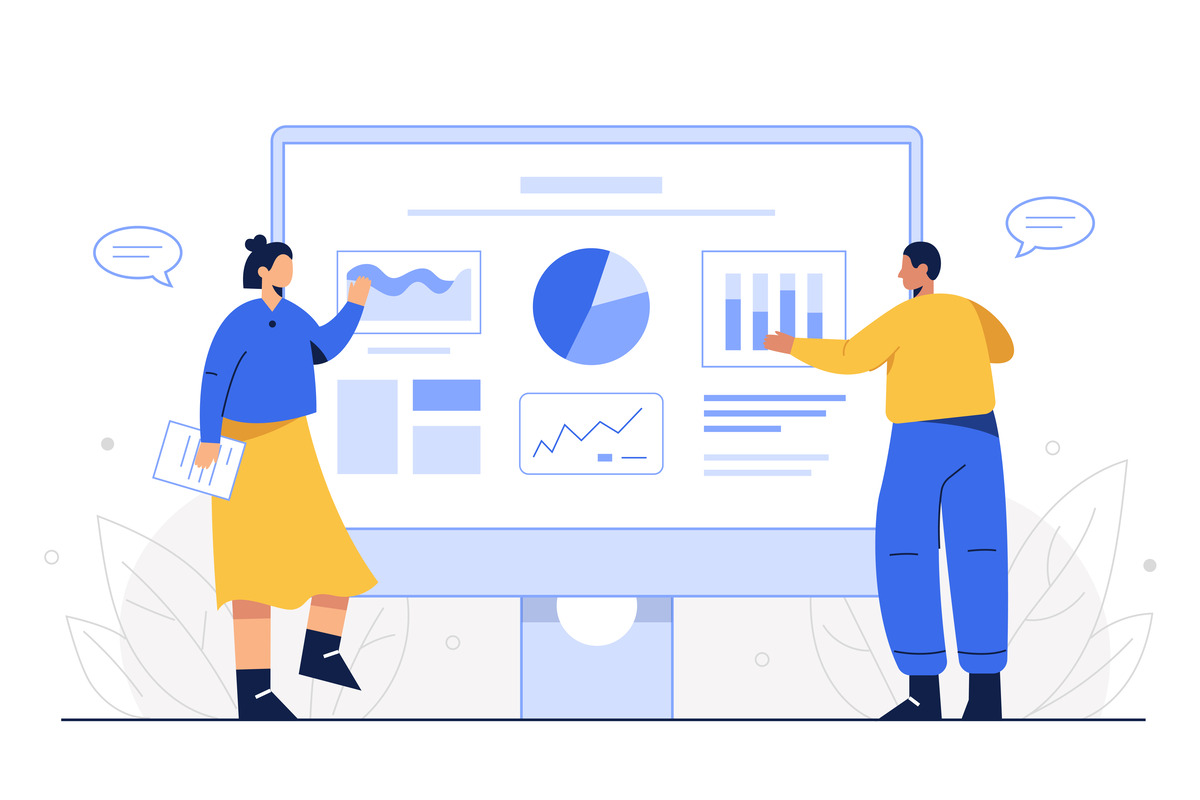
Data discovery and data observability are two recent trends in the data industry that have notable differences and some helpful overlaps. We often get questions from users evaluating a data discovery tool on how Select Star differs from data observability solutions and which one they should consider implementing first. I recently joined the team at Bigeye’s Data Reliability Conference (DRE Con) to deliver this session on how data leaders and managers should consider them.
Background
Data cataloging and governance have existed in the enterprise for some time, but you might be hearing increasingly more about the next-generation metadata management and data quality tools. So why is data discovery and data observability important now?
Many companies today are dealing with an explosion of data. Data is not just being used solely by engineering teams but by the whole business. The core data teams that deliver this data have too many data sets to manage. A common example is looking at user journey and interaction with the company in a 360 degree view. This type of analysis will require looking at data from internal tracking systems, marketing tools, and customer support platforms at minimum. Companies that want to create a 360 view of their customers will require connecting all the various data sources, which in turn, complicates data management.
While the amount of data and the different types of data sources are exploding, within today’s organizations data analysis is being decentralized. Each business domain has its own analysts and KPIs defined. This often creates siloed information and different definitions of how divisions measure their metrics.
Furthermore, by companies democratizing their data internally, various stakeholders within different business domains are starting to get data access via BI (Business Intelligence) tools. Now, a broad set of users are accessing company data with a different background, different context, and different understanding of the business.

