Data quality forms the cornerstone of successful AI initiatives, shaping how organizations leverage their data assets for intelligent decision-making. Effective data quality management is essential for handling growing data volumes and complexity in modern AI applications. The evolution of data quality approaches reflects the changing landscape of AI and machine learning, with new techniques emerging to address the challenges of modern analytics. This post covers the role of data quality in AI, 5 steps to improve data quality, and a real-world case study, drawing on experiences from data leader and investor June Dershewitz.
This post is part of INNER JOIN, a live show hosted by Select Star. INNER JOIN brings together thought leaders and experts to discuss the latest advancements in data governance and analytics. For more details, visit Select Star's LinkedIn page.
Table of Contents
- Role of Data Quality in the AI Era
- Step 1: Assess Your Current Data Quality
- Step 2: Build a Strong Foundation for Data Quality
- Step 3: Implement Continuous Monitoring and Automated Data Quality Checks
- Step 4: Leverage AI for Data Quality Enhancement
- Step 5: Tackle Common Data Quality Challenges for AI
- Case Study: Enhancing Data Quality for AI-Driven Decision Making
- Future Trends in Data Quality for AI
Role of Data Quality in the AI Era
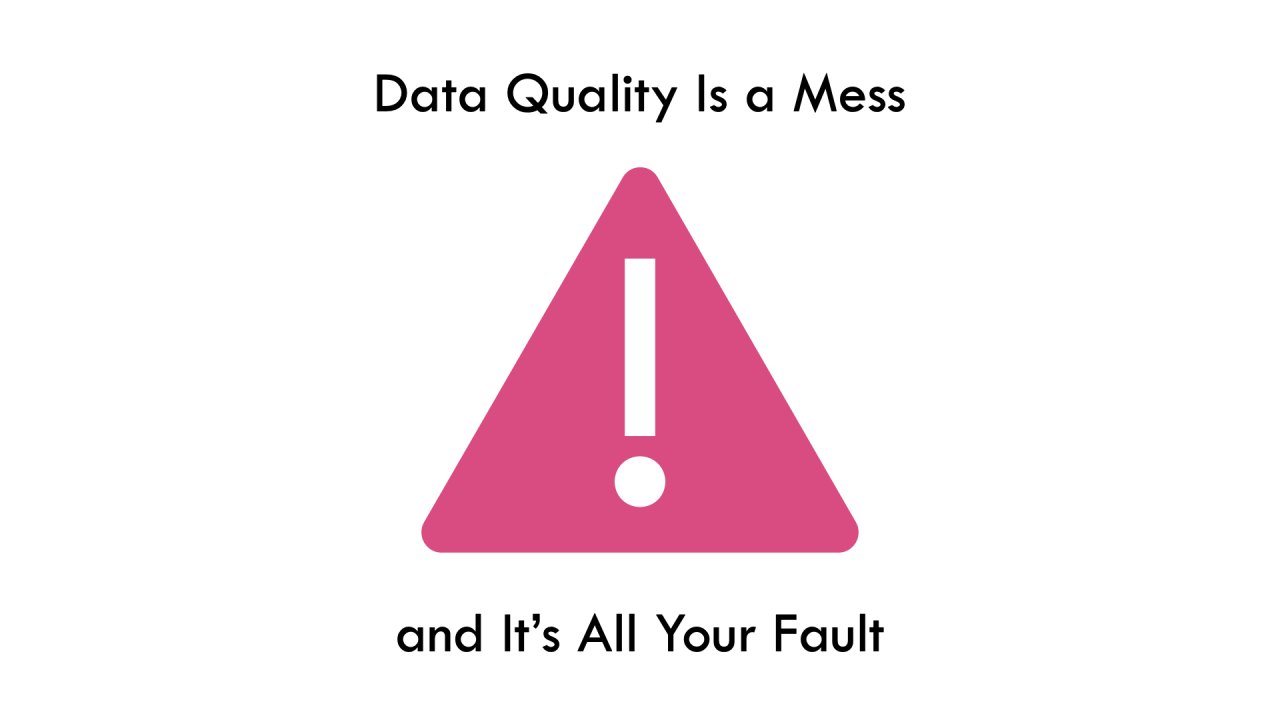
Data quality plays a crucial role in the success of AI applications. High-quality data ensures that AI models perform accurately and reliably, leading to better insights and decision-making. In the AI era, data quality encompasses not only traditional aspects like accuracy and completeness but also factors specific to AI, such as data bias and representativeness.
The significance of data quality for AI performance cannot be overstated. AI models are only as effective as the data they are trained on. Suboptimal data quality can result in biased or inaccurate results, potentially causing significant business impacts or ethical concerns. As organizations increasingly rely on AI for critical decisions, ensuring data quality becomes paramount to mitigate risks and maximize the value of AI investments.
