
Coalesce 2024, dbt Labs' annual conference, attracted a diverse crowd of over 1,800 data professionals to Las Vegas, while thousands more participated virtually. The event's central theme, "One dbt," highlighted the growing need for cross-platform collaboration and AI integration in the data industry. CEO of dbt Labs Tristan Handy delivered a keynote that outlined a comprehensive vision for the future of data management, emphasizing seamless interoperability and highlighting persistent challenges in the field, such as ensuring data quality across systems and establishing clear data ownership in complex environments. The conference served as a platform for introducing innovative solutions aimed at unifying data workflows and enhancing collaboration among data teams, regardless of their chosen tech stack or cloud environment.
Table of Contents
- The Analytics Development Lifecycle (ADLC)
- Innovations in Data Control and Visualization
- AI Integration in Data Workflows
- Semantic Layer Advancements
- Real-world Implementations Shared at dbt Coalesce 2024
- Conclusion
The Analytics Development Lifecycle (ADLC)
Analytics Development Lifecycle (ADLC) took center stage at Coalesce 2024, presenting a structured approach to standardize and scale analytics workflows. This framework addresses long-standing challenges in the data industry, including poor data quality, lack of data literacy, and ambiguous data ownership. By implementing ADLC, organizations can establish a more mature, integrated analytics workflow that promotes consistency and reliability across their data ecosystem. dbt Cloud supports this lifecycle by offering a unified experience that spans various data platforms and cloud environments, enabling seamless collaboration and enhancing data governance practices.
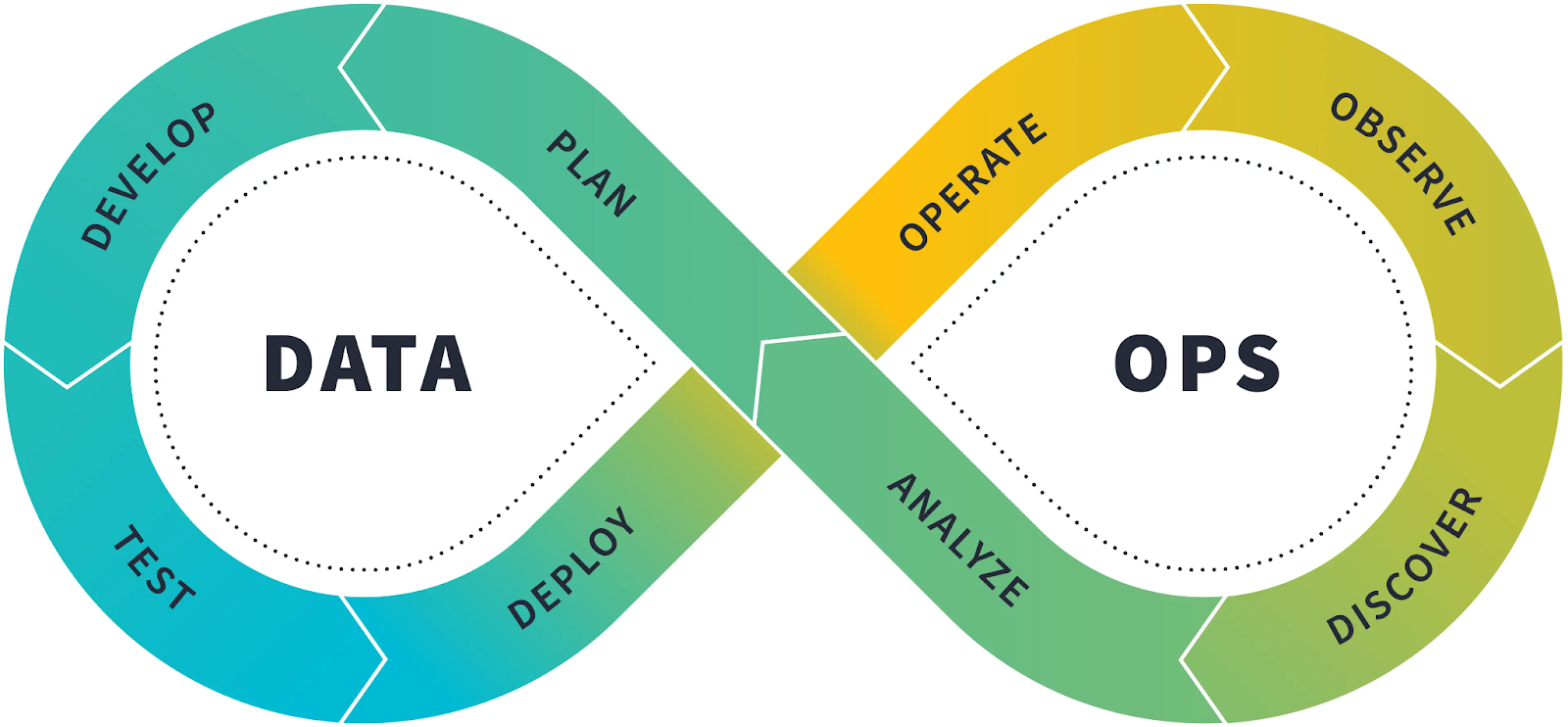
The ADLC approach aligns with dbt’s vision of "One dbt," fostering a cohesive data strategy that adapts to diverse technical landscapes. For a deeper dive into the Analytics Development Lifecycle and its impact on modern data practices, check out dbt’s comprehensive guide.
