Keeping up with a rapidly changing business environment creates complex webs of connected data. Without proactive change management, a company can quickly find itself overwhelmed.
Powering change management with data lineage transforms those tangled webs into clear, orderly maps. Lineage enables efficient, reliable data observability, so that businesses can keep up with change as it happens – and head off problems before they occur.
In our recent webinar, Shinji Kim, founder and CEO at Select Star, and Mei Tao, product at Monte Carlo, talked about how data lineage can save a company millions of dollars – and give data teams peace of mind.
Why You Should Care About Data Observability and Lineage
Data observability, as Monte Carlo defines it, provides full visibility into the health of your data and data systems. It makes you the first to know when data is wrong, what broke, and how to fix it.
This allows you to identify anomalies, errors, and inconsistencies in your data as early as possible, rather than hearing about problems for the first time when downstream users report disruptions.
Core Pillars of Data Observability
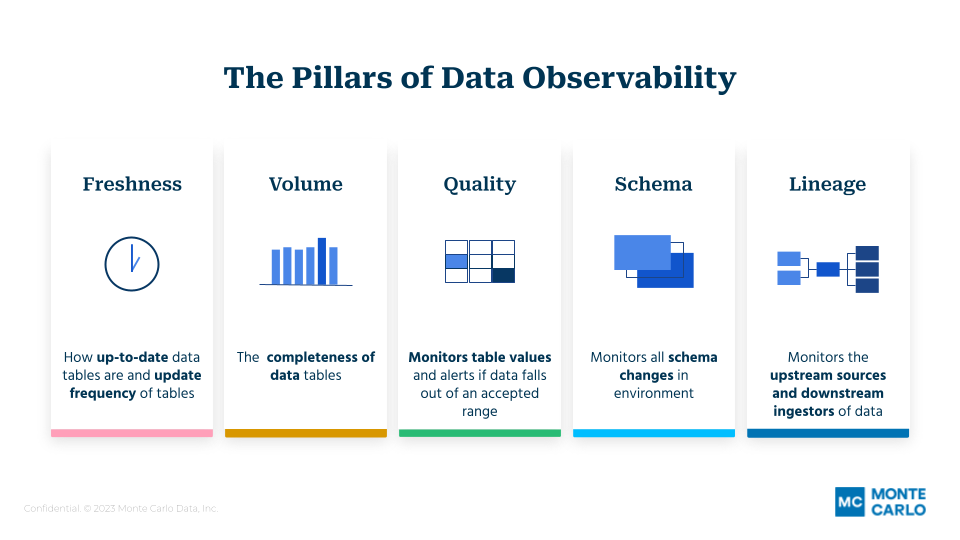
The five pillars of data observability are lineage, freshness, volume, schema, and distribution. When it comes to powering observability tools, Mei described lineage as "the map that helps you navigate your data systems."
Data lineage provides insights into the origin, flow, and transformations of data throughout its lifecycle. By using lineage to proactively detect issues and resolve discrepancies, data teams can prevent data downtime.
This is any period in which the information moving through the data infrastructure is incomplete, inaccurate, or potentially disruptive to business operations. Common causes of data downtime include metadata changes such as dropping a table or altering a column type.
How Lineage Helps Eliminate Data Downtime
Mei identified three phases of eliminating data downtime: detection, resolution, and prevention.
1. Detection
Advanced monitoring tools scan the data infrastructure and identify anomalies such as updates, volume fluctuations, or schema changes that could potentially disrupt operations.
In this stage, lineage maps dependencies and identifies potential upstream issues within the data infrastructure.
Aside from enabling proactive detection and timely resolution, lineage aids in calculating the Importance Score, or the number of downstream dependencies, allowing your organization to prioritize issues based on their potential impact on business operations.
"[Lineage] allows you to define what you really care about," Mei said.
2. Resolution
By tracing data lineage, data engineers and analysts gain valuable insights into the dependencies and transformations within their infrastructure, enabling them to pinpoint and address issues effectively.
Lineage plays two roles in the resolution stage:
- Prioritizing issues (Impact analysis)
Leveraging lineage data, data engineers and analysts can assess the potential impact of each issue on business operations, allowing them to allocate resources efficiently and address critical issues promptly.
By understanding the dependencies and relationships between different data components, your team can identify which issues require immediate attention and which can be addressed later.
- Resolving issues (Root cause analysis)
Once issues have been prioritized, the next step is to resolve them through root cause analysis. Lineage enables organizations to trace the root causes of data discrepancies and identify underlying issues.
3. Prevention
Lineage identifies the downstream impacts of proposed changes before they occur. It helps your organization understand how changes to data structures, schemas, or processes may affect downstream systems and applications.
This allows your team to anticipate potential issues, mitigate risks, and prevent data downtime.
Shinji said Select Star clients often comment on the peace of mind the tool gives them. They’re able to sleep soundly through the night without worrying their team will call them about a data emergency.