According to Gartner, data discovery and observability are part of this larger DataOps discipline. Gartner defines DataOps as “a collaborative data management practice focused on improving the communication, integration and automation of data flows between data managers and data consumers across an organization.” The goal is to make data creation and processing more integrated and iterative through the full lifecycle. The practice of DataOps is also in service of reducing the friction of using data across the business so it can be helpful to everyone. Hence, data discovery and data observability are both key components of DataOps discipline.
Data Discovery vs. Data Observability
Data Discovery
The purpose of data discovery is to provide a single source of truth that includes the context and shared knowledge for everyone in the organization. By making the data more discoverable, it helps people find, understand and use organizational data more effectively. Some common features of data discovery platforms include the following:
- Data Catalog & Search - Searching through data objects and reports across multiple data sources.
- Data Documentation - Documentation about data assets in a form of data dictionary, wiki, business glossary, etc. Additional metadata can also be added such as tags and owners.
- Data Usage - Identification of how each dataset is being used, what its relative popularity is compared to other datasets.
- Data Lineage - Visibility into dependencies in the data models and ETL pipelines.
- Integrations with data warehouses & BI tools - Automated tracking of new and updated data assets.
Through data discovery, data engineers and data analysts can self-service their data questions rather than relying on tribal knowledge. Business stakeholders can also utilize data discovery to gain visibility into the data models and analysis being done. Overall, data discovery promotes data literacy and self-service analytics throughout the business.
Data Observability
The purpose of data observability is to provide operational visibility and analysis for data engineering teams to ensure that the data quality is sound and working correctly. Data Observability tools often have the following features:
- Metadata Monitoring & Alerting - Monitor data health metrics like data freshness, volume and schema. Users will set up alerts when updates have failed.
- Data Tests - Rules and checks are applied to ensure that the data adds up according to the business semantics.
- Data Profiling - A snapshot of data tables to understand how data values are changing. This is helpful when you are trying to track down anomalies in your dashboards.
- Data Lineage - Visibility into dependencies in the data models and ETL pipelines.
- Integrations with data warehouses, BI & data pipeline tools - Combined with lineage, root cause analysis and impact measurement can be done when data quality fails.
Utilizing Data Discovery & Data Observability for Better DataOps
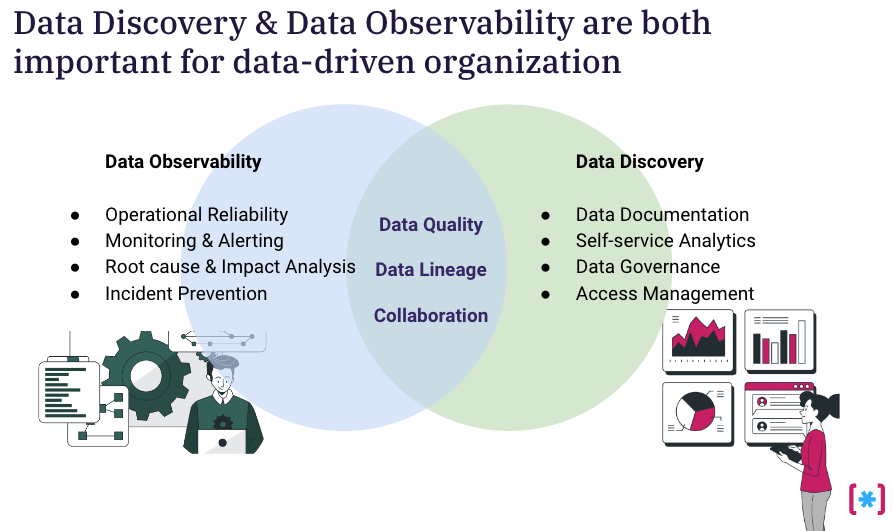
Data discovery and data observability are both important for data-driven organizations. While data observability is deployed to ensure the operational reliability of the data, data discovery is primarily adopted to house data documentation and knowledge sharing in one place. You can’t have only one and not the other as your data volume and variety of data grow. Yet, there are some overlaps in the features to think about: data quality, data lineage, and collaboration.
Data Quality
Data quality is usually measured in terms of the following dimensions: completeness, consistency, uniqueness, timeliness, and validity
Many people think data observability equals data quality. But to be precise, data observability is measurement and monitoring of the physical / technical dataset statuses and changes. When considering data quality, one should also ensure logical data integrity and data validity are in place in order to ensure data is being processed and used correctly.
So for data discovery, data quality means being able to answer questions like “Is this the right dataset to use?” or “Where did this data come from?”.