Innovations in Data Control and Visualization
Central to dbt's new offerings is the positioning of dbt Cloud as a data control plane for enterprise analytics. This approach centralizes metadata management, enabling cross-platform orchestration and observability across diverse data ecosystems. A standout feature is the new visual editing experience, currently in beta. This low-code, drag-and-drop interface allows less technical users to build and explore dbt models without extensive SQL knowledge, democratizing data transformation processes. Importantly, these visual models compile to SQL and adhere to version control practices, maintaining data governance standards while broadening participation in data workflows. For organizations looking to implement this visual editing approach, dbt offers a comprehensive guide on getting started with dbt Cloud.

AI Integration in Data Workflows
dbt Copilot, an AI engine integrated into dbt Cloud, is designed to boost productivity in analytics workflows. This innovative tool, currently in beta, brings several AI-powered capabilities to data teams. It can automatically generate tests for data quality assurance, create comprehensive documentation for models and transformations, and build semantic models to standardize metrics across an organization. The AI chatbot feature allows users to query their data using natural language, making data exploration more intuitive for both technical and non-technical team members. Additionally, dbt Copilot offers integration with OpenAI through custom API keys, enabling organizations to leverage advanced language models for various data-related tasks. These features collectively aim to reduce manual work, improve data quality, and accelerate the development of analytics projects. As the tool evolves, it has the potential to significantly transform how data teams interact with and manage their analytics workflows.

Semantic Layer Advancements
dbt announced a collaboration with Salesforce to integrate the dbt Semantic Layer with Salesforce Data Cloud, Tableau, and Agentforce. This partnership aims to create a unified data ecosystem with consistent metrics across platforms. By incorporating dbt models and metrics directly into Salesforce products, users can expect improved data trust and value. The partnership's goal is to eliminate data silos and foster collaboration between data teams and business users. By providing a centralized source for metrics and data definitions, organizations can benefit from better decision-making, fewer data inconsistencies, and quicker insights throughout their data infrastructure.
Real-world Implementations Shared at dbt Coalesce 2024
Coalesce 2024 featured an impressive lineup of real-world dbt implementations, showcasing how organizations are leveraging the tool to transform their data practices. We’ll highlight four diverse use cases: managing over 1,000 dbt models, enhancing FinOps at Workday, governing multiple dbt projects at nib Group, and integrating dbt for proactive data observability at SurveyMonkey. If you missed the live event or want to revisit talks, recordings are available online.
