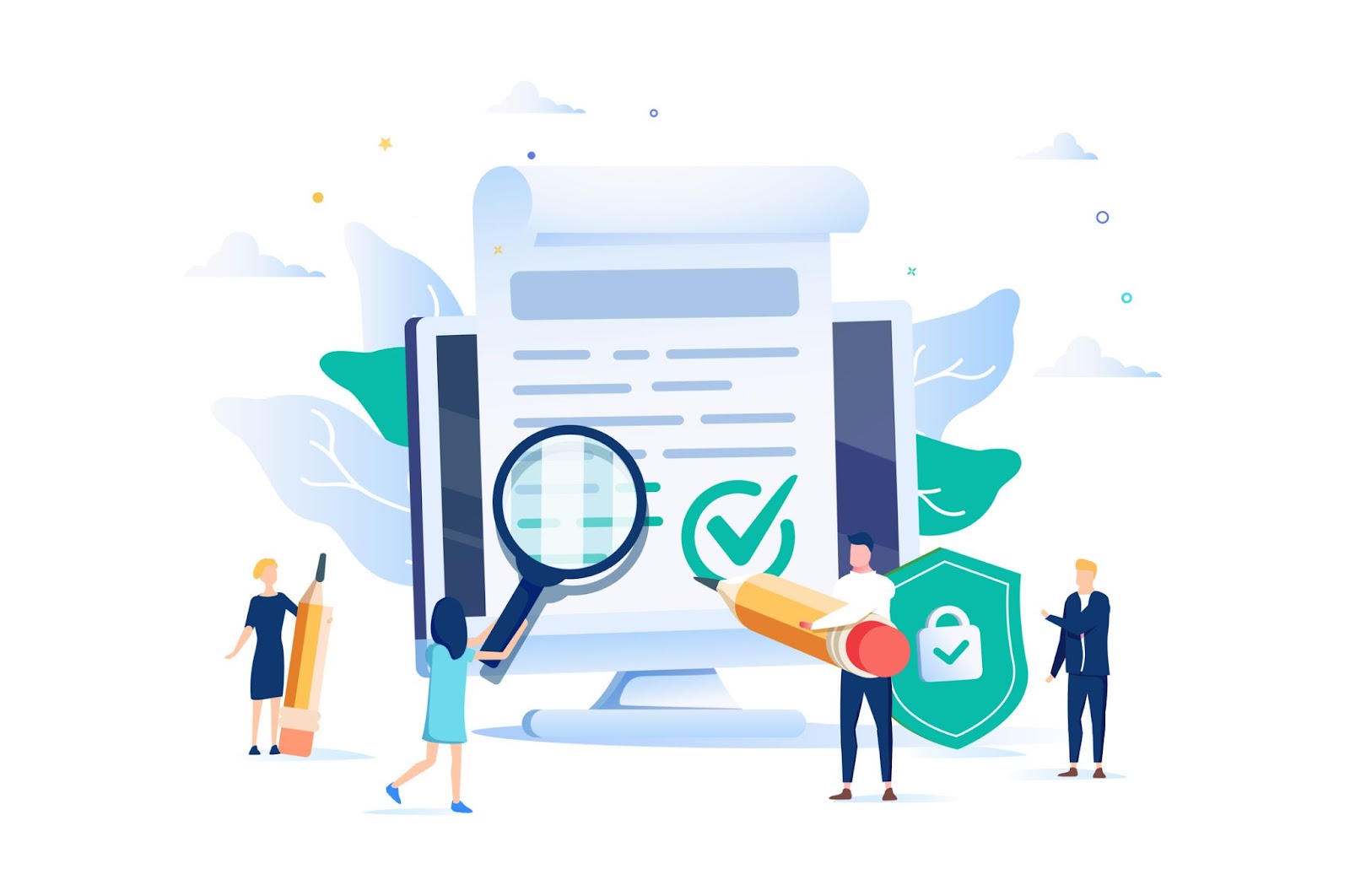
The emergence of new technologies in advanced analytics, machine learning, and the Internet of Things (IoT) has increased the demand for consistent data collection and use. However, poor data management practices that don’t ensure the quality, accessibility, and availability of reliable and secure data can make it difficult for organizations to manage this process and create meaning from their data reserves. This is where the concept of data governance comes into play.
