines. It works well with dbt, using existing tests and models for thorough data oversight. The tool offers automated anomaly detection, lineage tracking, and custom alerts for quick issue resolution. Its user-friendly interface makes data health visualization accessible to all team members. Elementary is ideal for organizations seeking effective data observability without excessive complexity.
AWS Glue Data Quality
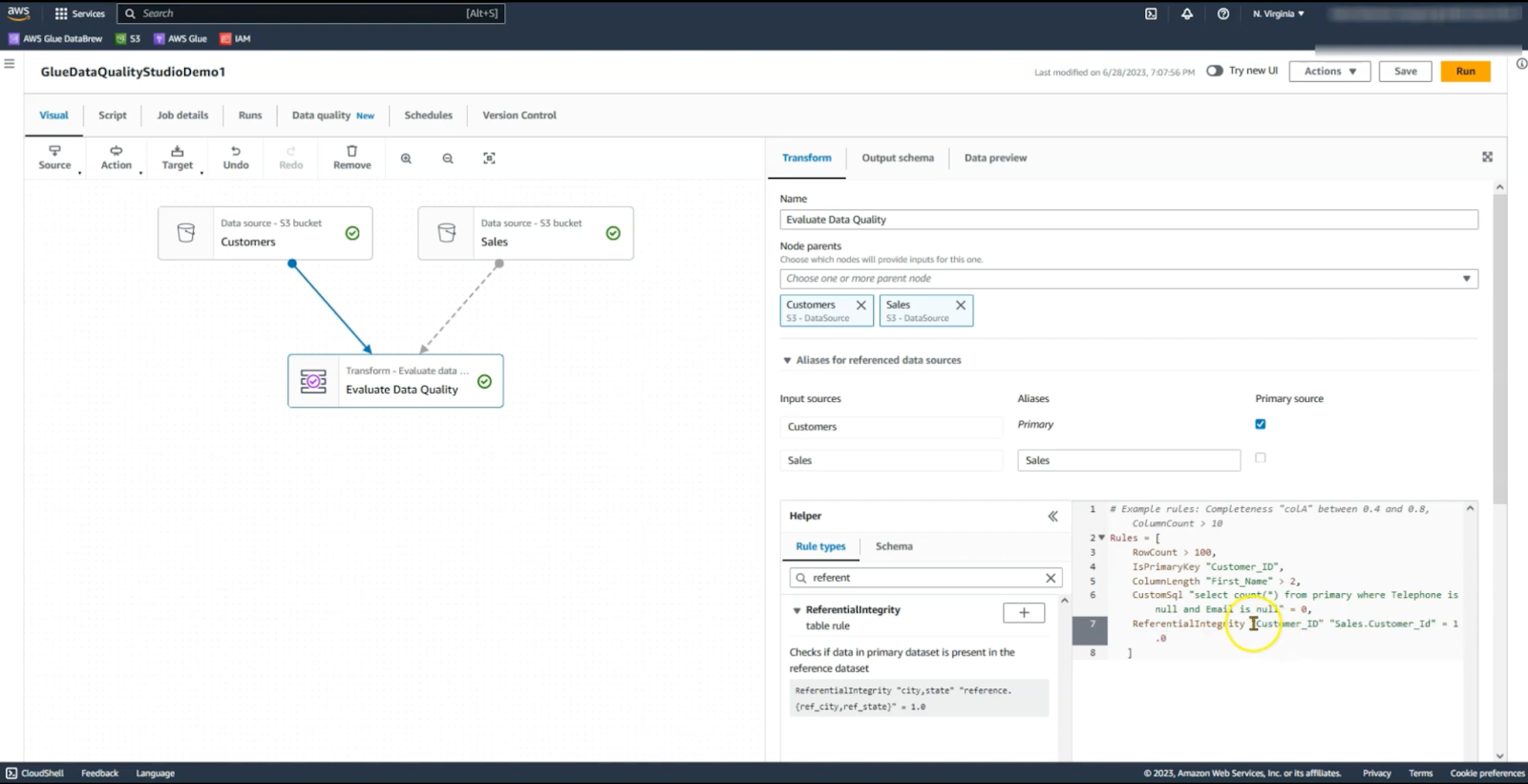
AWS Glue Data Quality is an open-source library for defining "unit tests for data" to maintain quality in large-scale datasets. Built on Apache Spark, it allows data professionals to set and verify data quality constraints. Deequ supports various metrics like completeness, uniqueness, and statistical distribution checks. Its ability to handle big data and integrate with AWS services makes it valuable for organizations using Amazon's cloud ecosystem. The tool's scalability and flexibility in creating custom quality rules ensure data reliability in complex environments.
