Data quality forms the foundation of effective decision-making in modern enterprises. As organizations adapt to managing data across multiple cloud platforms, ensuring data quality becomes increasingly crucial. Piotr Czarnas, founder of DQOps, shares his learnings and insights on measuring, implementing, and improving data quality in today's complex data environments.
This post is part of INNER JOIN, a live show hosted by Select Star. INNER JOIN is all about bringing together thought leaders and experts to chat about the latest and greatest in the world of data governance and analytics. To join us on our next episode, follow Select Star’s LinkedIn page.
Table of Contents
- Overlooked Cause of Data Quality Challenges
- Using Data Lineage to Improve Data Quality
- 5 Steps to Build an Effective Data Quality Strategy
- How to Measure Data Quality
- Future of Data Quality
Overlooked Cause of Data Quality Challenges
For data-driven organizations, poor data quality can become a major headache and significantly impact the business. Marketing teams may waste resources on ineffective campaigns due to unreliable customer data, resulting in poor returns on investment. Similarly, finance departments could produce misleading forecasts based on incomplete sales figures, potentially guiding the organization toward flawed strategic decisions. Czarnas explains that upon closer examination, what appears to be a data quality issue often stems from difficulties in locating and utilizing the correct data. Data analysts might spend hours sifting through numerous similar tables to find the right dataset, while business users may unknowingly rely on outdated reports, resulting in misguided decisions. By enhancing data discovery within your organization, you not only improve data findability and reliability but also bolster overall data quality and foster more informed, data-driven decision-making processes.
Using Data Lineage to Improve Data Quality
In addition to data catalogs that facilitate data asset discovery and understanding across organizations by centralizing metadata management and documentation, data lineage serves as a crucial component of data quality management. Data lineage offers a comprehensive view of data flows and transformations throughout an organization's ecosystem. Cross-platform data lineage enables organizations to trace data and potential quality issues across systems. This capability is particularly valuable in today's complex data environments, where information often traverses multiple platforms before reaching its final destination. With cross-platform lineage, data teams can pinpoint the exact source of quality problems, whether they originate in legacy systems, cloud platforms, or third-party data sources. This level of visibility not only facilitates faster issue resolution but also supports proactive data governance strategies, helping organizations maintain high data quality standards across their entire data landscape.
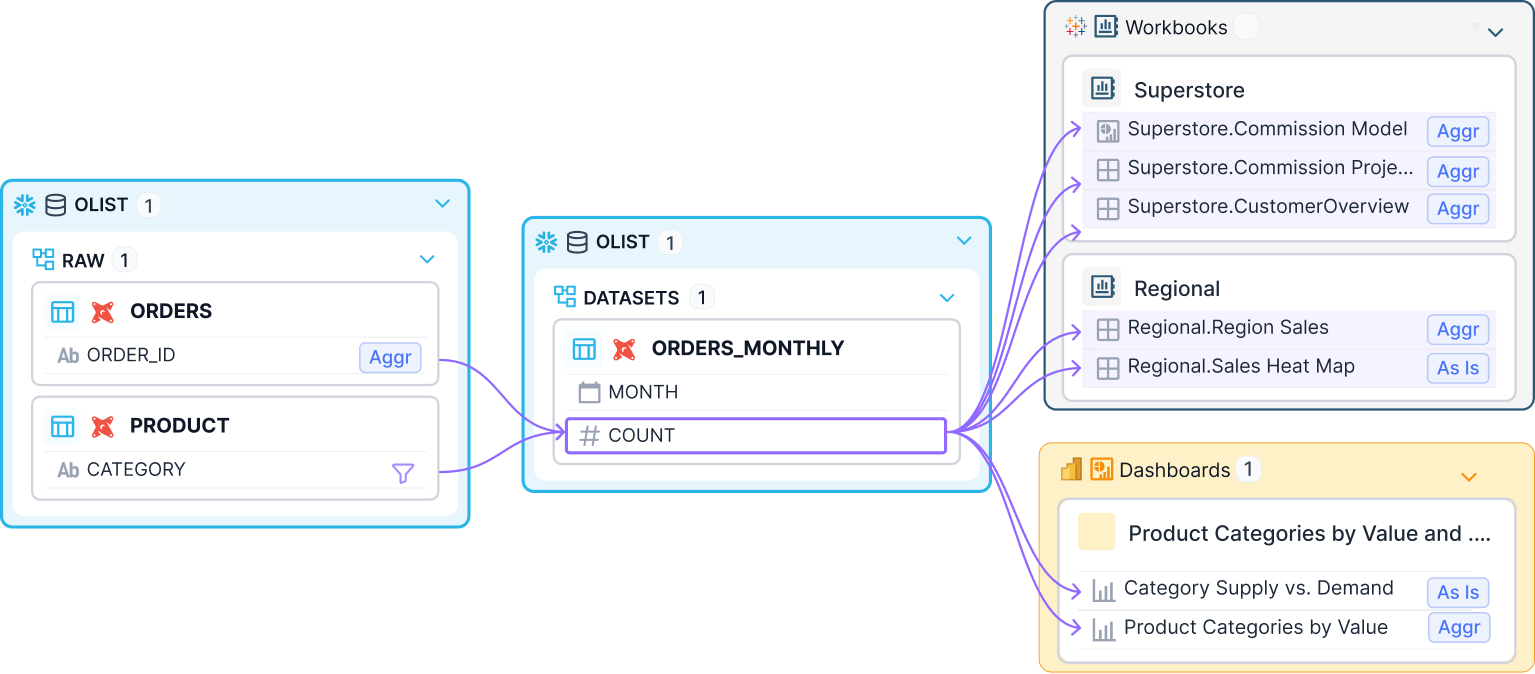
5 Steps to Build an Effective Data Quality Strategy
Understanding the root causes of data quality issues sets the stage for developing a robust data quality strategy. Czarnas outlines five key steps organizations can follow to improve their data quality:
- Engage data stakeholders: Involve data asset owners and data engineers to gather requirements and identify areas for pipeline improvements.
