AI-ready data forms the foundation for successful artificial intelligence and machine learning initiatives. It consists of datasets that are clean, well-structured, and organized in a format conducive to effective analysis by AI systems. The key components of AI-ready data include accuracy, completeness, consistency, timeliness, and relevance. These attributes ensure that AI models can derive meaningful insights and produce reliable results. Across industries, as more and more organizations are looking to leverage AI, the need for high-quality, AI-ready data has become increasingly critical. At the forefront of building with data and AI, David Gelman, Director of Data Solutions, and Danny Lee, Director of Growth and Strategy, from Brooklyn Data, share their experience and insights into preparing data for AI applications.
This post is part of Inner Join, a live show hosted by Select Star. Inner Join is dedicated to bringing together thought leaders and experts to explore the latest advancements in the world of data governance and analytics. For more details, visit Select Star's Inner Join page.
Table of Contents
- Debunking the All-or-Nothing Misconception
- Starting Your AI Journey with a Subset of Data
- Preparing Your Data for AI: A Step-by-Step Guide
Debunking the All-or-Nothing Misconception
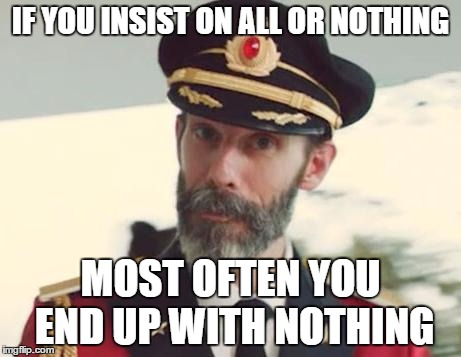
Many organizations mistakenly believe that to implement AI, their entire data ecosystem must be AI-ready. However, this approach can hinder progress and delay valuable insights. Instead, a targeted approach focusing on preparing a subset of high-quality data can yield significant benefits. By starting small, companies can initiate AI projects, build confidence in their capabilities, and progressively expand their data preparation efforts.
Starting Your AI Journey with a Subset of Data
Identify the Right Subset
Selecting an appropriate subset of data is crucial for initiating AI projects. This subset should be relevant to the specific use case and representative of the larger dataset. By focusing on a manageable portion of data, organizations can quickly demonstrate value and learn from the process.
Build a Proof of Concept
Starting with a small, manageable project allows teams to focus on demonstrating value and learning from the process. This approach helps build confidence in AI capabilities and provides valuable insights for future expansion.
Scale Up
As initial AI projects prove successful, organizations can gradually expand their data preparation efforts. This incremental approach allows for continuous improvement of data quality and AI models, ensuring a solid foundation for more extensive implementations.
Preparing Your Data for AI: A Step-by-Step Guide
Readying data for AI applications requires a methodical approach to ensure accuracy, reliability, and usefulness. This process involves several key stages to transform raw data into valuable assets that drive intelligent decision-making and innovation. Let's explore the essential steps to prepare data for AI.
1. Collect and understand your data
The first step in preparing data for AI involves gathering information from reliable and relevant sources. This process includes exploring data using descriptive statistics and visualizations to gain insights into its characteristics. Analyzing data quality and identifying potential biases are crucial steps in this phase.
2. Clean and transform data
Once collected, data must be enhanced and transformed to ensure its suitability for AI applications. This involves addressing missing values, outliers, and inconsistencies. Normalizing and standardizing data, as well as encoding categorical variables, are essential tasks in this stage.
3. Ensure data quality and governance
Implementing data quality rules and automated checks is vital for maintaining the integrity of AI-ready data. Establishing data lineage tracking and defining specific thresholds for data completeness and acceptable ranges contribute to robust data governance practices.
4. Make data accessible and share
Centralizing data access while implementing proper security measures and compliance protocols ensures that AI-ready data is both accessible to authorized users and protected from unauthorized access or breaches.