Data governance is a set of practices that enables an organization to manage the quality, integrity, usability, accessibility, compliance, and security of the data it collects or generates. These practices ensure that stakeholders have access to high-quality data and that it is managed properly throughout its lifecycle. With strong data governance, organizations can better analyze their data to gain insights, control costs, mitigate risks, and comply with regulations mandated by governing bodies. A thoughtful data governance strategy is vital in an increasingly data-driven culture, as shown in a recent Gartner survey.
This article will explain the components of a data governance framework and how to implement such a framework for modern organizations.
What Do You Need from a Data Governance Framework?
To build a modern data governance framework, you first need to answer the question, "Why do you need a data governance framework?"
Data, being an important asset for any organization, plays a pivotal role in decision-making. Organizations need to make data-driven decisions to be profitable while complying with the security and regulatory requirements of various governing bodies. They must have trust in their data management practices to ensure that all of these requirements are met. Without a proper data governance framework, they will have difficulty achieving these goals while also maintaining value for the stakeholders.
Having a data governance framework ensures the following…
- Trustable Data: The company’s data is accurate and reliable for making informed decisions.
- Accessibility: Data is usable and easily accessible by all the stakeholders when required.
- Security: Data is protected against potential misuse and violation of privacy issues.
- Compliance: The organization maintains compliance with governmental regulatory requirements and has access to a history of data access and changes for audit.
For example, a music streaming company tracks and stores huge volumes of user data, like demographics, what music they listen to, and how long they listen. With a proper data governance policy and tagging of specific data sets, the company can quickly navigate to the most reliable data without the risk of accessing personal information. The product team can then use data to recommend songs to new users without compromising the privacy of users. This is a win-win situation for both the end-user, who receives personalized recommendations based on user behavior, and the company, which increases user engagement and helps boost customer loyalty.
What’s Included in a Data Governance Framework?
Once an organization has determined what it needs to improve its data governance practices, it can begin to design the framework. A good data governance framework includes the following categories:
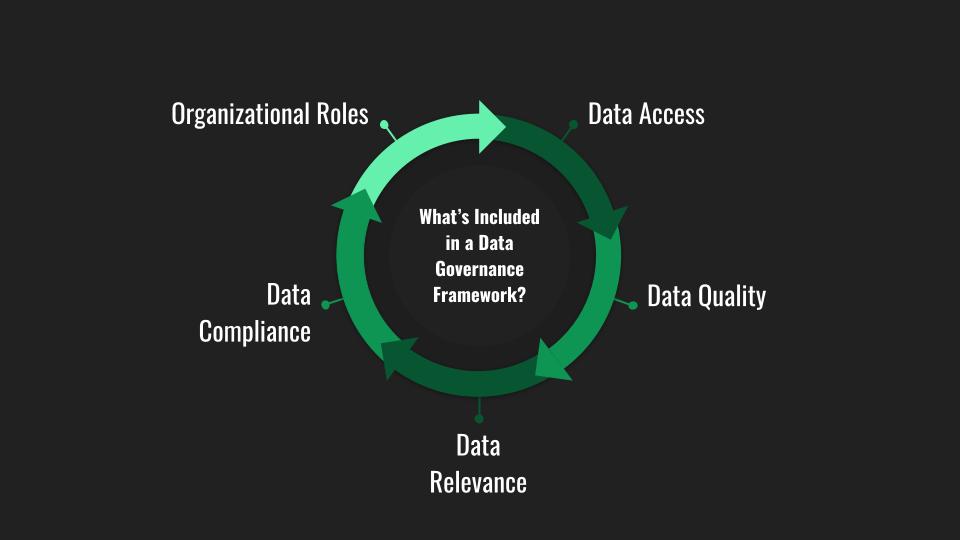
Data Quality
A 2019 McKinsey survey found that poor data quality and availability waste 30 percent of employees’ time. According to Gartner’s 2021 report, poor data quality costs organizations an average of $12.9 million yearly.
