Driving a data-driven culture requires more than just collecting information; it demands a strategic approach to data management, analysis, and communication. As a Data Science Tech lead at Square, Kasia Rachuta shares valuable insights, including five essential tools for empowering data teams and cultivating a thriving data-driven environment: ETL documentation, structured A/B testing, defining key metrics, proactive communication strategies, and AI-assisted data operations.
This post is part of INNER JOIN, a live show hosted by Select Star. INNER JOIN brings together thought leaders and experts to discuss the latest trends in data governance and analytics. For more information, visit Select Star's LinkedIn page.
Table of Contents
- ETL Documentation: A Foundation for Data Quality
- Structured A/B Testing: From Intuition to Evidence
- Metrics: The Backbone of Data-Driven Decision Making
- Communication: Strategies for Fostering Data Literacy Across Teams
- AI-Assisted Tools: Enhancing Data Team Efficiency and Insights
- Overcoming Challenges in Data Culture Adoption
1. ETL Documentation: A Foundation for Data Quality

Effective Extract, Transform, Load (ETL) documentation serves as the cornerstone of data quality and transparency. Comprehensive documentation in maintaining clarity and consistency across an organization's data ecosystem is important. A well-structured ETL document should include:
- Goals and non-goals sections
- Key analytical questions addressed
- Data quality check guidelines
By clearly defining the purpose and scope of each ETL process, teams can avoid misunderstandings and ensure that data transformations align with business objectives. The non-goals section helps prevent scope creep and clarifies what the ETL process is not intended to accomplish. Including key analytical questions in the documentation helps data consumers understand how to leverage the transformed data effectively.
Data quality check guidelines embedded within the documentation act as a safeguard against errors and inconsistencies. These guidelines prompt data engineers to consider potential issues and implement necessary checks, fostering a culture of data integrity from the outset.
Read Kasia’s post on ETL documentation here.
2. Structured A/B Testing: From Intuition to Evidence
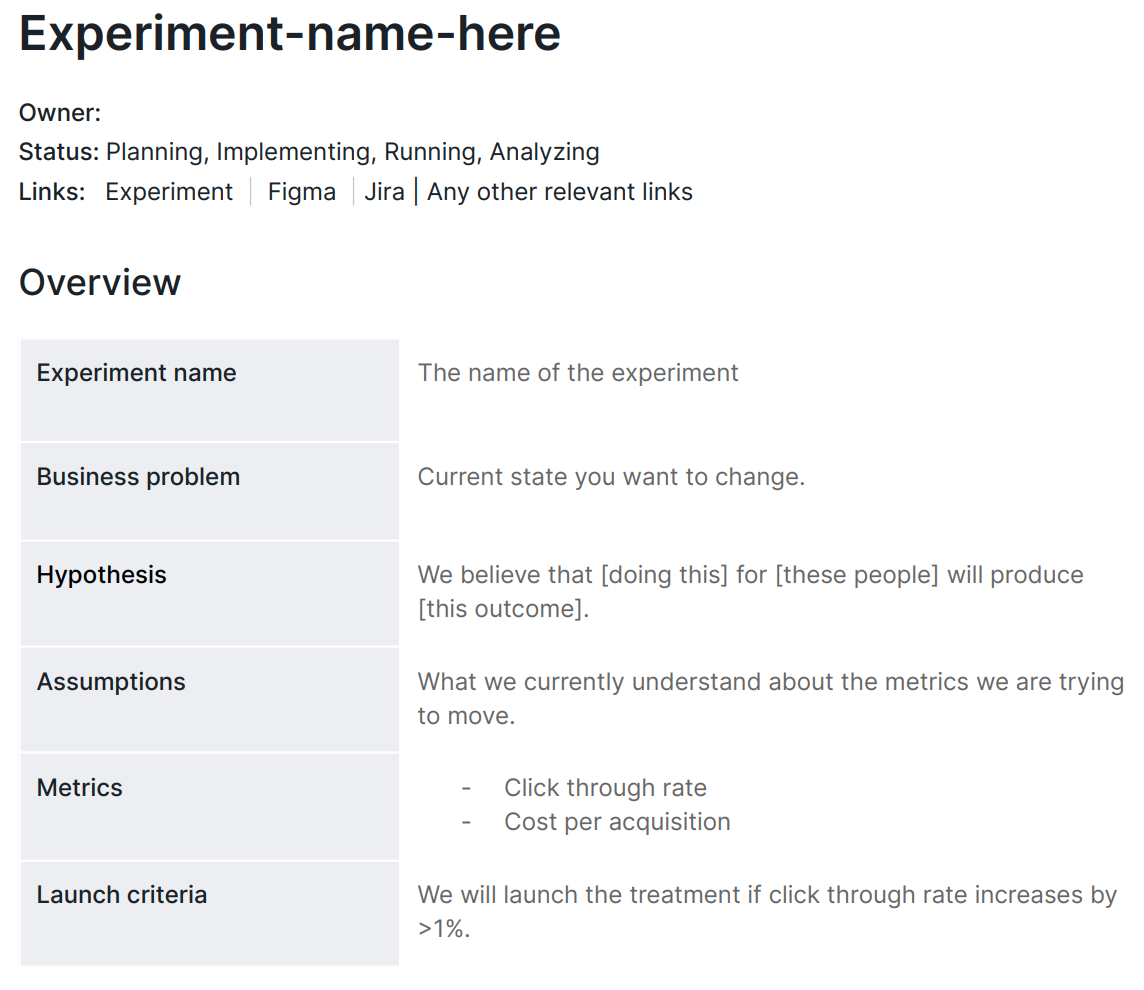
Implementing a structured approach to A/B testing marks a significant shift from intuition-based decision-making to evidence-driven strategies. Kasia’s team at Square transformed their A/B testing process by incorporating metrics directly into product requirements documents. This practice ensures that data considerations are at the forefront of product development from the earliest stages.
