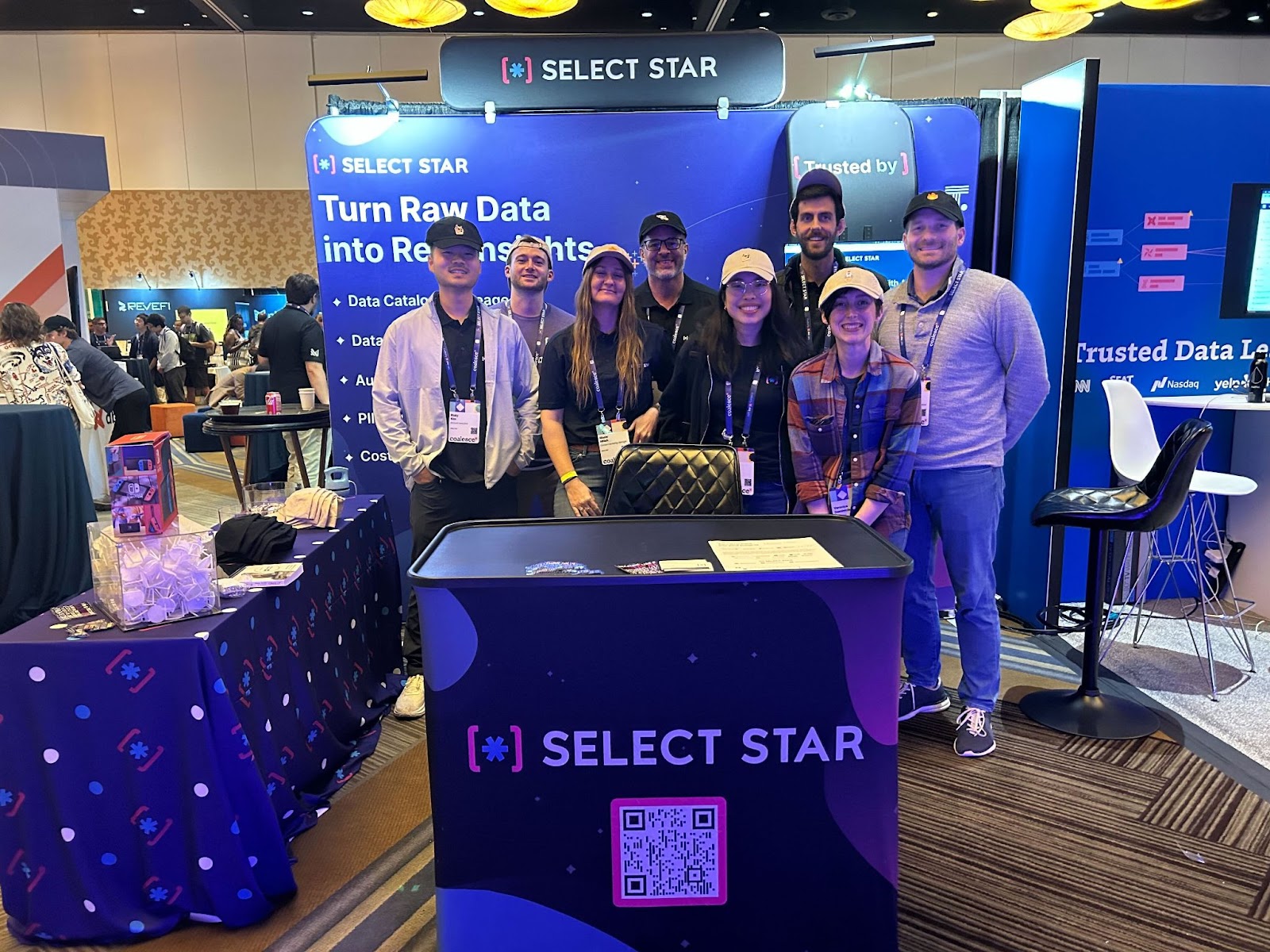
From October 16th to 19th, dbt’s Coalesce transformed the Hilton San Diego Bayfront into a hub for data enthusiasts. Drawing a global crowd keen on connecting and learning from data industry leaders, Coalesce became this year's go-to gathering for data professionals.
Central to the conference was the theme of data democratization—the push to make data more approachable and usable for all, technical or not. Here are the key takeaways from the sessions that drove the importance of democratization home.
Shift from isolated ecosystems to interconnected workflows
The first step toward data democratization is building an interconnected system where every tool and team is part of a cohesive whole.