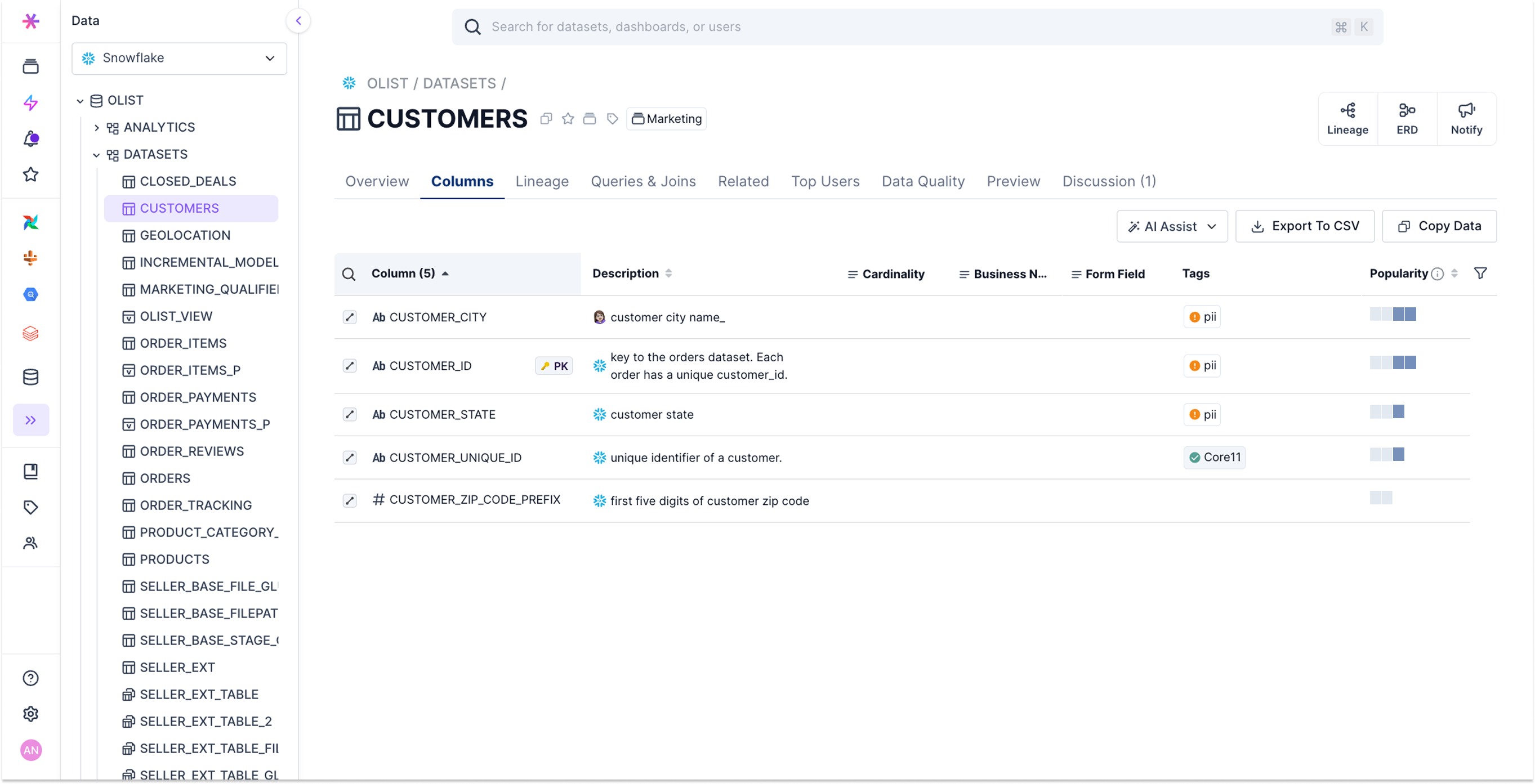
Modern data catalogs like Select Star play a crucial role in managing AI-ready data and models. They serve as central hubs for metadata management and documentation, streamlining the discovery and understanding of data assets across organizations. By providing visibility into data lineage, catalogs help maintain quality, ensure compliance, and optimize models. Usage analytics offered by data catalogs provide insights into data model utilization, identifying high-value assets and improvement opportunities.
Preparing data for AI is a crucial step in harnessing the power of artificial intelligence and machine learning. By focusing on a high-quality subset of data, organizations can effectively begin their AI journey without the need for a complete data overhaul. This approach allows for quick wins, builds confidence, and paves the way for more extensive AI implementations in the future. As data modeling principles continue to evolve, staying informed about emerging trends and best practices will be essential for organizations looking to derive maximum value from their AI initiatives. Let's embrace these advancements and guide your organization towards a data-driven future.