1. Managing 1,000+ dbt models
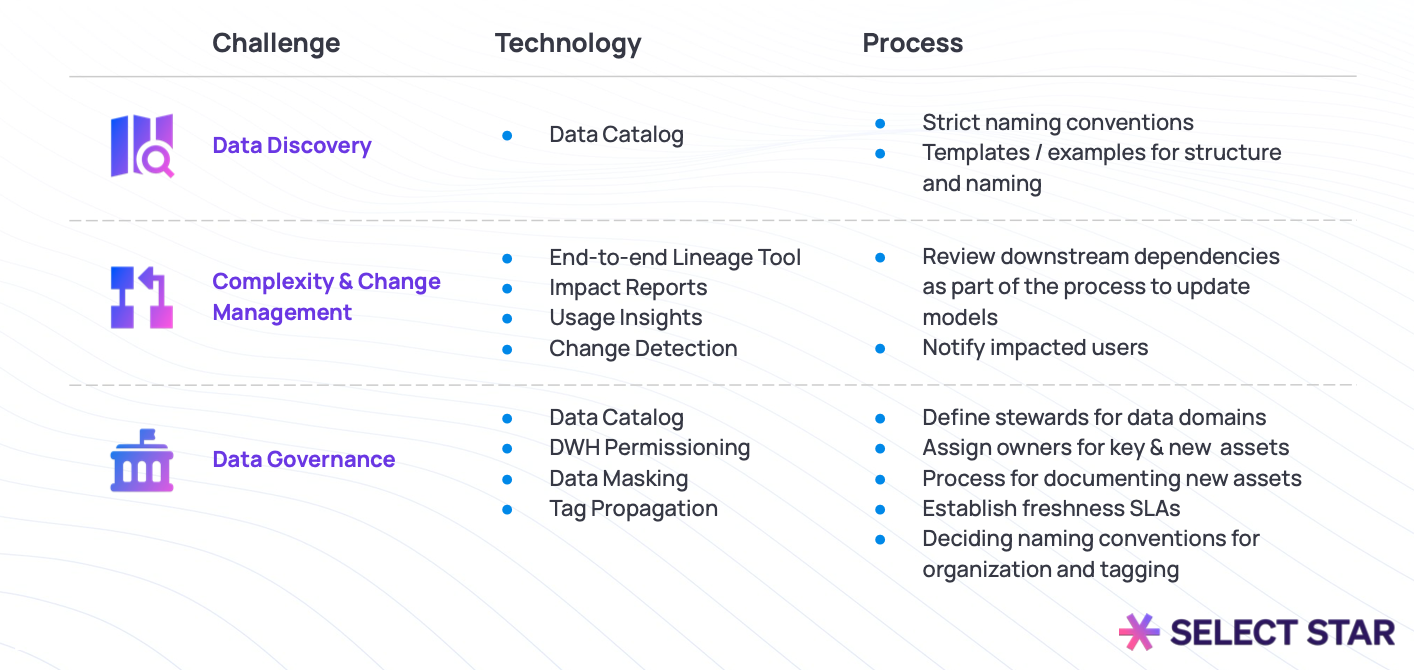
Rya Sciban, Head of Product at Select Star, shared insights on scaling dbt implementations to manage over 1,000 models. The key challenges highlighted in managing such a large number of models were complexity and change management, data discovery, and data governance. To address these challenges, Rya outlined several strategies.
Strategies for addressing these challenges include:
- Implement clear naming conventions and folder structures - This involves creating a hierarchy that groups models by purpose, business unit, or data source. For example, using prefixes like "dim_", "fact_", "stg_", or "int_" can quickly convey the type and stage of each model.
- Use data catalogs for visibility across the entire data stack - This allows all data users in the organization to understand what data exists, its meaning, and its relationships. A comprehensive data catalog can include information from various sources, from raw data ingestion to final BI tools.
- Leverage data lineage tools for impact analysis and change management - These tools help visualize dependencies between models, making it easier to understand how changes in one model might affect others downstream. This visibility is crucial when planning updates or troubleshooting issues.
See the full talk on YouTube for more.
2. Leveraging dbt for FinOps at Workday
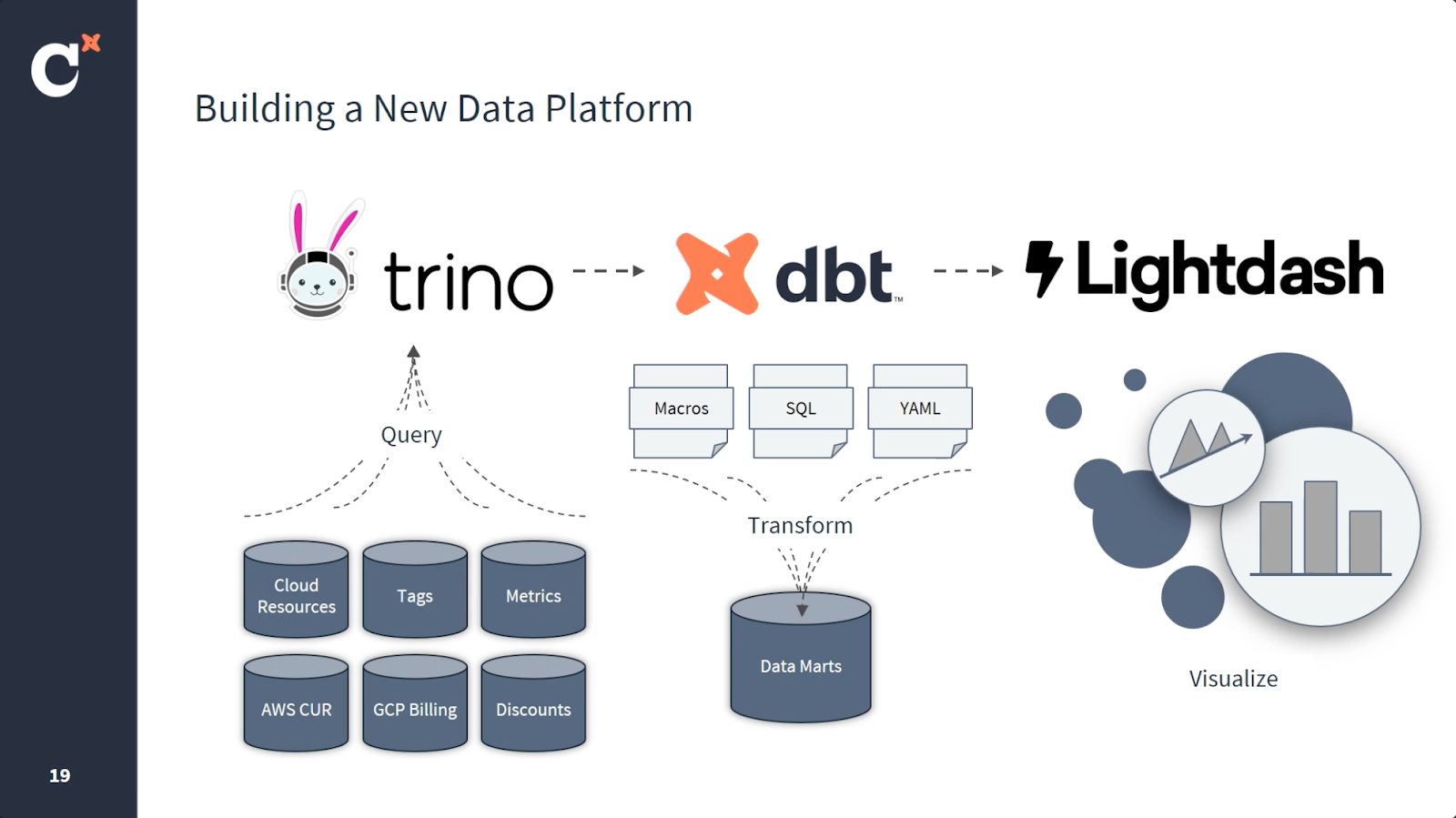
Workday leveraged dbt to enhance their FinOps practices and conduct in-depth cost analysis across their multi-cloud infrastructure. They created a series of dbt models to ingest, transform, and standardize billing data from various cloud providers like AWS, Azure, and Google Cloud. These models included steps to normalize cost categories, allocate expenses to specific departments or projects, and calculate key metrics such as cost per service and utilization rates. By utilizing dbt's incremental