Step 1: Assess Your Current Data Quality
The first step in enhancing data quality for AI is to assess the current state of your data. This involves identifying key data quality metrics relevant to AI applications and conducting a comprehensive data quality audit.
Key data quality metrics for AI may include:
- Accuracy: How correct and precise is the data?
- Completeness: Are there missing values or gaps in the data?
- Consistency: Is the data uniform across different sources and systems?
- Timeliness: Is the data up-to-date and relevant for AI applications?
- Bias: Does the data contain inherent biases that could affect AI model outputs?
Conducting a data quality audit involves analyzing datasets used for AI, documenting issues, and prioritizing areas for improvement. This process helps organizations understand their data landscape and identify critical quality issues that may impact AI performance.
Step 2: Build a Strong Foundation for Data Quality
Establishing a robust foundation for data quality is essential for successful AI initiatives. This step involves creating clear data requirements, developing a comprehensive data quality framework, and fostering a data quality culture within the organization.
Clear data requirements help define what constitutes high-quality data for AI applications. These requirements should be specific, measurable, and aligned with business objectives. For example, an e-commerce company might require product data to be at least 95% accurate and updated within 24 hours of any changes.
Developing a data quality framework provides a structured approach to managing and enhancing data quality. This framework should include:
- Data quality policies and standards
- Roles and responsibilities for data quality management
- Processes for data quality assessment and improvement
- Tools and technologies for data quality monitoring and enhancement
Creating a severity assessment for data issues helps prioritize quality improvement efforts. This assessment should consider factors such as the potential impact on AI model performance, business operations, and customer experience. For instance, a critical data quality issue might be one that could lead to significant financial losses or regulatory non-compliance if not addressed promptly.
Fostering a data quality culture involves educating employees about the importance of data quality for AI and empowering them to take ownership of data quality within their roles. This cultural shift can lead to more proactive identification and resolution of data quality issues across the organization.
Step 3: Implement Continuous Monitoring and Automated Data Quality Checks
Continuous monitoring and automated data quality checks are crucial for maintaining high-quality data for AI applications. These processes help identify and address data quality issues in real-time, preventing poor quality data from impacting AI model performance.
Setting up alerts for data pipelines is an essential component of continuous monitoring. These alerts can notify data teams of issues such as pipeline failures, data anomalies, or unexpected changes in data patterns. For example, an alert might be triggered if the volume of data flowing through a pipeline suddenly drops below a certain threshold, indicating a potential data quality issue.
Utilizing BI tool alerting features can help monitor data quality at the reporting and analytics level. These alerts can flag inconsistencies or anomalies in key metrics, ensuring that data used for decision-making remains reliable. For instance, a BI tool might alert users if sales figures for a particular region deviate significantly from historical trends.
Exploring data observability platforms can provide more comprehensive and automated data quality monitoring. These platforms offer features such as data lineage tracking, anomaly detection, and metadata management, enabling organizations to gain deeper insights into their data quality and quickly identify and resolve issues.
Step 4: Leverage AI for Data Quality Enhancement
AI itself can be a powerful tool for enhancing data quality. By leveraging AI techniques, organizations can automate and improve various aspects of data quality management.
Using AI for root cause analysis can help identify underlying issues causing data quality problems. Machine learning algorithms can analyze patterns in data quality issues and suggest potential root causes, enabling faster and more accurate problem resolution.
Applying AI to data quality triage processes can help prioritize and route data quality issues to the appropriate teams for resolution. This can significantly reduce the time and effort required to address data quality problems, especially in large and complex data environments.
Enhancing anomaly detection with AI can improve the accuracy and efficiency of identifying data quality issues. AI-powered anomaly detection can learn from historical data patterns and adapt to changing data characteristics, providing more nuanced and context-aware alerts compared to traditional rule-based approaches.