Great Expectations
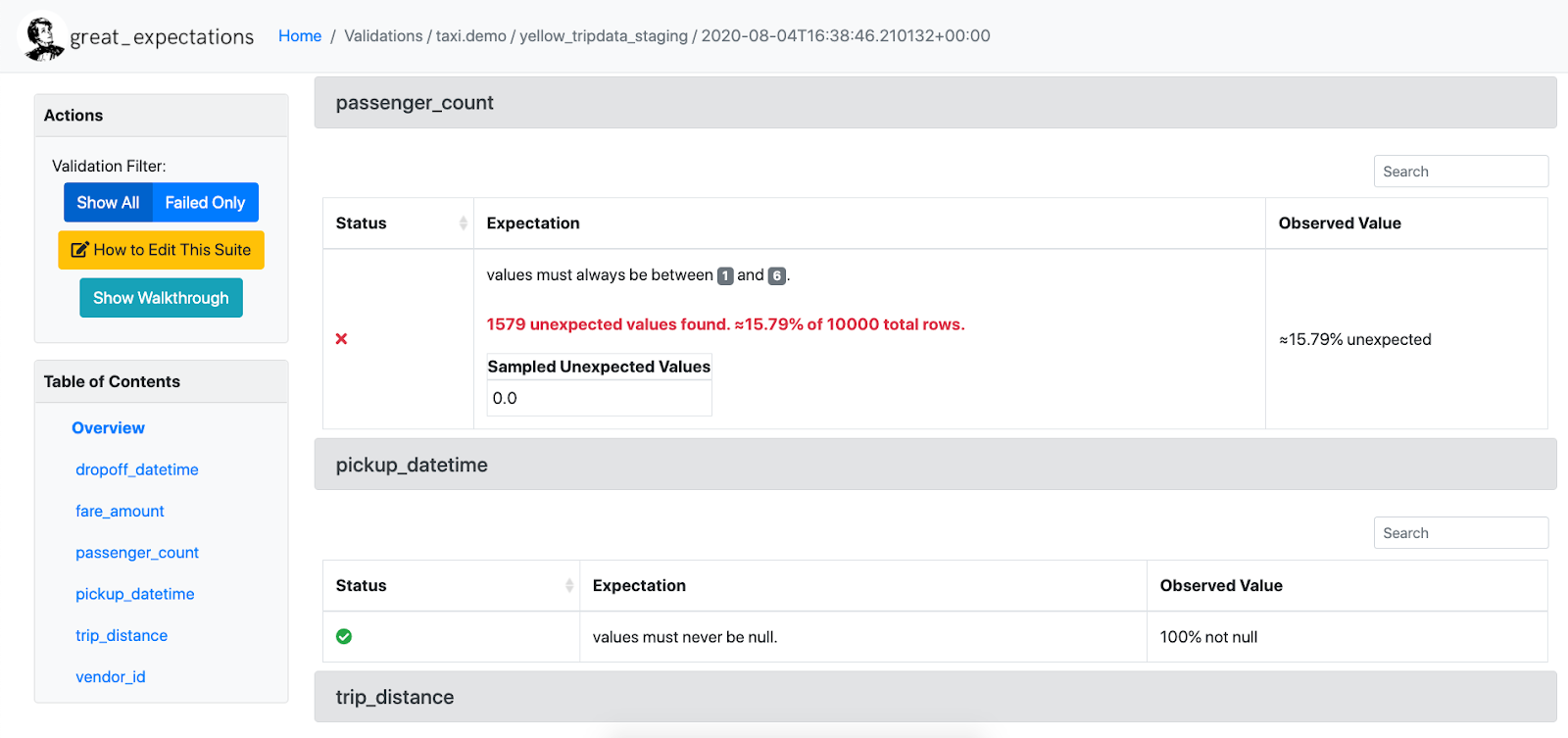
Great Expectations is an open-source Python library for validating, documenting, and profiling data. It allows data teams to define "expectations" - declarative statements about how data should look and behave. These expectations serve as a powerful tool for data testing, documentation, and quality assurance. Great Expectations integrates with various data platforms and workflows, enabling teams to catch data issues early and maintain high data quality standards throughout their pipelines.
