Data strategy serves as the cornerstone for organizations seeking to harness the power of their information assets. By creating a roadmap for leveraging data and analytics to achieve business objectives, companies can unlock new opportunities and drive informed decision-making. This post covers the key components of an effective data strategy, drawing insights from Dylan Anderson, an experienced data strategist and head of data strategy at Profusion.
This post is part of INNER JOIN, a live show hosted by Select Star. INNER JOIN brings together thought leaders and experts to discuss the latest trends in data governance and analytics. For more details, visit Select Star's Inner Join page.
Table of Contents
- What is a Data Strategy?
- How to Tailor a Data Strategy to Your Organization?
- How to Use Business Value to Define and Measure Success for a Data Strategy?
- 4 Steps to Implementing a Data Strategy Successfully
- Case Studies: Data Strategy in Action
- Emerging Trends in Data Strategy
What is a Data Strategy?
Data strategy is the framework that enables organizations to harness data and analytics to achieve their business objectives. It begins with a clear vision, aligning closely with overall business strategy to ensure data initiatives drive tangible value. A comprehensive data strategy encompasses several key components:
- Data Vision and Strategic Pillars
A data strategy begins with a concise vision statement that outlines the organization's aspirations for data utilization. This vision is typically supported by strategic pillars that guide the implementation of the strategy. For example, a company might envision becoming a data-driven organization, with pillars focusing on data governance, analytics capabilities, and cultural transformation.
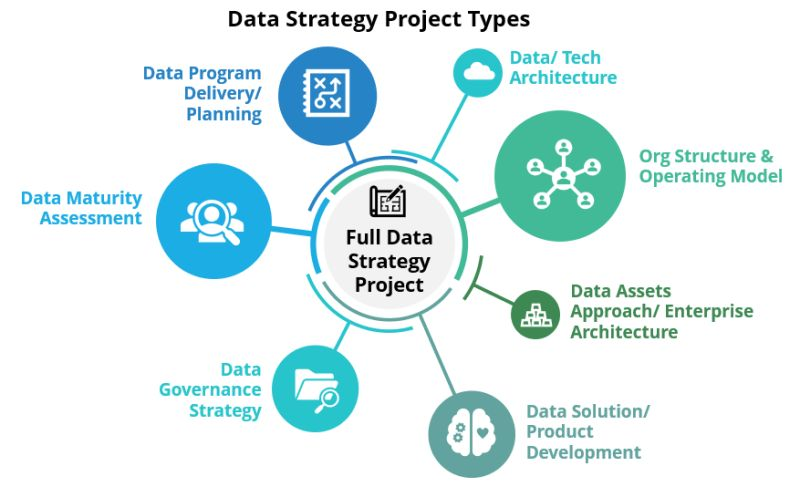
- Use Case Identification and Prioritization
Identifying and prioritizing use cases is crucial for focusing efforts on initiatives that deliver the most value. This involves collaborating closely with stakeholders to understand business needs and opportunities where data can make a significant impact. Use cases can range from building dashboards for real-time decision-making to implementing machine learning models for predictive analytics.
- Competency and Maturity Assessment
Understanding the organization's current data capabilities is essential for crafting a realistic and effective strategy. By identifying strengths and gaps, organizations can prioritize areas for improvement and investment. This assessment covers various aspects of data management, including:- Data governance and quality
- Data architecture and engineering
- Analytics and business intelligence capabilities
- Organizational structure and skills
- Operational Roadmap and Execution Plan
A successful data strategy must include a clear plan for implementation. This plan should outline key milestones, resource requirements, and timelines to execute the strategy and achieve the objectives. It's crucial to have a detailed execution plan to avoid the common challenge of strategies that fail due to lack of implementation. It should also include mechanisms for measuring progress and adjusting the strategy as needed.
How to Tailor a Data Strategy to Your Organization?
Organizations must adapt their data strategy to fit their unique context and needs. At a high level, the approach involves assessing current data capabilities, pinpointing crucial areas for enhancement, and establishing achievable goals with realistic timelines. This ensures that the data strategy aligns with the organization's unique needs and resources, setting the stage for successful implementation and measurable outcomes. The specific approach will vary depending on the company's size, industry, and current data maturity level.
- Startups and Small Businesses: For smaller organizations, the focus should be on establishing a solid data foundation while remaining agile. This might involve implementing basic data governance practices, setting up initial analytics capabilities, choosing scalable data infrastructure solutions, prioritizing key metrics and analytics that directly impact business growth, and identifying quick wins to demonstrate value.
