In early 2018, Uber acquired Jump, a bike-sharing tech startup where users can rent dockless scooters and electric bikes to explore major cities. At the same time, stricter data security policies were going into full effect and many companies, including Uber, needed to ensure compliance. Both Jump and Uber collected data on their users, the origin and destination of their trips, and various other pieces of data, which all need to be handled with care.
James Greenhill was the data engineering lead for Jump when they were acquired by Uber, and he was tasked with integrating Jump’s data and ensuring GDPR compliance. James and his team were responsible for implementing a data governance strategy that would help them combine Jump and Uber’s data and achieve compliance with a new set of regulations. He gave us insight into how they were able to take a collaborative approach to data governance that actually worked in a large company with a large amount of data.
Ultimately, James said the single most important aspect of their data governance strategy was their data catalog, Databook. In order to successfully integrate data, achieve legal compliance, and create sustainable data governance in the long run, the creation of a data catalog was a necessary first step.
Uber’s twofold need for data governance
Around the time of Uber’s acquisition of Jump, the General Data Protection Regulation (GDPR) and the California Consumer Privacy Act (CCPA) came into full effect. These laws require companies to meet certain standards of security when it comes to the use and storage of consumer data, especially Personally Identifiable Information, also known as PII, even if the company does not operate exclusively in California or the European Union.
James and his team were tasked with migrating Jump’s data into Uber’s data stack. They also needed to make sure Uber was compliant, especially with the GDPR guidelines, when both companies’ data involved not only PII for their users, but also location information.
The answer to both problems would center around identifying all of their sensitive data and then controlling access to the data, so only those who really needed to see data would be able to. In other words, they needed a data governance strategy. They also knew that their data governance would need to work for other businesses inside Uber.
When asked how he would define data governance, James explained, “It’s about making sure you give everyone the access that they need to have without leaking data they don’t need to have. Basically, bias for as little access as possible, while at the same time, not blocking people.”
Uber did not want to block employees who used data to make business decisions from accessing that data, but they also recognized the need to protect their users’ data from misuse and achieve compliance with laws like GDPR and CCPA.
The challenge of achieving data compliance
The biggest challenge Uber faced when trying to achieve compliance was the amount of data they needed to parse through. While many companies aspire to keep the number of data tables to a minimum so the data model is small and simple, new tables are often created with transformed or aggregated data to serve specific use cases more directly.
Uber was no different. James and his team knew they would have to find and identify all of the places PII or sensitive data existed in their raw tables, and also anywhere it was propagated downstream, where it may have changed names or forms. This work would require a lot of manual evaluation.
Fortunately, Uber already had an internal tool called Databook, which they had developed to organize and derive insights from their data. This tool became the key to James’ data governance strategy.
Using a data catalog to create data governance
James says that having a data catalog was the most important thing for achieving data governance. Databook already had a concept of ownership for datasets. This made it easy to distribute the work of documenting and classifying Uber and Jump’s data.
Teams were assigned tables, then asked to go through their datasets and classify them according to a certain timeline. They could mark which data did include PII, did not include PII, or required further examination. They would then be able to identify which datasets were already “GDPR reviewed” and get an overview of what still needed to be done. Access control could then be implemented based on which datasets had PII and who was allowed to access them.
Overall, James said the process was made much easier because they had a data catalog. The fact that Uber had already built Databook for their internal data cataloging was extremely valuable. But James said there were notable ways that Databook could have helped them even more.
Where Uber’s Databook could have helped more
Databook was a huge asset when James joined Uber. They could use Databook to categorize data and make it clear at a high level where PII existed, and how far along they were in their efforts to achieve GDPR compliance. Having an abstracted metadata layer like that was invaluable to James and his team, but that didn’t mean the process had been perfect.
During the categorization process, they would often need to manually examine PII columns that had been propagated to see if the PII was still exposed. They needed to try to determine if someone could infer location from a piece of data, or identify demographics. This would have been easier if the tool had been able to tell them if the data had been aggregated or transformed in some way that would make it impossible to get information on an individual user.
While Databook was great to use for storing information about their tables, it was easy to miscategorize data. James added, “If it was automatic or had suggestions, that would have been really nice. If it could profile the data, and say, ‘Hey we think this is PII, can you confirm?’”
Databook was able to show table lineages, so if one table contained PII, it would be obvious to check downstream tables for PII as well. However, that would have been even easier if Databook had shown column-level lineage. “Having it propagate down, where at the top of this tree of dependencies, we said this column is PII. And if it knew how all of the queries hit that source table, it could propagate down to all of the columns that are based on that column,” James said.
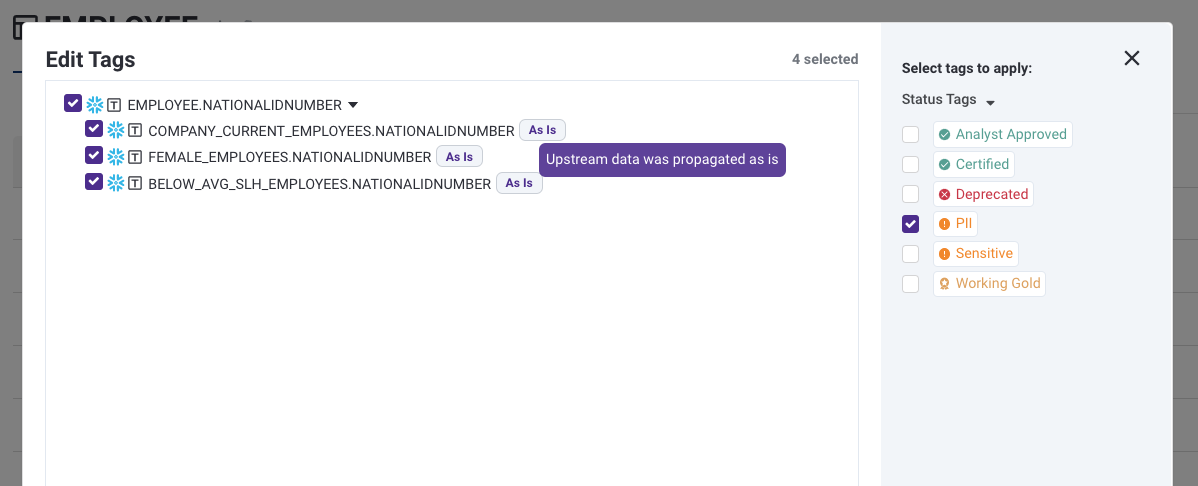
Data cataloging is the start of data governance
Uber had some struggles when trying to categorize their data to achieve GDPR compliance and organization-wide data governance, even with their high-powered, internally built tool. But other, smaller companies have it even harder. “I think most companies say, ‘We’re going to fly under the radar until this becomes a problem.’ It becomes a very acute pain that you have to solve, just because it’s so tough to manage without having an entire team dedicated to it,” James said.
But he still emphasizes the importance of having a data catalog, and how that investment can make the data governance process smoother and sustainable in the long run. James thinks tools like Select Star can help address some of the problems he wished they could have solved at Uber when becoming GDPR compliant.
“I’m excited for modern data catalogs like Select Star that have automated data lineage, because it makes it so that smaller companies can use these tools that larger companies have, that, without them, would make this terribly hard.” — James Greenhill
At Select Star, we believe having a good understanding of the metadata and its usage can give you the insights you need for your data governance. To learn more about how Select Star can help your organization build a simple, scalable data governance model, schedule a demo today.