Metaplane
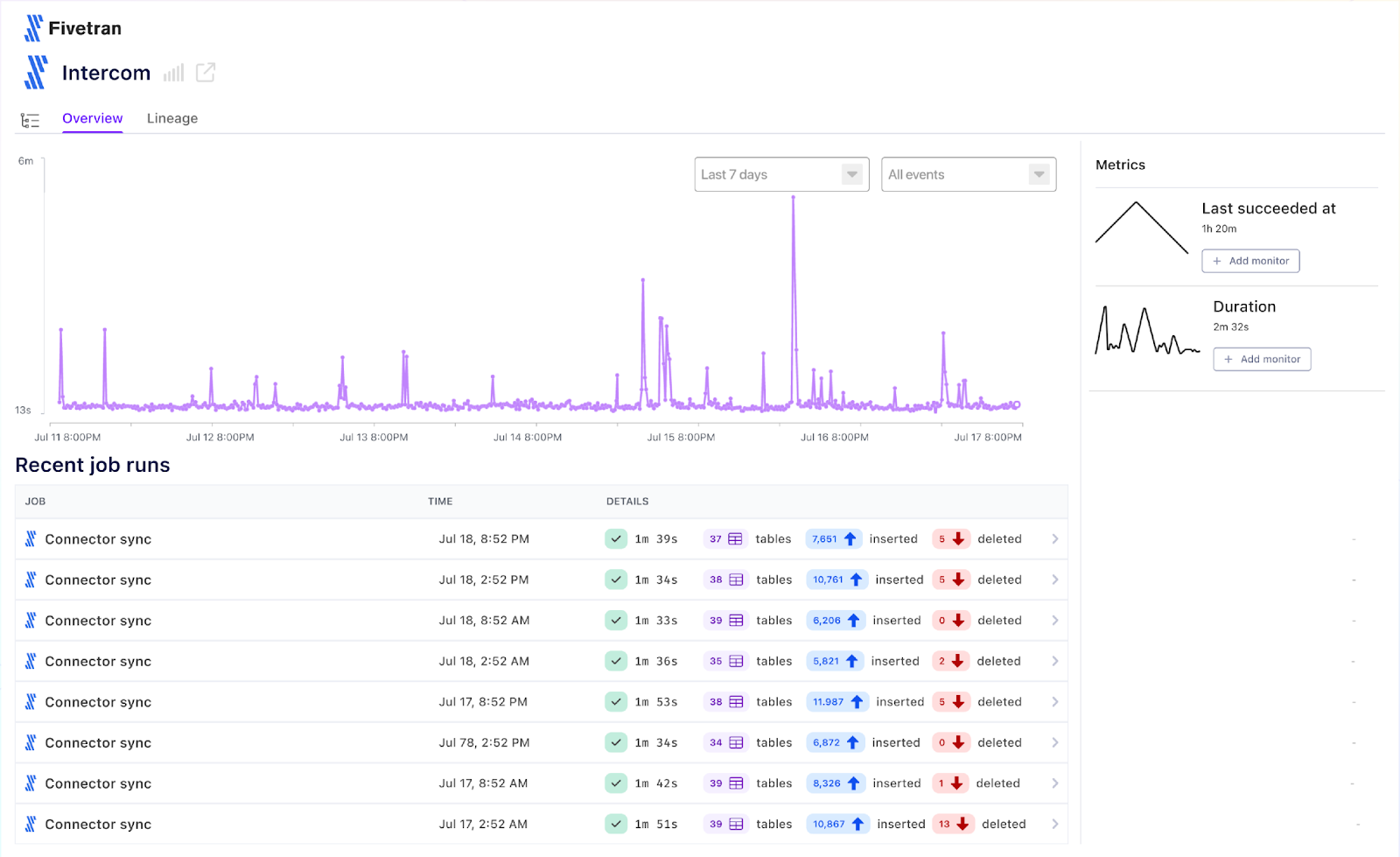
Metaplane offers a comprehensive data observability solution with automated monitoring and smart alerts. It uses machine learning to detect anomalies in data quality, freshness, and volume across various sources. The platform's user-friendly interface allows teams to set up custom monitors easily. With strong integration and collaboration features, Metaplane helps organizations maintain data reliability and foster a data-driven culture.
Monte Carlo Data
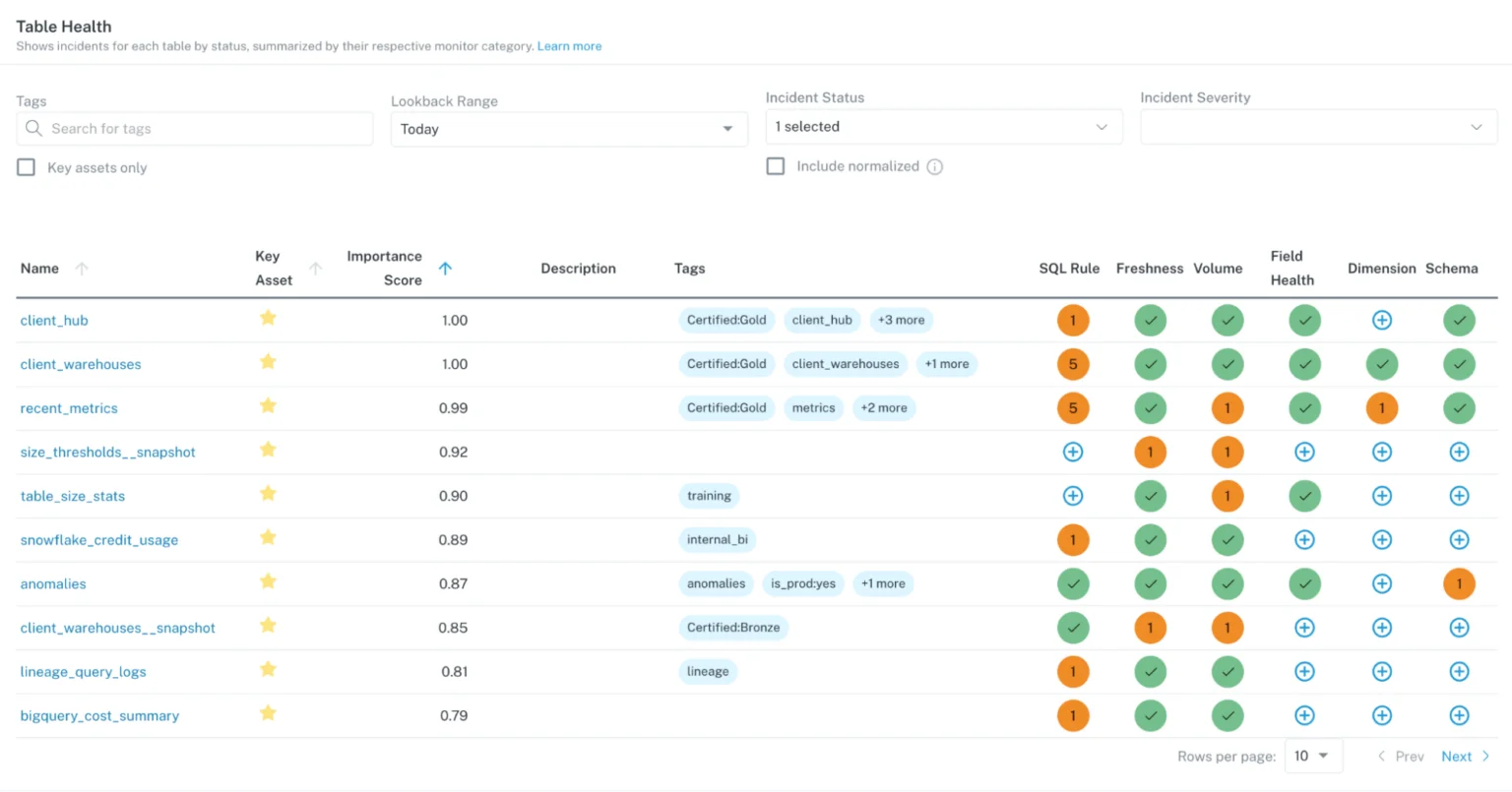
Known for its end-to-end data observability, Monte Carlo Data excels in automated anomaly detection and incident management. The platform's machine learning-enabled capabilities and integrated data lineage tools make it a strong choice