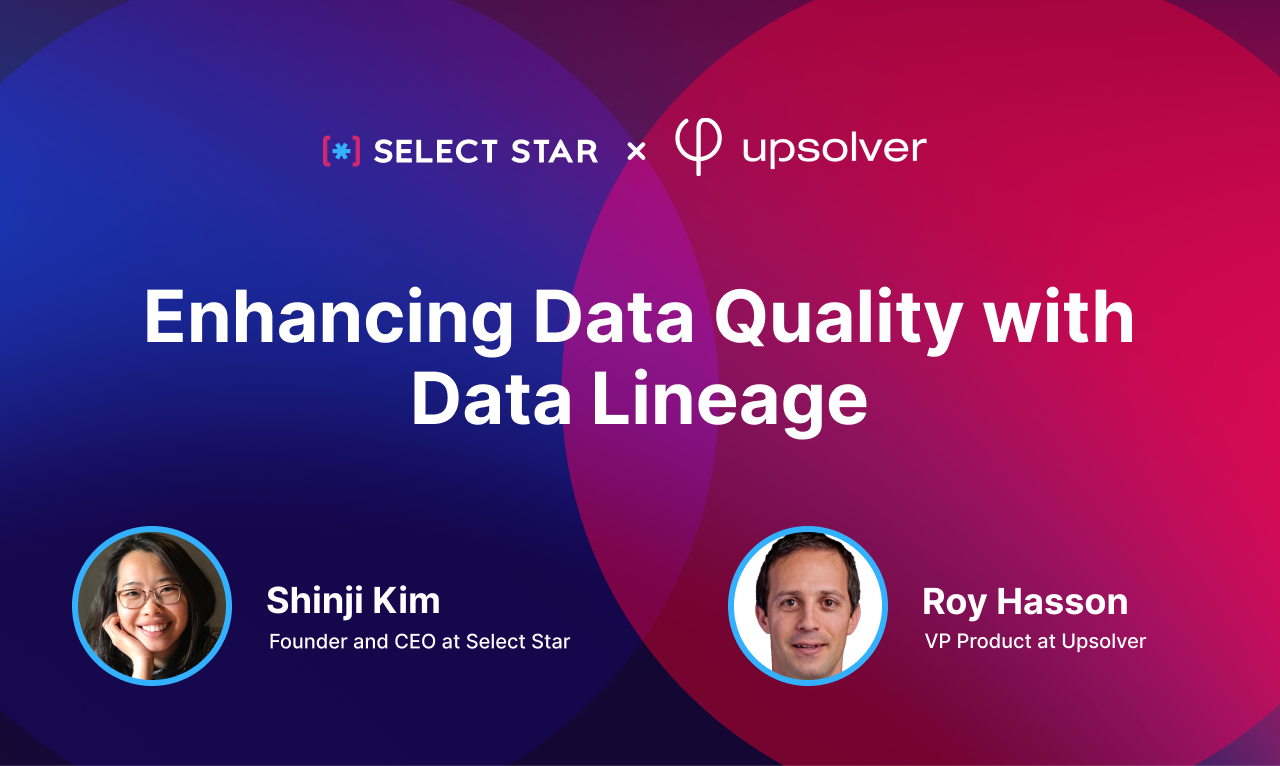
In data management, analysts, data engineers, and managers grapple with numerous challenges stemming from one central issue – data quality.
High-quality data is fresh, accurate, complete, reliable, and applicable to the business case it informs. Maintaining that quality is as important as it is daunting.
An organization’s day-to-day operations introduce many opportunities to damage data quality. Multiple collection channels produce duplicate data. ETL failures lead to incomplete fields. Inconsistent data formats skew transformations.
As data management becomes increasingly complex, the significance of high-quality data takes center stage.
In a recent live chat, Shinji Kim, founder and CEO of Select Star, and Roy Hasson, VP of product and marketing at Upsolver, discussed the transformative role of data lineage in enhancing data quality and governance.
The Link Between Data Lineage and Data Quality
Data lineage visualizes the journey of data flowing from its sources to various transformations and destinations.
“I would consider this a map that shares the data asset dependencies, kind of like its children,” Shinji explained. “It shows where and how the data was created. When you are able to understand all the sources of data, you can connect them into a graph or a tree-like view that shows all the dependency chains of every table and column."