For data observability, data quality means being able to answer questions like “Is the data complete?” or “Is this data up to date?”.
Both are important to ensure that the data is correct and trustworthy to be used in your analysis.
Data Lineage

Data lineage is a type of metadata that demonstrates dataset dependencies at the table, column, and/or job level. You can trace where the data is coming from, and everywhere else it’s being used throughout the system.
From the data observability lens, usage of data lineage is in understanding engineering issues and impacts to data pipelines. If there’s a data quality issue, data observability should help users to answer questions like “what is the root cause?”, “which dashboards will get impacted?”.
For data discovery, data lineage is commonly leveraged for data understanding and change management. It helps data analysts to answer questions like “How was this dashboard generated?” or “Where else is this data being used?”. For change management, product engineers and data engineers can plan for better migration and data remodeling by understanding the impact of moving tables and columns.
Collaboration
As data teams grow bigger, both data discovery and data observability solutions promote collaboration within the team to manage data better. For data observability, this collaboration is often between the data engineering and platform engineering teams. For data discovery, the main collaboration happens between the data engineering team and the BI, data analytics and data science teams.
Many data discovery solutions including Select Star, promote less technical, and/or business stakeholders to leverage the platform and collaborate with others as well. Because of this difference in user personas, you’ll find the functionalities and user interface of data observability vs. data discovery tools to be somewhat different.
Data Discovery and Data Observability: Which Comes First?
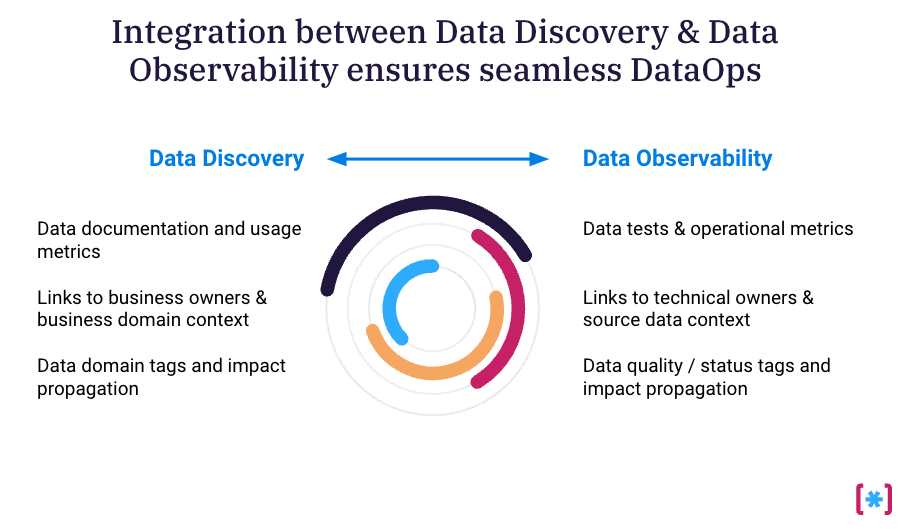
Both data discovery and data observability sound great, but which one should you implement first?
We recommend understanding where your data team spends more of their time today: Is your team spending too much time supporting broken dashboards because the data wasn’t up to date? Or is your team spending too much time answering ad hoc questions explaining the data model and building queries for others? This may clarify if you need a data observability first (former case) or data discovery first (latter case).
Another consideration is if you already know which datasets should be tracked and monitored for quality. It becomes expensive and noisy very quickly if you try to monitor every single dataset in your data warehouse. We find that many companies only use 10-15% of their data today. Through data discovery, you can find out the usage levels of different datasets, their dependencies, and prioritize which ones you should monitor with a data observability solution.
Last but not least, you should think about where your organization is on its data democratization journey. If your company has a primary data warehouse/data lake that is open to many teams, then you are already on your way to data democratization. If your company is investing in data and you’re hiring data analysts and data engineers actively, then you’d need to transfer the knowledge and have documentation to get the new team members onboarded. In these cases, you should look at data discovery first.
If your company data is open to fewer people and you need to ensure your report is 100% correct, you’d want to invest in data observability first to ensure that data quality is being monitored and tracked.
Data Discovery and Data Observability: Better Together
DRE Con was a great opportunity to surface the ideas we have been collecting on this topic while helping our customers navigate their journey with data discovery and data observability. When combined, they provide a powerful foundation for DataOps and data utilization in the business, and we’re excited to see the rapid development in the market. If you would like to discuss your specific case on data discovery and data observability, please reach out to us!