for organizations dealing with complex data environments. Monte Carlo Data is particularly well-suited for large enterprises looking to minimize data downtime and improve overall data reliability.
Pantomath
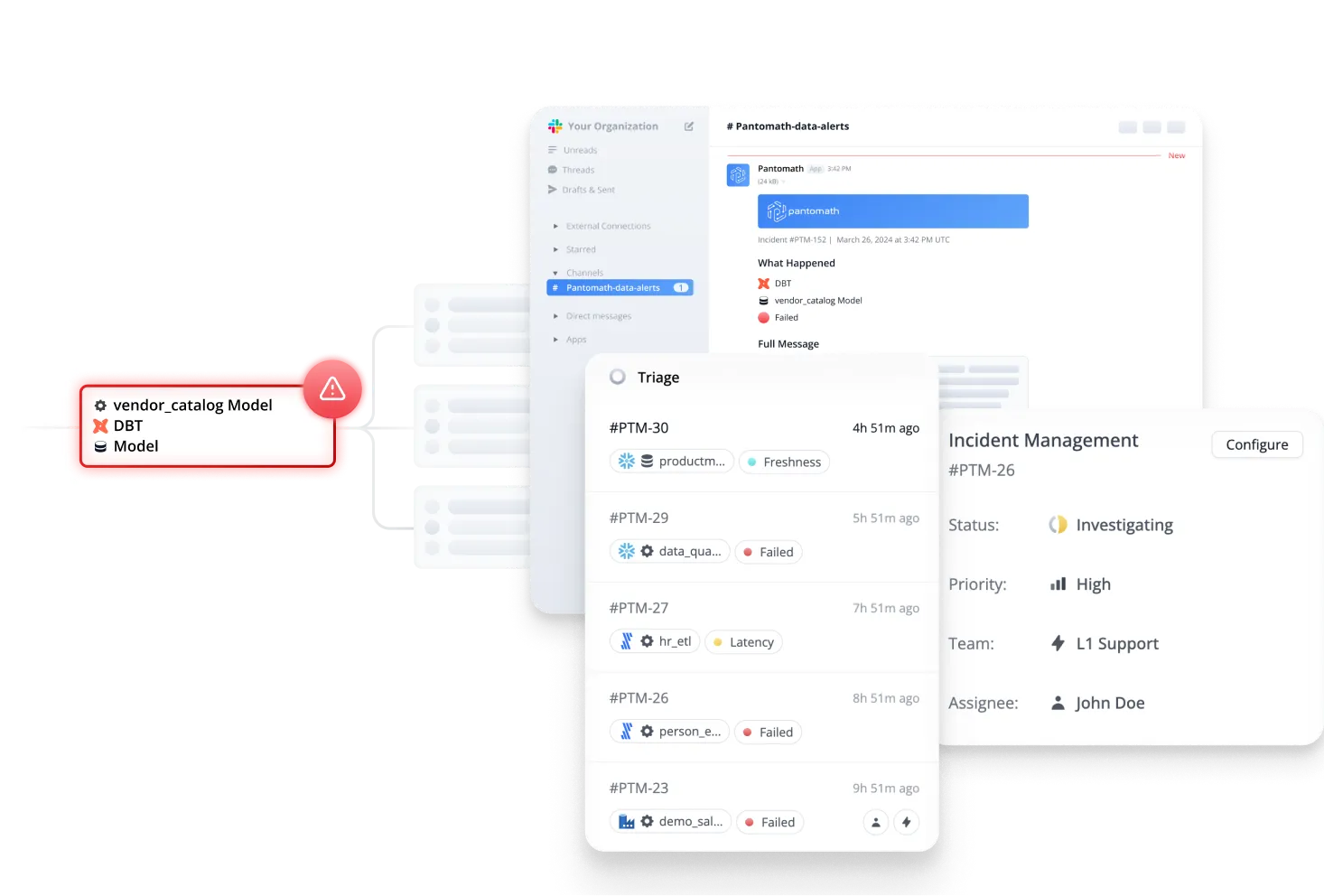
Pantomath blends machine learning and domain expertise for data observability. It offers predictive analytics to flag potential issues early. The platform's smart alerts prioritize critical anomalies, reducing alert fatigue. Adaptable to various data environments, Pantomath suits both startups and large enterprises. Its intuitive interface and custom dashboards provide quick data health insights. Through proactive monitoring and root cause analysis, Pantomath helps maintain data quality and minimize downtime across data ecosystems.