Jisan Zaman’s session, Using data pipeline contract to prevent breakage in analytics reporting, explored this concept in practice. In this talk, Jisan explained how Xometry initially faced challenges due to the software development team using different tools than the data engineering team. This separation made it hard to trace issues and collaborate on unified solutions.

To get started, the Xometry team created something that they coined DPICT, which natively integrated with their existing application engineering stack components like Github, GitLab Pipelines, and Docker. Central to the DPICT architecture was the implementation of Select Star's column-level lineage feature, which provided clear visibility into how changes in upstream objects could affect downstream processes. This foresight allowed Xometry to proactively manage changes by setting up error messages that link directly to Confluence pages which detail the implications of each change.
Using their DPICT architecture, Xometry ensures that every team member, regardless of their specific function, has visibility and understanding of the broader data landscape. This integration led to 200+ hours saved annually for their data engineering team and a 36x boost in data debugging speed.
Lean into investing and automating early
The importance of interconnected tools and workflows was further emphasized in Fanduel’s session, How Fanduel migrated a mature data organization. Fanduel, an online sports betting arena and sportsbook, ingests massive amounts of data—processing 6 TB of data a day and storing nearly 500 TB of data.

The challenges quickly became clear as Fanduel began to scale:
- Mounting business pressure: If there were upstream issues, reports were delayed
- Incomplete insights: There were no data quality checks in place
- Loss in trust and reliability: Data customers discovered most data issues (duplicates, missing, or incorrect)
Fanduel was dealing with what they called “dependency spaghetti,” so they began refactoring their data ingestion pipelines to adopt an ELT paradigm and migrate all 15+ DAGs to dbt. They set out to increase data quality, improve development turnaround time, and reduce SLA failures.
They decided to start small to prevent any major outages, so they established a pilot project. In this pilot project, they upskilled a small team with dbt, focused on one product (in this case, sportsbook online), and established best practices and development procedures. Then, once all the kinks were worked out, they performed an organization-wide rollout.
The results spoke volumes. Their migration effort led to a whopping 992% improvement in data quality checks, a 1600% rise in data cataloging, and ensured a consistent 100% fulfillment of daily report SLAs over the past four months.
Go beyond a single source of truth
Modern data management is fundamentally rooted in the creation of cohesive, interconnected systems that are guided by a single source of truth. However, to achieve true data democratization, this “single source of truth” principle must be extended. It's not just about having a central repository of information; it's about fostering an environment where data is contextualized with clear lineage.
Appropriately, this was the topic of discussion during the lunch 'n' learn session, How data teams drive impact with data discovery and data lineage.

Select Star played a pivotal role in both Xometry’s and Fleetio’s implementations, emphasizing not just the 'where' but the 'how' and 'why' of data interactions. By tracking data from the source to the destination at the column level, Xometry’s and Fleetio’s data engineers could track their data back to the root cause of the issue and debug quicker than ever.
Because they had been through the implementation process from start to finish, Evan asked Jeff and Jisan some pointed questions, including:
- What aspects of lineage are the most important to evaluate?
- How did you make the business case for bringing on a tool to help with discovery and governance internally?
- What was the biggest challenge with implementing data discovery in your organization?
In general, Jeff and Jisan agreed that finding a low-maintenance column-level lineage tool that works down to the BI layer is the biggest step toward democratization. That’s why integrating Select Star into their workflows has been a game-changer for both Xometry and Fleetio—it provides them with comprehensive data lineage that tracks the history of their data from end to end. Transparency at this level ensures that all users, regardless of technical skill, can understand the implications of their data, empowering them to make informed decisions and contribute meaningfully to the collective data narrative.
Adapt processes to evolve as you scale
Jeff Chen, director of data & analytics at Fleetio, expanded on Fleetio’s data evolution in his session, Beyond Metrics Modernization at Fleetio.