materialization strategy, Workday ensured that their cost data remained up-to-date without unnecessary recomputation. They also implemented dbt tests to validate data integrity and catch anomalies in billing information. This comprehensive dbt implementation enabled Workday to generate detailed reports and dashboards, providing actionable insights for optimizing cloud spend and improving resource allocation across their organization. Check out the session on YouTube for more.
3. Governing dozens of dbt projects at nib
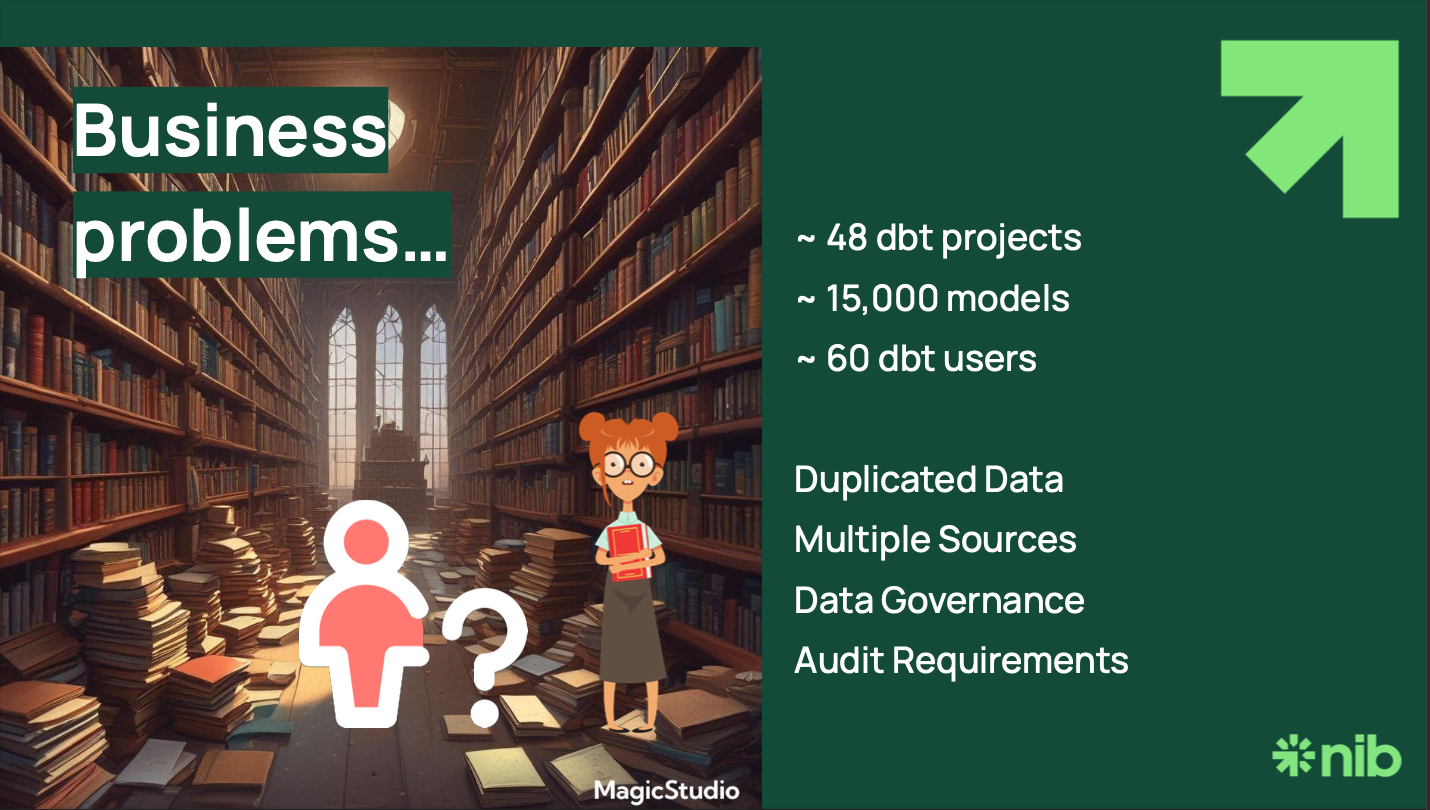
A presentation from Data Product Manager Pip Sidaway and Lead Data Engineer Juliana Zhu at nib highlighted their approach to governing dozens of dbt projects and environments over five years. Their comprehensive strategy emphasizes several key aspects of data management.
- Tagging and classification of data assets: This enables efficient organization and retrieval of information. This approach helps maintain clarity in complex data ecosystems.
- Automated processes for propagating tags and enforcing data contracts: This automation ensures consistency across projects and reduces manual errors
- Custom tooling for privilege management and impact reporting: These tools allow for granular control over data access and provide detailed insights into how changes affect the overall data landscape.
Additionally, nib shared how they've implemented a "tag propagation party" to engage team members in the classification process, fostering a culture of data governance. They also highlighted the importance of establishing clear data stewards for different domains and implementing a robust process for documenting new data assets. This multi-faceted approach has enabled nib to scale their dbt implementation effectively while maintaining strong governance practices. Head over to YouTube for the session recording.
4. Integrating dbt for proactive data observability at SurveyMonkey