Synq
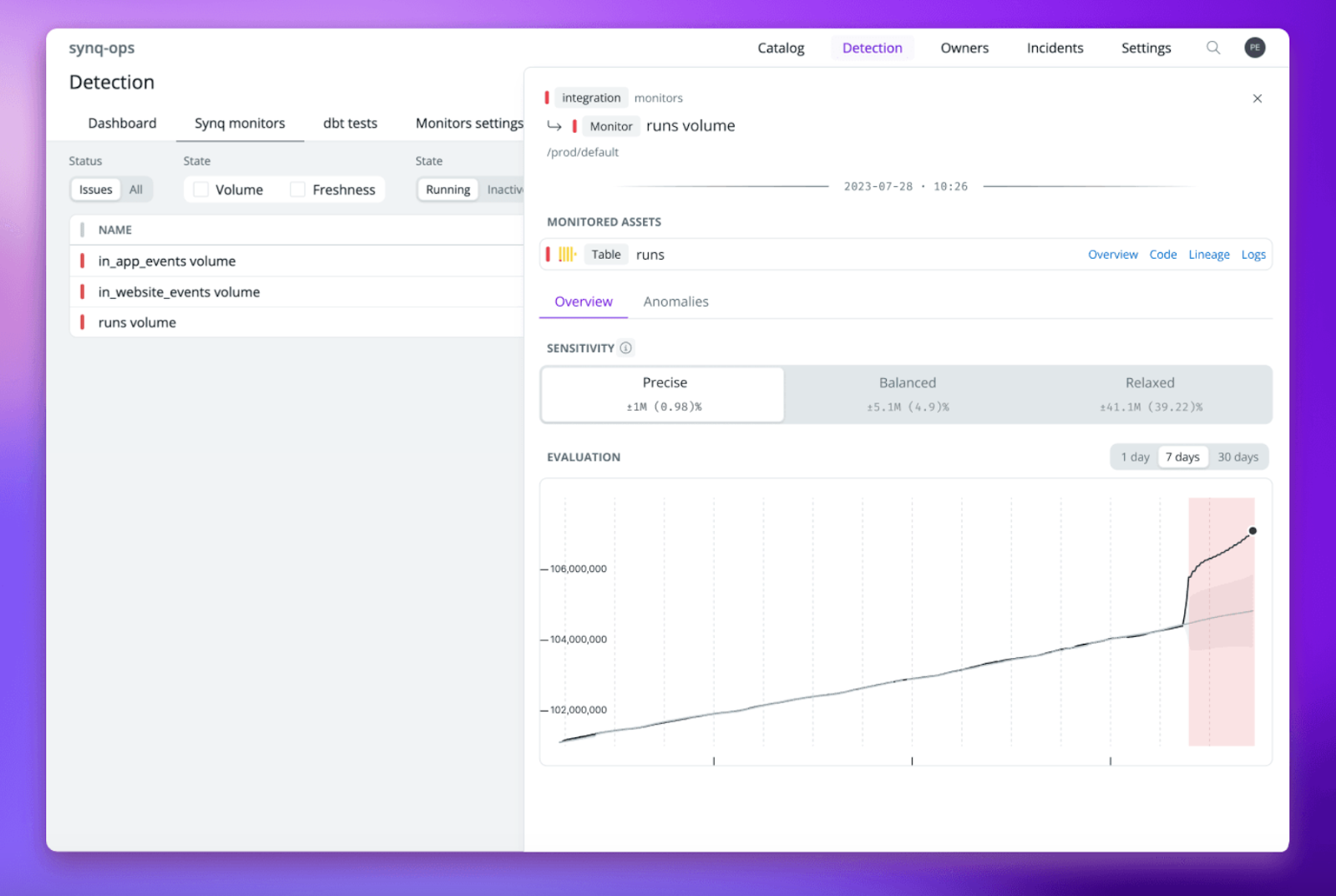
Synq offers a robust data observability solution that focuses on real-time monitoring and anomaly detection. The platform utilizes machine learning algorithms to analyze data patterns and identify deviations from expected behavior. Synq's automated data quality checks and customizable alerting system enable teams to respond quickly to potential issues. The tool's end-to-end data lineage tracking provides valuable insights into data flow and dependencies. With its user-friendly interface and comprehensive reporting features, Synq helps organizations maintain data integrity and optimize their data pipelines.