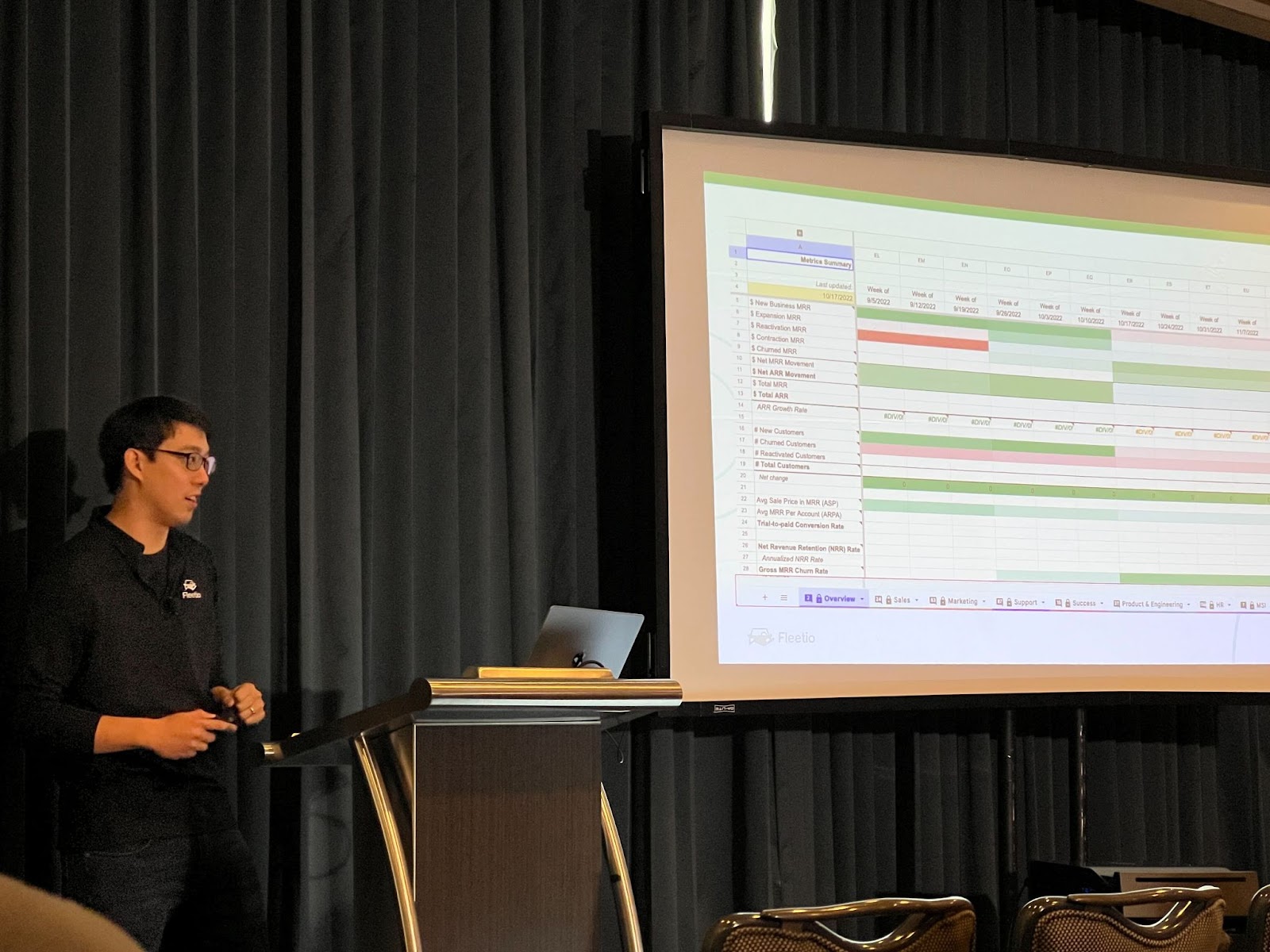
When Fleetio launched in 2012, their SaaS metrics (e.g. MRR and CAC) were tracked using spreadsheets. This method worked initially, but as Fleetio grew, the limits of spreadsheets became glaring—they even hit the maximum capacity of Google Sheets which prevented them from updating new metrics.
Faced with these growing pains, Fleetio evaluated their options. They needed something scalable, which narrowed their choice down to 3 options:
- Build models in dbt and dashboards in a BI tool
- Buy a BI tool that supports the semantic layer
- Build something on their own
After weighing their options, Fleetio built their own tool, leveraging Streamlit, which would allow them to write data applications in Python. Their custom solution involved defining metrics as raw SQL with separate configurations for filters and dimensions. This approach was inspired by the dbt metric specification and allowed Fleetio to pull definitions from dbt Cloud, working in tandem with their SQL generator and dbt’s Semantic Layer.
Fleetio's journey highlights a critical aspect of data democratization: evolving from simple, manual systems to automated, sophisticated ones that not only handle growth but make data insights more accessible and actionable across the organization.
The future of data is democratization
Regardless of an organization’s starting point, data discovery & governance platforms like Select Star empower everyone to engage with data meaningfully. After all, data shouldn’t be an exclusive domain of the few—it should be a shared, democratic asset that underpins every informed decision within an organization.
Want to hear more about how you can use Select Star to enable data democratization in your organization? Book a demo with one of our experts.