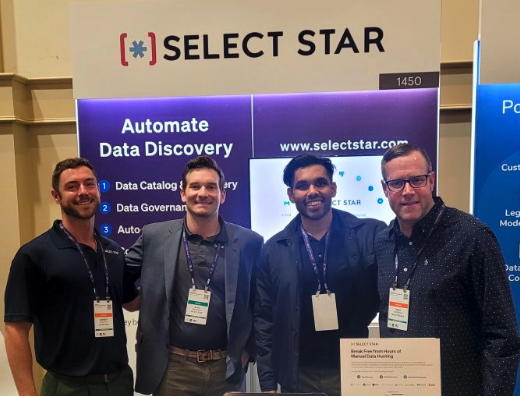
March marked a busy month for team Select Star. It was the beginning of three weeks on the road, talking to data people and showcasing our customers in speaking sessions. Our first stop was Orlando for the Gartner Data and Analytics conference. This was the first year the Select Star team would sponsor the event and we were excited to connect with data leaders in person.
Throughout the three-day event, we spoke to hundreds of data professionals seeking modern data discovery and data governance solutions. We also hosted a theater session with Pitney Bowes on how Select Star is helping them enable a data mesh architecture. While conversations varied throughout the three days, we noticed 3 major themes pervasive in all our conversations.
Data Mesh or Data Fabric?
One topic that seemed to own precedence in the clamor was the distinctions and implementations of data mesh and data fabric architectures. While education at the Gartner Summit was comprehensive on these topics, to many who deal with data daily, the concepts and differences are still largely an open conversation with no concrete definitions.
So what are data fabric and data mesh? Gartner defines the data fabric as “an architecture and set of best practices for unifying and governing multiple data management disciplines, including data integration, quality, active metadata, master data, pipelines, catalogs, orchestration, analytics, DataOps, and much more.” In contrast, a data mesh is defined as “a next-generation data management architecture based on domain-driven, distributed data management.”
Gartner Analyst Ehtisham Zaidi aimed to clear up the confusion on Tuesday morning with his session “Data Fabric or Data Mesh: Debate on Deciding Your Future Data Management Architecture”. For a data mesh, the resulting data products align well with the business domain that the data and analytics team reports to, as compared to data products that come from a distant, central team and its data. A data fabric architecture is well-equipped to address a broad set of data formats, processing paradigms, and deliver data reliably. Whether that is to a domain or a centralized data team.
Pitney Bowes' Data Mesh Journey
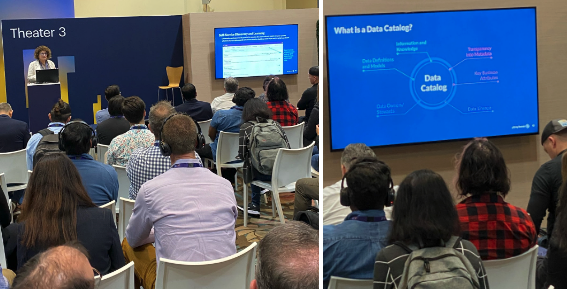
One of the most inspiring examples of enterprise-wide adoption of a data mesh architecture is Select Star customer Irina Ashurova from Pitney Bowes. In her talk titled “Building an Enterprise Data Mesh for Self-Service Data Discovery”, she outlined the considerations for building an enterprise data mesh including how to best support the domains with repeatable best practices. She detailed the toolkit her teams use to execute on the key deliverables of a data mesh. She will admit that she is still on a journey to the ideal framework and chronicled the future aspirations of the Pitney Bowes data team. For more about how Select Star and Pitney Bowes work together, check out this video.