Organizations have departments such as marketing, finance, operations, and administration, and each has its own method of storing and crunching data. This can result in data silos that are inaccessible to other departments, making data sharing within an organization difficult. The organization may not know which department’s data is reliable enough for decision-making and insight. This leads to data inconsistencies and duplication.
Data quality is also affected by human errors in data entry, interpretation, or formatting. This is why also understanding data lineage is critical. To avoid a negative impact to downstream analytics, organizations must ensure that data is complete, error-free, and unbiased.
There are several ways to improve data quality:
- Tracking metadata: This basic yet important information (such as data type, date created, and source) significantly improves data usability.
- Data Profiling: Tagging and profiling data will help users better navigate to the right data sets. This may include monitoring data statistics such as average/sum or tracking anomalies.
- Column-level lineage: Data lineage should be maintained at the column level. Among other things, this helps team members determine the origin of the data so that they can more easily track errors.
- Clear documentation: A single source of truth implemented across an organization helps ensure data consistency.
A metadata management tool should facilitate all of these, and in turn, improve your data quality.
Once an organization’s data governance framework is implemented, it’s important to regularly monitor the success/challenges and create a feedback loop to improve the data governance strategy. There are many ways a monitoring process can be added. For example, setting alerts for when users access deprecated or erroneous data; tracking across the data chain on how data is modified and used; and compliance monitoring will identify areas where governance policies are failing.
Data Access
If trusted data is key to making informed and successful decisions, accessing it at the right time is crucial for taking action. Organizations must ensure their data lifecycle is frictionless and accessible by the parties authorized to make decisions. For example, in an ideal organizational setting, classifying data into different access levels helps determine who has access to what data and how long the data can be retained. It provides a better understanding of who is using data and what the data is being used for, which in turn allows organizations to track down data that is being misused or exposed to unauthorized individuals and ensures compliance.
Organizations should also have a systematic way to identify what data exists and describe the datasets (data discovery) and ultimately provide efficient processes for providing access to the data. This is important in preventing duplication of work and efforts.
There are many barriers to data accessibility in an organization. Employees might not have the right technical or systems knowledge, they might not know where the data is stored or which copy of the same data is the most reliable, or they might be subject to regulations restricting them from access. If an organization is committed to adopting a data-driven culture, it must enable its employees to acquire the necessary technical skills and provide them with secure data access.
Data Relevance
As the demand for data analytics grows, organizations must invest in the systems and people that help transform raw data into data that the analytics team can trust and utilize freely. There are often multiple steps involved in adding relevance to raw data, including data wrangling, ETL processes, and data munging. Gathering irrelevant data will make the data collection process time-consuming and costly leaving the team unable to make informed decisions through downstream business intelligence tools and dashboards.
Understanding an organization’s data model and use cases will aid in targeted data collection. Tools for data discovery such as a data catalog and data tagging can add further contextual relevance to data sets, ensuring that companies can operate their data collection in a lean manner while still obtaining all the relevant data needed for analysis. This is optimal when analytics teams work closely with their engineering and IT counterparts to better scope requirements.
Data Compliance
As greater amounts of user data are captured and shared, organizations become more vulnerable to online attacks or data breaches that compromise user privacy and security. For example, in March 2022, Shields Health Care Group suffered a data breach that affected approximately 2 million Americans. During the breach, sensitive customer data was compromised, including names, addresses, and Social Security numbers.
