In today's data-driven world, organizations are grappling with an ever-increasing volume and complexity of data. As data flows through various systems and transformations, understanding its journey becomes crucial for ensuring data quality, compliance, and informed decision-making. This is where data lineage comes into play.
Data lineage is the process of tracking data from its origin to its destination, including all the transformations and movement it undergoes along the way. It provides a comprehensive view of data's lifecycle, enabling data professionals to trace the path of data elements, understand their relationships, and identify potential issues or inconsistencies.
Implementing robust data lineage is no longer a luxury—it's a necessity. As organizations strive to become more data-driven, the ability to trust and understand data becomes paramount. Automated data lineage serves as the foundation for this trust, offering clarity in an increasingly complex data landscape.
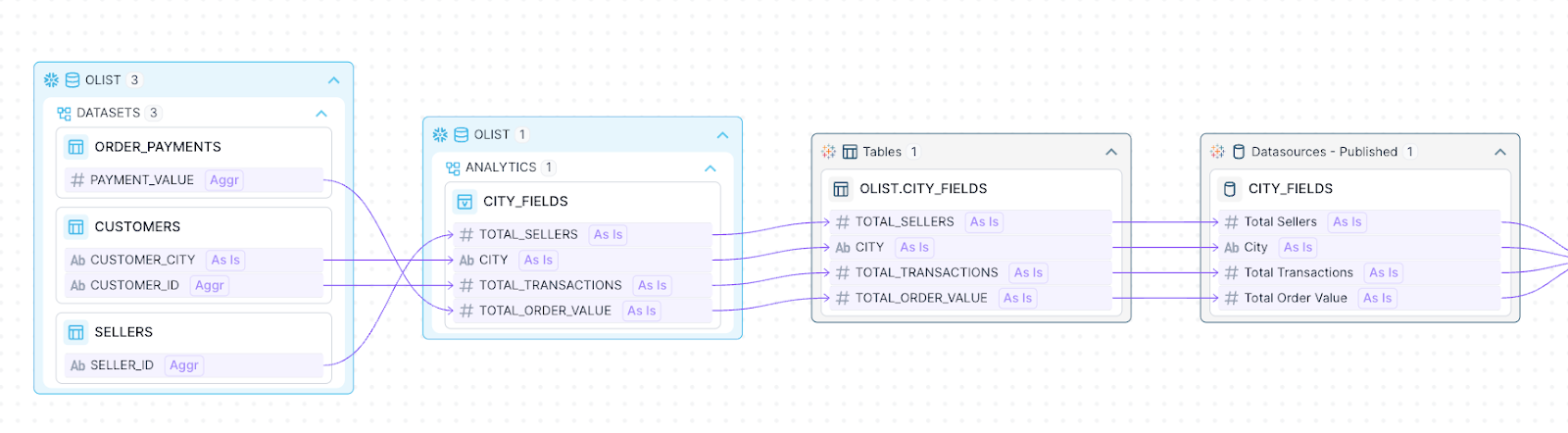
The Need for Data Lineage Tools
As data ecosystems grow in complexity, manual tracking of data lineage becomes virtually impossible. Modern data environments often involve multiple data sources, various transformation steps, and numerous downstream applications. This complexity introduces several challenges affecting data quality and reliability.
- Root cause analysis: Without visibility into data flows provided by lineage, root cause analysis can be painstakingly manual search to identify the upstream error that caused the issue. This prolongs the incident resolution times and potential disruptions to business operations.
- Impact analysis: Without comprehensive lineage information, making changes to data systems can lead to unintended consequences. These consequences can include unexpected breakages and downtime.
- Data governance: Effective data governance requires a clear understanding of data ownership, usage, and transformation. Data lineage provides the necessary context for implementing robust governance practices.
To address these challenges, organizations are turning to tools to implement robust, automated data lineage. Discover the benefits and the details of how data lineage works in our Complete Guide to Data Lineage.
5 Key Features of Modern Data Lineage Tools
When considering data lineage tools for your organization, there are several key features to look for:
- Automation: Manual lineage tracking is no longer feasible due to being time-consuming and prone to errors. Modern data lineage tools leverage automation to continuously capture and update lineage information, ensuring accuracy and reducing the burden on data teams.