By distributing responsibility for data management across the organization, lineage allows data team’s collaboration and accountability, ensuring that all stakeholders are actively involved in maintaining the reliability and integrity of the data infrastructure.
How decreasing data downtime increases ROI
Shinji and Mei discussed three ways a tool that reduces data downtime impacts your ROI:
1. Making productive use of engineers’ time
Automated tools with good user experience supercharge productivity.
Reduced downtime means engineers can spend less time fixing the data infrastructure and more time optimizing it. A lineage tool that streamlines root cause analysis shortens troubleshooting from hours to minutes.
Automated tools also streamline compliance reporting. Real-time insights into data integrity and reliability make reporting less of a chore.
Finally, when a good tool works out of the box, its cost of ownership is dramatically reduced by calculating the number of engineering hours it would have taken to build and maintain the function in-house.
With fewer interruptions and less time on manual tasks, engineers can spend precious hours on revenue-generating activities like feature development and process optimization.
2. Rooting out wasted spend
Connectors serve as bridges between different data sources, systems, and applications, facilitating the flow of data across the organization's ecosystem. Businesses often don’t realize they’re paying to maintain obsolete bridges hidden away in forgotten corners of the infrastructure.
With visibility into the entire data flow pipeline, from data sources to end users, teams can analyze the usage and impact of each connector. Redundant and underutilized bridges can be identified and safely removed.
Organizations can then focus their resources on maintaining and optimizing essential connectors, ensuring smooth and efficient data flow while mitigating risks.
3. Protecting the bottom line
In industries where there is a direct correlation between downtime and bottom-line losses, even minor downtime can result in missed opportunities and lost revenue.
Organizations leveraging a data-driven pricing model could lose millions in downtime due to inaccuracies in the model.
A flaw in advertising data, for example, may lead to misallocated resources, ineffective ad placements, or missed revenue opportunities. The longer it takes to identify and rectify inaccuracies, the greater the financial repercussions.
Select Star customer Xometry developed an AI-driven algorithm for pricing manufacturing runs but struggled with data outages and accuracy issues.
Select Star’s automated column-level lineage capabilities let them easily identify data sources, detect issues in real time, and prevent data outages.
With improved data quality and trust, Select Star saved Xometry over 200 hours a year in troubleshooting and millions of dollars that would otherwise have been lost to inaccuracies.
What Makes a Good Lineage Tool?
There are two factors to take into account before building or buying a lineage tool:
1. It's easy to use
“Easy” is subjective, but there are a few objective ways you can measure whether a tool will be easy to use.
- It should automatically analyze SQL code and create the downstream and upstream dependencies.
- It needs seamless one-click integration with BI tools like Tableau, Looker, and Mode, along with customizable integrations.
- It should have a user-friendly interface (UI) that makes overwhelming data sets easy to access and digest. It should also have fine-grained access control to make sure that team members see what they’re supposed to see.
- It should allow users to propagate tags like a Personal Identifiable Information (PII) classification of the column. This feature enables you to distinguish whether the data value has been replicated or transformed.
2. It offers column-level lineage
Column-level lineage provides a much higher level of precision compared to table-level lineage, making it 10 times more useful in understanding data dependencies and transformations.
Column-level lineage provides significantly more detailed information about the flow of data within a system. If you're going through a compliance audit, identifying PII data across a data stack is impossible to do with a table-level lineage.
At the column level, you can trace the journey of PII data across processes and systems, enabling more precise identification and mitigation of compliance risks.
While table-level lineage may suffice for initial model design, column-level becomes indispensable when addressing issues, particularly in compliance and change management.
The Role of Lineage in the AI Future
Lineage is a critical tool for ensuring transparency, accountability, and reliability. As such, it complements generative artificial intelligence (GenAI), Mei said.
"At the end of the day, GenAI product is a data product — it has all the upstream data sources that it depends on, and it's serving data consumers," she explained. "Lineage helps GenAI products in the same way that it helps any data product. It reflects the flow of data within your GenAI stack, assisting in root cause analysis of issues.”
As GenAI gets more attention, the need for lineage will become even more crucial, Mei predicted.
With increasing concerns about issues such as bias, fairness, and transparency in AI-generated content, there is a growing need for tools to ensure accountability and traceability.
"As companies adopt and integrate GenAI, it's going to be a lot more important to ensure that the quality of output and the product that GenAI creates is monitored,” Shinji agreed. “A lot of that will be backed by lineage.”
As the capabilities and applications of generative artificial intelligence continue to expand, so does the scrutiny surrounding its use.
In this context, lineage is a critical component in the AI future, providing a mechanism for monitoring and auditing intelligent systems.
You Can Master Change Management with Data Lineage
Organizations can minimize data downtime, enhance data reliability, and improve overall operational efficiency as they manage business transformations.
Data lineage is a powerful tool for change management. And its ability to enable transparency and accountability will make it a key element in shaping the way businesses integrate AI into their data stack.
To discover how your organization can benefit from the power of data lineage, book a demo.