- Create a data quality data warehouse: Establish a central repository to track and analyze data quality issues across the organization.
- Identify and prioritize critical data elements: Focus on the most important tables and data points that drive business decisions. Leverage a data catalog with lineage tracking and usage analytics to understand usage patterns and dependencies.
- Triage data quality issues: Develop a system for initial review and prioritization of data quality challenges.
- Balance automation and manual correction: Implement automated data quality checks while maintaining the ability to manually intervene when necessary.
How to Measure Data Quality
Measuring data quality is critical to successfully implementing a data quality strategy. Measuring data quality involves a combination of objective and subjective approaches. Czarnas recommends calculating data quality KPIs based on failed checks, which provides a quantitative measure of data integrity. However, he emphasizes the importance of balancing these objective metrics with subjective assessments of perceived data quality among users. This holistic approach ensures that data quality measures align with both technical standards and user expectations.
To effectively measure data quality:
- Implement automated checks to identify failed data quality rules
- Track the number of failed checks over time

- Gather feedback from data users on their perception of data quality
- Combine objective metrics and subjective assessments for a comprehensive view

Future of Data Quality
The field of data quality continues to evolve, with several emerging trends shaping its future. Czarnas highlights the growing integration between data catalogs and data quality tools, enabling more comprehensive metadata management and lineage tracking. This integration supports better governance and facilitates more effective collaboration across data teams.
Advanced data lineage capabilities are becoming increasingly important, allowing organizations to trace data origins and transformations more accurately. This enhanced visibility helps in identifying root causes of quality issues and assessing the impact of data changes across systems.
Czarnas also predicts increased engagement of business users in data quality processes. As data becomes more critical to business operations, non-technical stakeholders will play a larger role in defining quality standards and assessing data fitness for use. This shift underscores the importance of perceived data quality alongside technical metrics.
Organizations are recognizing the need for dedicated data quality resources. As data ecosystems grow more complex, having specialists focused on maintaining and improving data quality becomes essential for supporting data-driven decision-making and maintaining trust in organizational data assets.
By implementing these strategies and staying attuned to emerging trends, organizations can significantly improve their data quality, leading to more reliable analytics and better-informed business decisions. As data continues to grow in volume and importance, effective data quality management will remain a critical component of successful data strategies. The Select Star team is ready to support you on your data quality journey, sharing how customers use the platform to achieve their data quality goals. Schedule time with our team to explore how we can support your data quality initiatives and drive your organization toward data-driven success.