
As organizations embrace data-driven decision-making, they often grapple with an explosion of data sources, dashboards, and reports. This was the reality for Tim Hughes, the BI architect at AlphaSense, who faced the challenge of navigating dashboard sprawl with AlphaSense’s 1,900+ dashboards. At the Tableau Conference this year, Tim shared how an upstream data change had the potential to lead to double-counting in downstream dashboards. He leveraged Select Star to pinpoint the dashboards that would be be affected by the upstream data change. The critical insights he derived from Select Star’s column-level lineage led the VP to delay the rollout until those impacts could be mitigated.
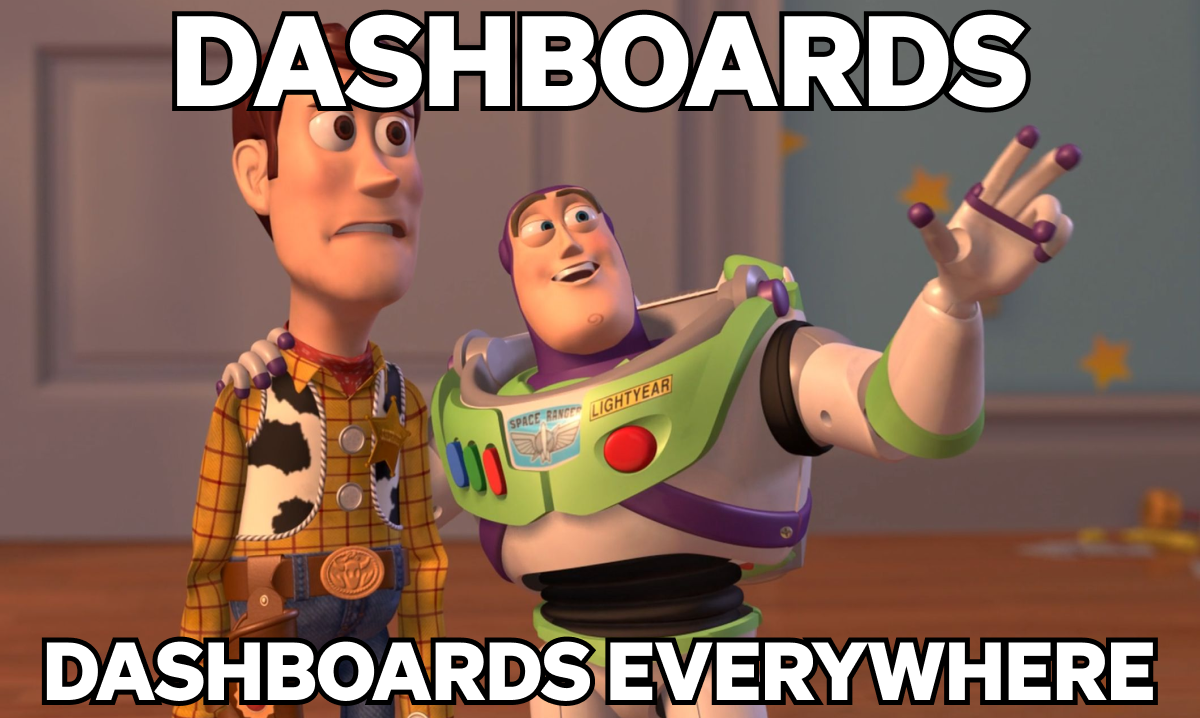
Data & Analytics at AlphaSense
AlphaSense leverages GenAI to ingest, synthesize, and summarize information, enabling professionals to make smarter business decisions with confidence and speed. AlphaSense’s legacy reporting tool couldn’t keep up with the amount of data the company ingests. Data flows into the business from 2,600 source objects using Stitch, Google Cloud Functions, and BigQuery.
Adverse Upstream Data Changes in the Context of Dashboard Sprawl
AlphaSense’s RevOps team had planned to make changes to how renewal dollars were tracked in Salesforce. While this was needed from a business perspective, if no other changes were made, this would lead to double-counting of dollars in an unknown number of AlphaSense’s almost 2,000 dashboards.
How Select Star helped AlphaSense identify dashboards impacted by the data change
Step 1: Document upstream changes
The first step to mitigating this impact was meticulously documenting the planned upstream changes. It was important to understand the purpose and scope of the changes so the team could fully grasp their potential impact on existing infrastructure and dashboards.
Step 2: Quantify the impact
It can be difficult and time-consuming to identify impacted dashboards out of thousands. Select Star streamlined this step, using column-level lineage to quickly and efficiently identify between 190 and 223 impacted dashboards.
.png)
Step 3: Sound the alarm
When Tim had enough data from Select Star, he notified stakeholders of the changes’ potential impact. Decision makers had enough evidence to conclude that additional time was needed to prepare for the changes.
.png)
Step 4: Co-design a solution with RevOps
AlphaSense's decision to delay the changes put them in a good spot to identify potential paths forward and co-create a solution.
Step 5: Manage the project to accommodate the changes
After reaching a solution, AlphaSense used Select Star as an API. This provided a precise impact analysis that identified 85 dashboards that would definitely be affected. Thirty of the impacted workbooks were archived. Of 27 published data sources, only two required changes to the upstream dbt models. The remaining changes could be accommodated in the dashboard layer.
.png)
What’s next for AlphaSense?
Navigating this adverse upstream change was just one step in AlphaSense’s data governance journey. AlphaSense has three focus areas to tackle next:
- Complete data transformation tool migration from Dataform to dbt using Select Star to manage downstream dependencies
- Monitor for unadvertised upstream breaking changes using Select Star’s schema change detection
- Reduce dashboard sprawl by developing an automated asset archival process using Select Star’s API
Key Strategies for Every Organization Struggling With Dashboard Sprawl
The success story at AlphaSense highlights the power of harnessing metadata to navigate the complexities of dashboard sprawl. Shinji Kim, Founder and CEO of Select Star, then expanded the discussion to outline several key strategies to effectively manage dashboard sprawl for every organization:
- Track dashboard usage: By monitoring the usage of dashboards, organizations can identify which assets are actively being viewed and which ones are potentially redundant or obsolete. See an example of usage with Select Star’s popularity feature.
- Deprecate or remodel: Information from dashboard usage can identify unused or less-used dashboards and can guide decisions around deprecation and consolidation. By streamlining and focusing on the most critical assets, organizations can improve efficiency and reduce the burden of maintenance.
- Leverage data lineage: Use lineage to check upstream datasets and their downstream impacts. Tools like Select Star provide granular lineage information, enabling teams to proactively manage and communicate the effects of modifications to the data pipeline.
For another perspective on managing dashboard sprawl and other data products, hear from Fivetran and how they navigate dashboard sprawls with data product management. By leveraging tools like Select Star, organizations can gain a comprehensive understanding of their data ecosystem, enabling them to make informed decisions, streamline processes, and maintain the integrity of their analytics pipeline. To learn more details about how AlphaSense harnessed metadata to manage dashboard sprawl, check out the full slides here.