- Mid-sized Companies: Mid-sized companies often face challenges in scaling their data capabilities. Their strategy might emphasize investing in data quality and integration initiatives, developing more advanced analytics capabilities, standardizing data processes, and fostering a data-driven culture across the organization.
- Enterprises: Large enterprises typically require more comprehensive data strategies that address complex organizational structures and diverse data needs to navigate complex data ecosystems and legacy systems. Their strategy may focus on enterprise-wide governance frameworks, advanced data architecture to support various business units, and change management and cultural transformation initiatives. It's crucial for these organizations to break down data silos and promote cross-functional collaboration.

How to Use Business Value to Define and Measure Success for a Data Strategy?
Aligning data initiatives with business KPIs is crucial for demonstrating the value of data strategy. This involves identifying key performance indicators that data initiatives can impact, establishing clear metrics for measuring the success of data projects, and regularly reporting on the ROI of data investments. Organizations must balance short-term gains with long-term value creation. While some data initiatives may yield immediate results, others, such as building robust data infrastructure, may take longer to show returns but are essential for future success. This balanced approach ensures that the data strategy remains relevant and impactful, both in the near term and for sustained organizational growth.
4 Steps to Implementing a Data Strategy Successfully
Transforming a data strategy from concept to reality requires careful planning, strong leadership, and organizational alignment. The journey from strategic vision to tangible results involves overcoming various challenges, from technical hurdles to cultural resistance. By focusing on key implementation principles, organizations can navigate these obstacles and unlock the full potential of their data assets. Let's explore the critical steps that contribute to the successful implementation of a data strategy.
- Secure Executive Buy-in and Sponsorship
Executive support is critical for the success of any data strategy. This involves educating leadership on the importance of data-driven decision-making and demonstrating how the data strategy aligns with overall business objectives.
- Build Cross-functional Teams and Collaboration
Effective data strategies require collaboration across various departments. Building cross-functional teams helps ensure that data initiatives address diverse business needs and that insights are effectively translated into action.
- Iterative Assessment and Strategy Refinement
Data strategies should be viewed as living documents that evolve with the organization's needs and capabilities. Regular assessments and refinements help ensure that the strategy remains relevant and effective in the face of changing business conditions and technological advancements.
- Overcome Common Implementation Challenges
Implementing a data strategy often faces obstacles such as resistance to change, data silos, and skill gaps. Addressing these challenges requires clear communication, targeted training programs, and phased implementation approaches. Organizations must also tackle technical hurdles like legacy systems integration and data quality issues to ensure smooth strategy execution.
Case Studies: Data Strategy in Action
Examining real-world examples provides valuable insights into the practical application and impact of data strategies. Here, we highlight how two organizations from different industries have tackled their unique data challenges, implemented effective strategies, and reaped tangible benefits.
Media Organization's Journey to Improve Data Quality
A major media company had poured significant resources into a data platform without realizing the anticipated benefits. A thorough data strategy evaluation revealed deficiencies in governance and quality. The resulting strategy prioritized cross-divisional collaboration to dismantle data silos, established strong data governance processes, and concentrated on use cases with direct business impact, such as audience targeting and content optimization. This strategic shift resulted in enhanced data quality and a more comprehensive grasp of how data could propel business objectives forward.
Logistics Company's Data Ecosystem Optimization
A logistics company with strong data teams encountered challenges in interdepartmental cohesion. Their data strategy addressed this by recognizing interdependencies between data domains, documenting processes, establishing clear use cases, and enhancing collaboration among data governance, engineering, and analytics teams. These strategic changes led to significant improvements in the company's overall data management efficiency and effectiveness, demonstrating the power of a well-executed data strategy in overcoming organizational silos and optimizing data utilization across the enterprise.
Emerging Trends in Data Strategy
As organizations continue to recognize the critical role of data in driving business success, data strategies will evolve to meet new challenges and opportunities. Future trends may include:
- Greater emphasis on real-time data processing and decision-making
- Integration of external data sources for more comprehensive insights
- Continued focus on data democratization and self-service analytics
- Increasing integration of AI and machine learning
- Adaptive data governance with more flexibility and a focus on prioritization
Effective data strategy remains crucial for organizations seeking to thrive in a data-driven world. By aligning data initiatives with business goals, fostering collaboration across teams, and adapting to emerging trends, organizations can unlock the full potential of their data assets and drive meaningful business outcomes. At Select Star, our modern data governance platform helps you govern and manage your data. Connect with us to learn more about how we can support your data strategy.