- Column-level lineage: While table-level lineage provides a high-level view of data flows, column-level lineage offers the granularity needed to truly understand data transformations. It allows data professionals to trace individual data elements as they move through various systems and processes.
- Advanced search capabilities: With the vast amount of data and metadata in modern organizations, powerful search functionality is crucial. Users should be able to quickly find relevant datasets, transformations, and lineage information. Robust search functionality allows users to navigate complex data landscapes efficiently.
- Context enrichment: The best data lineage tools go beyond simple tracking of lineage and enrich the lineage with context and usage metrics. This enrichment allows users to quickly understand the data and key detail, including how it is being used and transformed.
- Coverage: From data warehouses to BI tools, your existing data stack should be supported by the lineage tool. Your tool should also support a wide range of data asset types, tables, dashboards, and more. This reduces the risk of any blind spots where issues may lurk and positions lineage to become a pillar of your data management strategy.
Top Data Lineage Tools
Organizations seeking to implement data lineage have a range of options available, from open-source solutions to comprehensive proprietary platforms. These tools cater to different needs and budgets, offering varying levels of functionality and support. Open-source options provide a cost-effective entry point for companies looking to explore data lineage without significant upfront investment. They often benefit from active community development and customization possibilities.
Several open source options offer robust lineage capabilities including:
- OpenMetadata
- DataHub
- Apache Atlas
- Marquez (OpenLineage)
While open source data lineage tools offer a solid foundation, many organizations find they need more robust solutions to address the full complexity of their data ecosystems. Proprietary tools often provide enhanced features, dedicated support, and seamless integrations that are critical for enterprise-scale deployments. These solutions can offer advanced automation, deeper analytics, and customized workflows that may be lacking in open source alternatives. For organizations dealing with sensitive data, complex regulatory requirements, or large-scale data operations, the following proprietary solutions provide comprehensive capabilities to meet these demanding needs.
3 Best Practices to Consider When Implementing Data Lineage
Implementing data lineage successfully within your organization requires careful planning and execution. It's not just about selecting the right tools, but also about fostering a culture that values data transparency and governance. To achieve this, you'll need to align your data lineage strategy with broader business objectives, secure buy-in from key stakeholders across departments, and develop a phased approach that allows for iterative improvements. The three best practices below will help you lay the foundation for a robust data lineage framework that enhances decision-making and data trust throughout your organization.
- Start small and scale up: Begin with a pilot project focused on a specific data domain or use case. This allows you to demonstrate value quickly and refine your approach before scaling to the entire organization.
- Involve stakeholders across the organization: Data lineage impacts various teams, from data engineering to compliance. Ensure all relevant stakeholders are involved in the planning and implementation process.
- Continuous monitoring and improvement: Data lineage is not a one-time project. Implement processes for ongoing monitoring, maintenance, and improvement of your lineage capabilities.
Future Trends in Data Lineage
Automated data lineage has become a critical component of modern data governance strategies. As organizations grapple with increasingly complex data ecosystems, the ability to trace data flows and understand data transformations is no longer a luxury—it's a necessity.
By implementing robust data lineage practices and leveraging advanced tools, organizations can improve data quality, enhance governance, accelerate incident resolution, and ultimately extract more value from their data assets. As we look to the future, data lineage will continue to evolve, becoming more automated, intelligent, and integral to data-driven decision making.
- AI and machine learning integration: AI and ML technologies are being increasingly applied to data lineage, enhancing automation, anomaly detection, and insight generation.
- Increased focus on data collaboration: Future lineage tools will place greater emphasis on collaborative features, enabling teams to work together more effectively in understanding and managing data flows.
- Enhanced automation in lineage tracking: As data volumes and complexity grow, automation will become even more critical. Future tools will offer more sophisticated automated lineage capture and analysis capabilities.
For data professionals navigating this landscape, staying informed about the latest data lineage tools and best practices is crucial. By embracing these technologies and approaches, you can help your organization build a more transparent, trustworthy, and valuable data ecosystem.