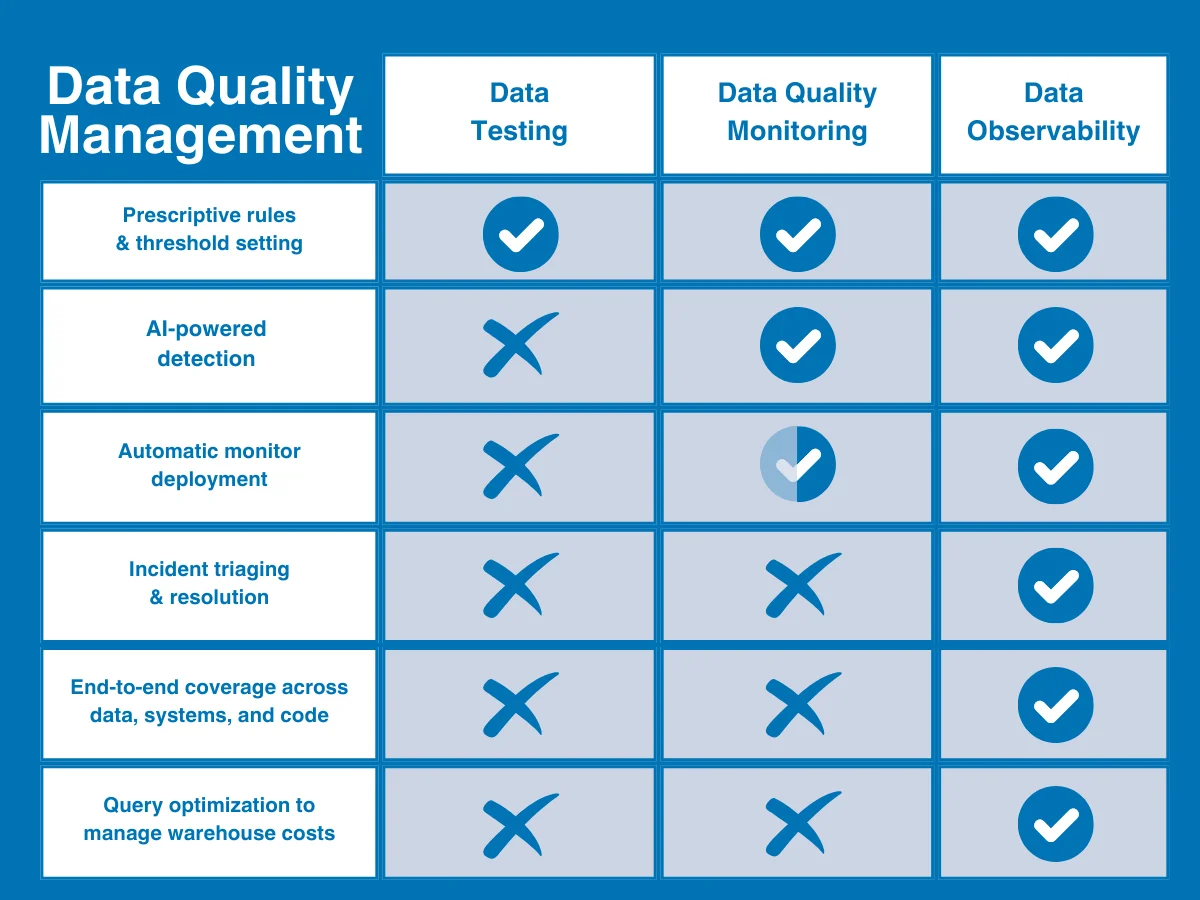
Step 5: Tackle Common Data Quality Challenges for AI
AI applications often face specific data quality challenges that need to be addressed to ensure optimal performance. Some common challenges include:
Managing incomplete or missing data: AI models often require complete datasets to perform accurately. Techniques such as data imputation or model architectures that can handle missing data may be necessary.
Handling biased or skewed datasets: Bias in training data can lead to biased AI outputs. Identifying and mitigating bias through techniques like data augmentation or balanced sampling is crucial for fair and ethical AI applications.
Ensuring data consistency across sources: AI applications often rely on data from multiple sources. Maintaining consistency in data definitions, formats, and quality across these sources is essential for accurate AI results.
Balancing speed and accuracy in data processing: AI applications often require real-time or near-real-time data processing. Striking the right balance between data quality checks and processing speed is crucial for timely and accurate AI insights.
Case Study: Enhancing Data Quality for AI-Driven Decision Making
A real-world example of enhancing data quality for AI can be seen in the healthcare industry. A large healthcare provider sought to implement an AI-driven system for predicting patient readmission risks. The organization identified critical data points for their AI models, including patient demographics, medical history, and treatment outcomes.
To enhance data quality, the healthcare provider implemented several measures:
- Standardized data entry processes across all departments
- Implemented automated data validation checks at the point of entry
- Developed a data quality scoring system for patient records
- Established a dedicated team for ongoing data quality monitoring and improvement
These efforts resulted in significant improvements in data quality, with the completeness and accuracy of patient records increasing by over 30%. This enhanced data quality led to more accurate AI predictions of readmission risks, enabling the healthcare provider to implement targeted interventions and reduce readmission rates by 15%.
Future Trends in Data Quality for AI
As AI continues to evolve, several trends are emerging in the field of data quality. Advanced data quality tools are incorporating AI and machine learning capabilities, while there's an increased focus on ethical considerations in AI data quality, such as fairness and transparency. We're also seeing greater integration of data quality processes with AI model development workflows, and the rise of federated learning and privacy-preserving AI techniques, which are impacting data quality requirements. Organizations that prioritize data quality and stay ahead of these trends will be better equipped to harness the full potential of AI technologies. By implementing robust data quality practices and leveraging emerging tools and techniques, businesses can build a strong foundation for successful AI initiatives.
Considering AI initiatives at your organization? Connect with our team at Select Star to discuss how we can support implementing effective data quality tailored to your needs and establish a strong foundation for your AI initiatives.