Telmai
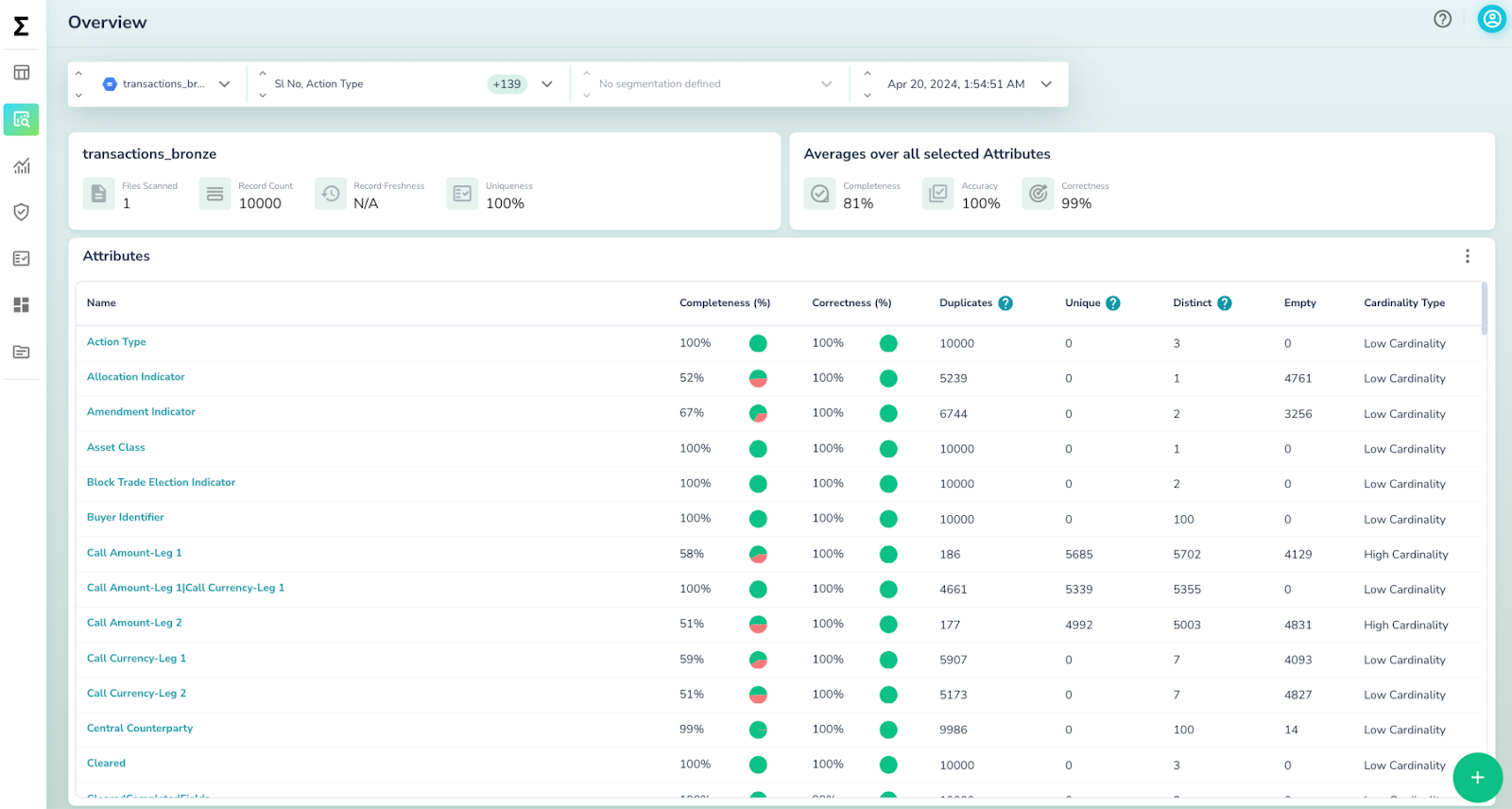
Telmai provides an AI-powered data observability platform designed to automate data quality management and monitoring. The tool offers advanced anomaly detection capabilities, leveraging machine learning to identify data quality issues across diverse data sources. Telmai's no-code approach allows users to easily set up data quality rules and monitoring workflows. The platform's real-time alerting system and collaborative features enable teams to address data issues promptly. With its ability to scale across large datasets and integrate with various data tools, Telmai is well-suited for organizations seeking to enhance their data reliability and governance.