SurveyMonkey showcased how they utilized dbt to enhance their data observability practices. By implementing dbt tests and documentation features, they created a robust system for monitoring data quality and lineage across their data pipeline. SurveyMonkey's data team developed custom macros to automate the creation of data quality checks, ensuring consistency across their models. They also leveraged dbt's exposure feature to map out critical data assets and their dependencies, providing clear visibility into how data flows through their system. This implementation not only improved data reliability but also facilitated faster issue resolution and increased trust in their data among stakeholders. The team reported a significant reduction in data-related incidents, with a 40% decrease in time spent on troubleshooting data discrepancies. Furthermore, the enhanced observability allowed them to proactively identify and address potential data quality issues before they impacted downstream processes. SurveyMonkey's success with dbt for data observability has inspired them to expand its use across other areas of their data infrastructure, fostering a culture of data quality throughout the organization. Hear more in SurveyMonkey's session on YouTube.
Conclusion
Coalesce 2024 showcased significant advancements of dbt, and the analytics engineering community. The introduction of cross-platform dbt Mesh, visual editing capabilities, and AI-driven features like dbt Copilot signal a future where data workflows are more accessible and efficient. As organizations continue to grapple with complex, multi-platform environments, tools that unify workflows and enhance collaboration will be crucial for maintaining data quality and trust.
We’re excited for Coalesce 2025! In the meantime, if you're interested in how Select Star can help scale your dbt instances and support your data journey, schedule a demo to learn more.