Clearly defined roles and responsibilities within the A/B testing framework eliminate ambiguity and streamline the testing process. By delineating the specific tasks assigned to product managers, data scientists, and engineers, teams can execute tests more efficiently and derive meaningful insights.
Collaboration between data scientists and product teams is crucial for successful A/B testing. This partnership allows for the alignment of business goals with data-driven methodologies, resulting in more impactful experiments and actionable results.
3. Metrics: The Backbone of Data-Driven Decision Making
Establishing and tracking key metrics forms the backbone of data-driven decision-making. It’s important to define both health metrics and leading indicators to gain a comprehensive view of business performance. Health metrics provide a high-level overview of the organization's status, such as revenue growth, customer retention rates, and employee satisfaction scores. Meanwhile, leading indicators offer insights into potential future trends, helping teams anticipate and prepare for upcoming challenges or opportunities. Examples of leading indicators include website traffic growth, new user sign-up rates, customer engagement metrics like time spent in-app, and changes in search volume for product-related keywords.
Regular review and iteration of metrics ensure their continued relevance and effectiveness. As business needs evolve, so too should the metrics that guide decision-making. This iterative approach allows organizations to stay agile and responsive to changing market conditions.
The inclusion of metrics in product and engineering documents fosters a data-centric mindset across teams. By making data considerations an integral part of all projects, organizations can create a culture where data-driven decision-making becomes second nature.
4. Communication: Strategies for Fostering Data Literacy Across Teams
Proactive communication strategies play a vital role in fostering data literacy throughout an organization. Here are five approaches implemented at Square:
- One-on-one onboarding sessions for stakeholders: These personalized meetings introduce key data concepts, metrics, and tools specific to each stakeholder's role. Sessions cover how to access and interpret relevant data, fostering confidence in data-driven decision-making.
- Slack announcements for metric changes: Regular updates in dedicated data channels highlight significant shifts in key metrics, providing context and potential impact. This real-time communication keeps teams informed and aligned on data trends.
- Quarterly all-hands presentations on data updates: These comprehensive sessions showcase major data initiatives, success stories, and lessons learned. They also introduce new data tools or methodologies, reinforcing the organization's commitment to data-driven culture.
- Data literacy workshops: Periodic training sessions that cover fundamental data concepts, statistical analysis, and data visualization techniques, catering to various skill levels across the organization.
- Data office hours: Weekly slots where data team members are available to answer questions, provide guidance on data usage, and assist with data-related challenges, promoting a supportive learning environment.
These targeted communication efforts ensure that all team members, regardless of their technical background, have a clear understanding of key metrics and their implications. By making data accessible and comprehensible, organizations can empower employees at all levels to make informed decisions based on data insights.
5. AI-Assisted Tools: Enhancing Data Team Efficiency and Insights
The integration of AI-powered tools presents exciting opportunities for transforming data team operations. Soon, AI will automate routine data queries and tasks, freeing up data scientists to focus on deeper analytical work.
AI-assisted tools can help answer common questions about data sources, definitions, and usage, reducing the time data teams spend on repetitive inquiries. This automation not only increases efficiency but also allows data professionals to engage in more strategic and impactful projects.
However, it is important to balance automation with critical thinking. While AI can streamline many processes, human expertise remains crucial for interpreting results, identifying nuances, and making strategic decisions based on data insights.
Overcoming Challenges in Data Culture Adoption
Implementing a data-driven culture is not without its challenges. Here are 3 ways to overcome common obstacles:
- Securing leadership buy-in for data initiatives is crucial. By demonstrating the tangible business impact of data-driven decision-making, data teams can gain support from top management and secure necessary resources.
- Iterating on approaches for different teams recognizes that one size does not fit all when it comes to data culture. Tailoring strategies to the specific needs and workflows of various departments ensures more effective adoption of data-driven practices.
- Continuous education and reinforcement are essential for maintaining a strong data culture. Regular training sessions, workshops, and knowledge-sharing initiatives help keep data literacy at the forefront of organizational priorities.
The journey to fostering a robust data culture is ongoing and requires persistence, adaptability, and collaboration across all levels of an organization. By implementing these five tools – comprehensive ETL documentation, structured A/B testing, well-defined metrics, proactive communication strategies, and AI-assisted operations – data teams can drive meaningful change and empower their organizations to harness the full potential of their data assets.
As data ecosystems continue to grow in complexity, the role of data teams in driving business impact becomes increasingly critical. By embracing these tools and best practices, organizations can create a foundation for data-driven success, enabling more informed decision-making and fostering a culture of continuous improvement and innovation. To learn how Select Star can help your organization implement these strategies and enhance your data management capabilities, book a demo today.