To reduce this risk, organizations should be vigilant that they adhere to data privacy, security, and compliance policies with local or national regulatory bodies. Such regulations include the Health Insurance Portability and Accountability Act (HIPAA) in the US or the General Data Protection Regulation (GDPR) in Europe. It is often challenging for companies to maintain their governance policies as they scale and start to ingest more data sources. More incoming data that is external to the organization and growing analytics teams often put stress on compliance practices. One potentially overlooked vulnerability is what the data analyst or data engineer can see or should see in their work.
Compliance monitoring is especially important because compliance can be difficult, due to ever-changing requirements around data security. Noncompliance is often not an option because of the risk of hefty fines and the potential impact on business reputation and productivity. Governments and policymakers are becoming stricter, and fines are increasing massively.
Data discovery platforms like Select Star help by providing services like column lineage tracking and PII tagging, which identify where your data is stored, who should have access to what fields, and how it's being used. These insights can help inform data governance practices. For example, Select Star often encourages organizations to look at usage analytics because popular usage can often tell the user what data sets are most used and admins can look to ensure that the right people have access to those data sets both upstream and downstream in the analytics tools. This also allows admins to easily report who has accessed the data and remediate if appropriate.
Organizational Roles
Managing the data governance initiative requires a team that oversees the day-to-day functioning and compliance of an organization’s data governance policies and instills and evangelizes a culture of data governance within the organization. The roles may vary by company, but here are some industry-defined roles inspired by the SAS data governance framework for managing an organization's data governance:
- Data stakeholders are the end users of the data, and the data governance framework must keep all internal and external stakeholders in the loop. For example, data scientists and business analysts are internal stakeholders, while customers and vendors are external stakeholders.
- Data stewards are responsible for collecting, classifying, and categorizing the data per the business rules and the policies set by the data governance committee. This ensures consistent and valid data throughout the organization so stakeholders can derive business value from it. They are also responsible for maintaining the metadata definitions, determining usage details, and reporting on quality metrics.
- Data owners are responsible for storing, engineering, and managing relevant data for stakeholders. They have the authority to make data-related decisions and often work with internal stakeholders. These roles are often filled by data engineering or other technical teams.
- Governance and compliance teams ensure compliance with all legal, privacy, security, and governmental regulations.
There is no one-size-fits-all solution for data governance. Smaller and more agile teams, for instance, may not have a defined role for data steward or a fully comprised data governance team. In these scenarios, the roles are often distributed between the data and analytics teams supplementing with heavy documentation to ensure that everyone is working on the same framework.
Steps for Building a Data Governance Framework
Once all the policies, frameworks, teams, and tools are defined, the next step is implementing the data governance framework. The implementation steps may look like the following:
- Define the teams and roles needed to address business goals, benchmark deliverables, and track key metrics from the organization’s data products.
- Ensure that data sources and processes are instrumented with data discovery solutions so that the governance framework can address the specific needs of the existing usage and access.
- Identify the most used/popular data sources and start to build your framework from the most used data assets.
- Tag and document datasets to more easily assign tagged data to teams and ownership levels.
- Tag and document PII data fields and mask exposure to the end users
- Maintain data quality by putting alerts and checks in place to catch erroneous data before it enters databases.
Conclusion
There are multiple factors and processes to consider when implementing a modern data governance framework, from data quality to security to management. However, building such a framework properly and informed by data discovery can help an organization make more accurate decisions and meet its business goals.
Implementing such a framework can be overwhelming, but there are tools to help you. Select Star provides a single source of truth for your data. It instantly identifies the most popular data sources and gives you deep insights into usage and access with column level lineage. Select Star provides collaborative documentation that helps users quickly identify the right data sets to use and informs the governance function on the intent of the data. It provides easily accessible metadata management across your organization so that all stakeholders and administrators can stay on top of how the data is being used.
Sign up for free to see what Select Star can do for your organization.