Unravel
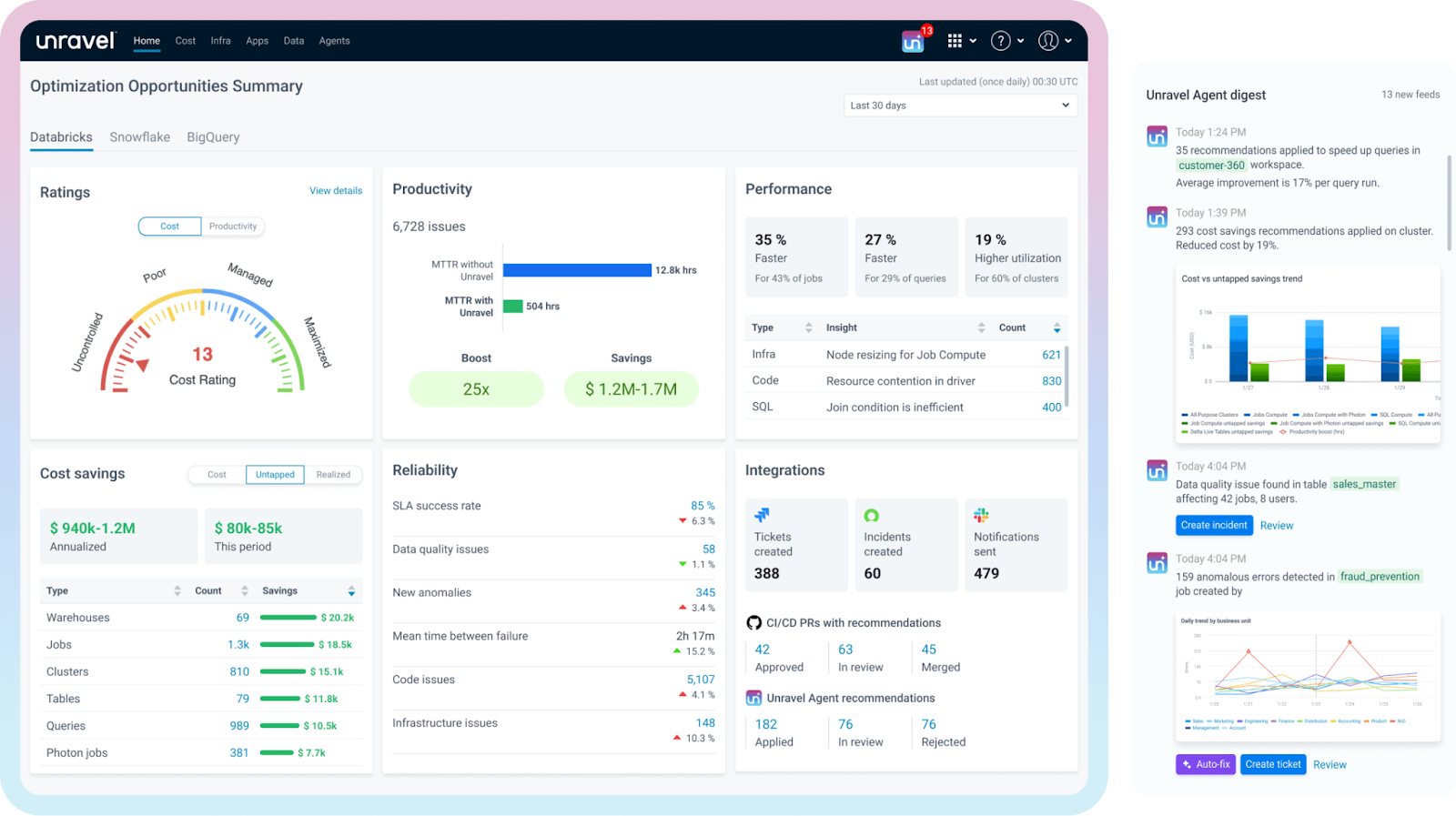
Unravel delivers a comprehensive data operations platform that includes powerful observability features. The tool provides deep insights into data pipelines, applications, and infrastructure performance. Unravel's AI-driven recommendations help organizations optimize resource allocation and improve overall system efficiency. The platform offers end-to-end visibility into data flows, enabling teams to quickly identify and resolve bottlenecks. With its robust cost management capabilities and detailed performance analytics, Unravel empowers organizations to maximize the value of their data investments while ensuring reliable operations.