Clear data lineage provides a comprehensive view of the data supply chain, allowing you to pinpoint where quality issues may have occurred.
Data Observability tools ensure data meets established criteria like correct row counts, unique columns, and adherence to reference data standards.
However, there’s more to data quality than technical correctness. Understanding where the data came from is important.
“Even if the data is all correct and sound and it's the latest and greatest data, if the data is labeled wrong or if the table does not have the right columns, then it's still not great data,” Shinji said.
She pointed out that even correct data is unreliable when it’s misapplied.
“I may end up using it without understanding that the data has been already filtered by certain conditions, or whether this data was actually about active users of my definition versus somebody else's definition,” she said.
Can Data Lineage Help in Data Quality Troubleshooting?
The lineage won’t tell you where things are broken, Shinji explained. But you can use lineage to troubleshoot within minutes instead of hours.
The average company collects data from 400 different sources. Unless they automate quality checks like validation, organizations must choose between the likelihood of low-quality data and the cost of dedicating engineering hours to manual checks and cleansing.
Automation is a good first step, but it can’t prevent quality issues entirely. Lineage is a powerful tool data teams can use to zero in on data quality issues and fix them as they occur.
The faster the issue is resolved, the less damage it causes downstream, and the sooner the correct data can inform critical business decisions.
Data lineage provides a visual guide that can be analyzed when anomalies such as a malfunctioning dashboard or problematic table arise.
Instead of digging into extensive lines of code in repositories, engineers can follow lineage’s concise map of the journey from upstream data sources to where the data looks broken.
This approach accelerates troubleshooting, offering insights into recent changes and facilitating the identification of the root cause of data quality issues.
While lineage may not solve the problem outright, it acts as an instrumental guide, helping data engineers and analysts define and understand when the problem arose and what activity may have caused it.
How Does Data Lineage Contribute to Security and Regulatory Compliance?
When it comes to regulatory compliance, looking into the downstream lineage allows automated tracking and auditing.
Shinji emphasized two significant aspects that emerge in the context of regulatory adherence:
- Classifying sensitive data
- Tracing how data has been utilized
“One of the more differentiating features of Select Star is understanding the column usage in lineage,” Shinji explained. “When we show the lineage in the column perspective, we will display whether this column or field has been utilized as is, whether there was a transformation like anonymization, or whether an aggregation of that data has happened.”
Tracing data back to its source facilitates respect for user privacy – ensuring, for example, a person whose data was collected for regulatory compliance doesn’t end up in a marketing sequence. It’s also invaluable in the event of a breach, making it possible to quickly find and respond to the sources of suspicious activity.
Most regulating agencies want to see consistent handling of data. Data lineage proves to auditors that your organization’s data management practices are compliant.
Exciting Times Ahead: Enriching Lineage with Metadata
According to Roy, the data landscape is entering an exciting new era.
“I think the lineage graph, the visualization, the early map is the V1 of lineage,” he said. “V2 is when you start enriching it with a lot of metadata.”
To illustrate the significance of both technical scalability and user experience, Shinji highlighted the experience of Select Star customer Block.
Block manages an extensive data environment with over 42 million lineage nodes and edges across 600,000 tables, she said. A scalable solution is crucial.
“The overall processing of lineage, like being able to actually keep it up to date... is one part,” Shinji explained. “The other part is user experience.”
To enhance the user experience, Select Star introduced features like a list view of lineage, enabling users to open or close branches selectively, and filter and search functionalities.
These additions allow users to quickly locate specific tables or data assets within the expansive lineage, streamlining the overall navigation and search process.
The goal is to efficiently summarize and present relevant information while ensuring a swift and responsive user experience.
See Data Lineage in Action with Select Star
As organizations strive for better data quality and governance, data lineage should not be underestimated. The power of lineage goes beyond technical troubleshooting, as it provides a comprehensive understanding and definition of data products within the organization.
Select Star's lineage not only addresses the technical challenges of lineage implementation, but enriches it with rich metadata to make it useful for data quality.
To learn more examples of how lineages are being used by data teams, check out this post. To see lineage in action, start a free trial or request a demo today.