Migration to Cloud Data Platforms
While cloud-native data solutions are increasing in popularity, many companies are still on their own journey of moving their data workloads to the cloud. Some companies may have a cloud data platform like Snowflake, Databricks, Azure, or Google BigQuery for certain initiatives. Still, it’s often a much harder reality to migrate the company’s center of gravity, especially if your company’s data practice has been around for more than 10+ years. While many of the attendees we spoke to were aware of the benefits associated with migrating to cloud platforms such as reduction in cost, dynamic scaling, self-service and automation abilities, etc., many simply were looking for where to start.
Gartner offered guidance on this topic in a breakout with Richard Watson titled “How to Apply Gartner's 5Rs Framework for Cloud Migration Decision Making”. Richard’s 5 R’s refer to “Rehost, Refactor, Revise, Rebuild, or Replace” and point to key decision points in how people should not only approach their cloud migration but also how they should look at implementing new cloud data platforms. As companies move to the cloud, it’s often easy to lose control over the purview of your data assets. As data is replicated to multiple cloud environments, duplication can often happen causing confusion for data teams. Implementing a data discovery solution that leverages automation and metadata can ensure you aren’t simply guessing when operating in multi-cloud environments.
Data Governance in the Cloud
Another key topic of conversation was how to design data governance for modern cloud-based systems. The previous strategies in play in many peoples’ environments rely on a centralized and tightly controlled governance model that was best fit when data was only stored in a handful of places and primarily structured. Data teams now realize that the tools and practices that were used for many years to perform data governance no longer fit the requirements for dynamic cloud environments. So many are back to the drawing board, aiming to provide an enforceable governance model for data that is largely under the control of end-users (vs. central IT). These solutions require careful consideration as they involve not only technology but also process improvements.
Let’s take for example a data mesh architecture, in this model, data needs to be governed in the center to ensure that each domain receives reliable and relevant data. From there, the domains will each continue to enrich and change the data. In this example, is the domain now the end of the governance chain? Should each domain then submit those changes back to a central governance authority?
In Gartner analysts session “Ask The Expert: Everything You Wanted to Know About the Data Fabric But Were Afraid to Ask” Ehtisham Zaidi points to his research that “The data mesh does not provide guidance around optimal utilization and reuse of existing data management infrastructure assets (components of the logical data warehouse) that most organizations have spent decades creating and managing. Rip-and-replace to a completely decentralized domain-oriented design is enticing but, in most cases, not practical.”
These are areas of debate currently in the world of professional data workers. As Irina mentioned in her talk, data discovery becomes a critical component of data governance. It guides data leaders to the most accessed and queried data assets to ensure they roll out data governance strategies on the most impactful data. Another key concept is data ownership, which helps define who is responsible for maintaining data at the individual asset or domain level. Documenting data ownership helps remediate potential missteps in rolling out data governance.
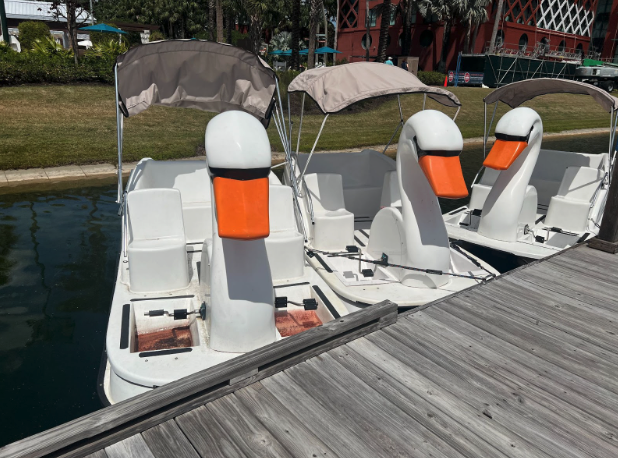
On the Road Again…
We would be remiss if we didn't thank all who ventured over to our small but humble booth space to learn more about what Select Star does. If you stopped by we would love to continue the conversation with you. Or, if you couldn’t attend this year, we would still love to connect and chat. You can book some time with our data discovery experts to learn more about how we are helping enterprises deploy data governance and the key pillars of a data mesh.