Validio
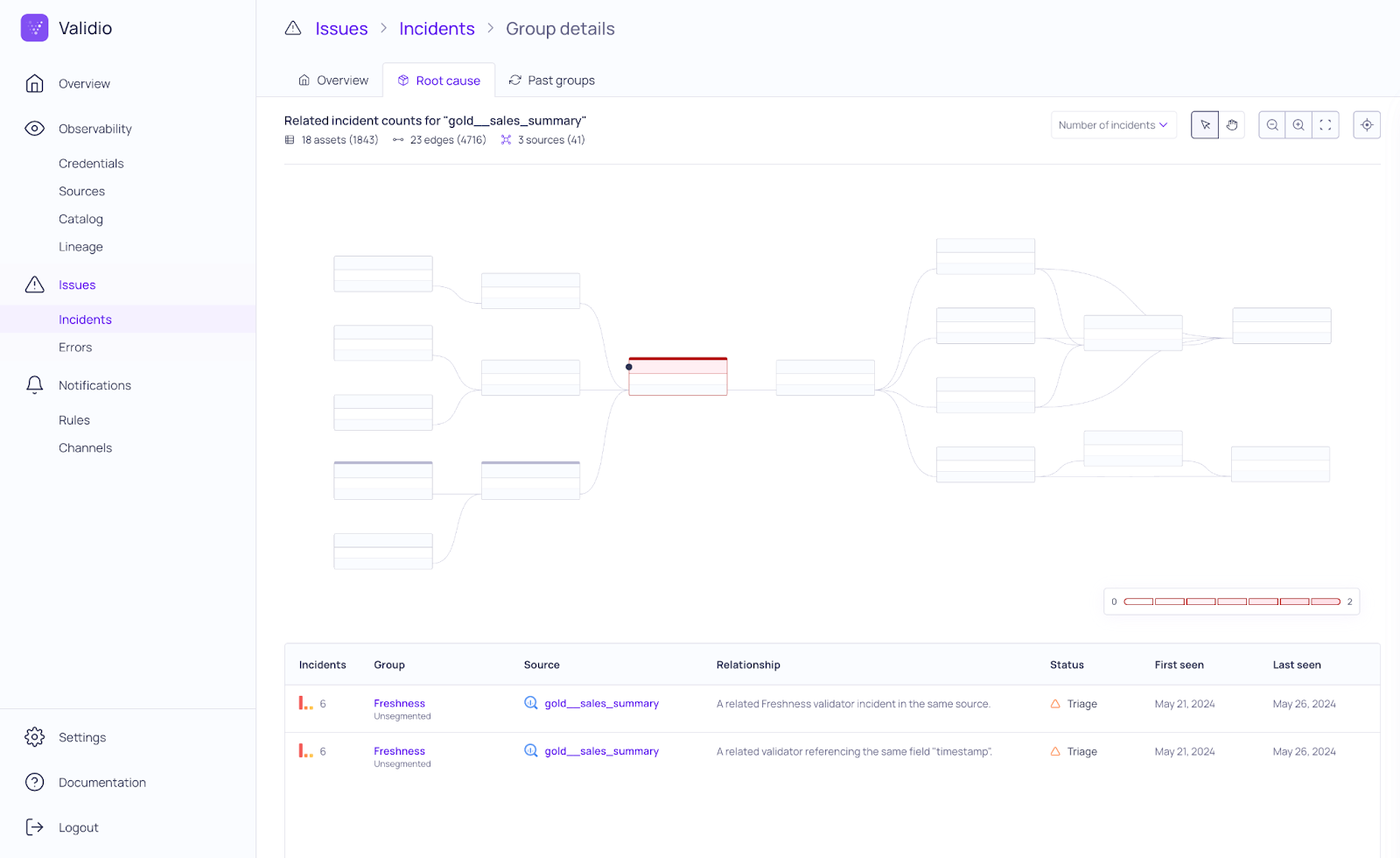
Validio offers a modern data quality platform that combines observability with automated data validation. The tool employs machine learning algorithms to detect anomalies and data drift across various data sources. Validio's schema evolution tracking and data contract validation features help maintain data consistency and reliability. The platform's intuitive interface allows users to easily define and manage data quality rules. With its ability to integrate seamlessly into existing data workflows and provide real-time monitoring, Validio enables organizations to proactively address data quality issues and maintain trust in their data assets.
Selecting the Right Data Observability Tool for Your Organization
When selecting a data observability tool, organizations should consider factors such as scalability, integration capabilities, key features, and budget constraints. It's crucial to evaluate how well a tool aligns with specific business needs and existing data infrastructure. By implementing the right data observability solution, companies can significantly improve their data quality, reduce downtime, and make more informed, data-driven decisions.
As data continues to play an increasingly critical role in business operations, investing in a reliable data observability tool has become a necessity rather than a luxury. These tools not only help in maintaining data integrity but also contribute to building a culture of data trust within organizations. By providing clear insights into data health and usage, observability tools empower teams to work more efficiently and confidently with their data assets.
Choosing a data observability tool requires careful consideration of an organization's unique needs, data ecosystem complexity, and future data management objectives. Keeping abreast of emerging tools and industry best practices in the data observability field is crucial for organizations aiming to make effective, data-driven decisions.
Frequently Asked Questions
What are data observability tools?
Data observability tools are platforms that monitor, analyze, and ensure the health and performance of data systems. They provide insights into data quality, track data lineage, and detect anomalies within data flows, helping organizations maintain data integrity and reliability.
What are the different types of data observability tools?
There are several types of data observability tools, including real-time monitoring platforms, AI-driven anomaly detection systems, data quality management tools, and end-to-end data lineage trackers. Some tools focus on specific aspects of observability, while others offer comprehensive, all-in-one solutions.
What is data observability?
Data observability refers to the ability to understand, monitor, and troubleshoot the health and performance of data systems. It encompasses various aspects such as data quality, lineage, metadata management, and system performance, providing a holistic view of an organization's data ecosystem.